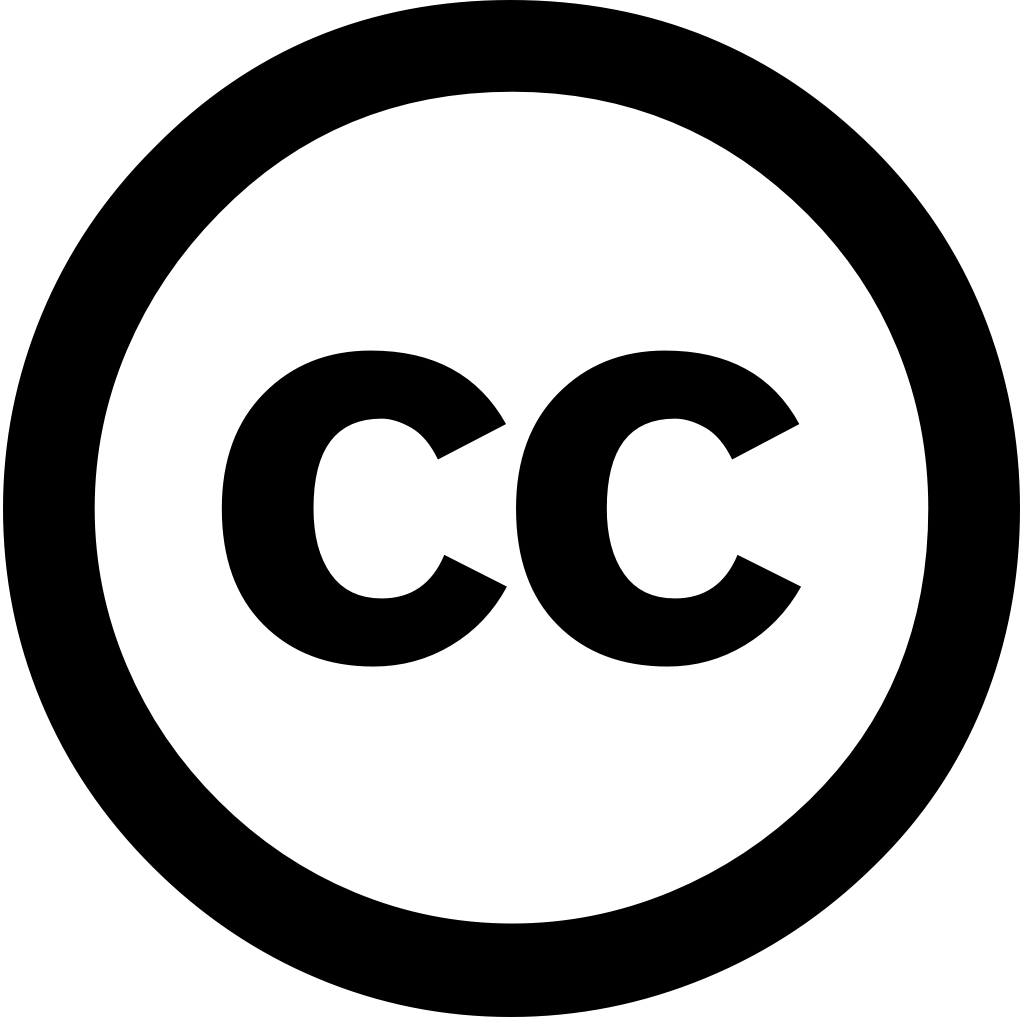
Research Square (Research Square), Journal Year: 2025, Volume and Issue: unknown
Published: March 24, 2025
Language: Английский
Research Square (Research Square), Journal Year: 2025, Volume and Issue: unknown
Published: March 24, 2025
Language: Английский
Forum for education studies., Journal Year: 2025, Volume and Issue: 3(2), P. 2730 - 2730
Published: April 1, 2025
This paper explores the transformative role of artificial intelligence (AI) in medical education, emphasizing its as a pedagogical tool for technology-enhanced learning. highlights AI’s potential to enhance learning process various inquiry-based strategies and support Competency-Based Medical Education (CBME) by generating high-quality assessment items with automated personalized feedback, analyzing data from both human supervisors AI, helping predict future professional behavior current trainees. It also addresses inherent challenges limitations using AI student assessment, calling guidelines ensure valid ethical use. Furthermore, integration into virtual patient (VP) technology offer experiences encounters significantly enhances interactivity realism overcoming conventional VPs. Although incorporating chatbots VPs is promising, further research warranted their generalizability across clinical scenarios. The discusses preferences Generation Z learners suggests conceptual framework on how integrate teaching supporting learning, aligning needs today’s students utilizing adaptive capabilities AI. Overall, this areas education where can play pivotal roles overcome educational offers perspectives developments education. calls advance theory practice tools innovate practices tailored understand long-term impacts AI-driven environments.
Language: Английский
Citations
0European Journal of Clinical Pharmacology, Journal Year: 2025, Volume and Issue: unknown
Published: April 9, 2025
Language: Английский
Citations
0Research Square (Research Square), Journal Year: 2025, Volume and Issue: unknown
Published: March 24, 2025
Language: Английский
Citations
0