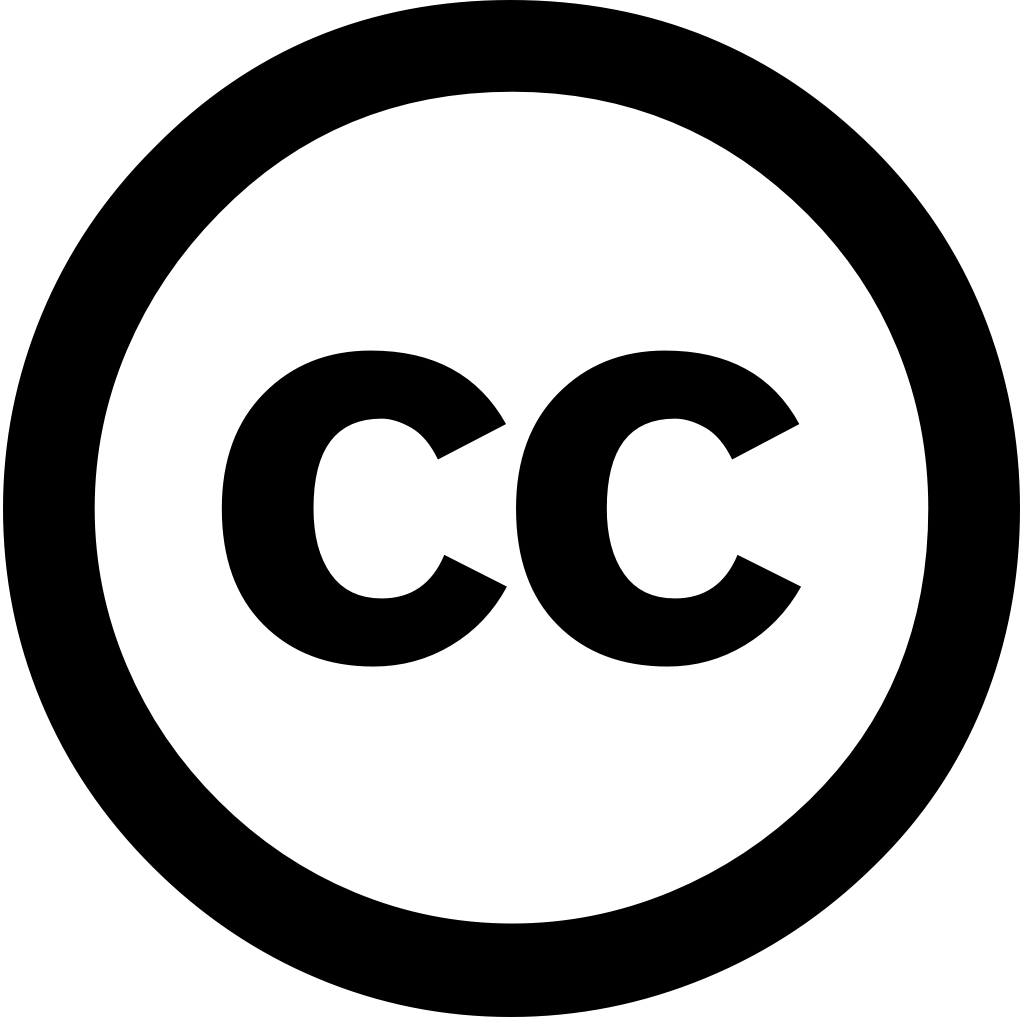
IEEE Access, Journal Year: 2024, Volume and Issue: 12, P. 172358 - 172367
Published: Jan. 1, 2024
Language: Английский
IEEE Access, Journal Year: 2024, Volume and Issue: 12, P. 172358 - 172367
Published: Jan. 1, 2024
Language: Английский
International Journal of Molecular Sciences, Journal Year: 2025, Volume and Issue: 26(3), P. 917 - 917
Published: Jan. 22, 2025
Advances in neuro-oncology have transformed the diagnosis and management of brain tumors, which are among most challenging malignancies due to their high mortality rates complex neurological effects. Despite advancements surgery chemoradiotherapy, prognosis for glioblastoma multiforme (GBM) metastases remains poor, underscoring need innovative diagnostic strategies. This review highlights recent imaging techniques, liquid biopsies, artificial intelligence (AI) applications addressing current challenges. Advanced including diffusion tensor (DTI) magnetic resonance spectroscopy (MRS), improve differentiation tumor progression from treatment-related changes. Additionally, novel positron emission tomography (PET) radiotracers, such as 18F-fluoropivalate, 18F-fluoroethyltyrosine, 18F-fluluciclovine, facilitate metabolic profiling high-grade gliomas. Liquid biopsy, a minimally invasive technique, enables real-time monitoring biomarkers circulating DNA (ctDNA), extracellular vesicles (EVs), cells (CTCs), tumor-educated platelets (TEPs), enhancing precision. AI-driven algorithms, convolutional neural networks, integrate tools accuracy, reduce interobserver variability, accelerate clinical decision-making. These innovations advance personalized neuro-oncological care, offering new opportunities outcomes patients with central nervous system tumors. We advocate future research integrating these into workflows, accessibility challenges, standardizing methodologies ensure broad applicability neuro-oncology.
Language: Английский
Citations
2Current Opinion in Otolaryngology & Head & Neck Surgery, Journal Year: 2025, Volume and Issue: unknown
Published: March 4, 2025
This review aims to explore the integration of artificial intelligence (AI) in laryngology, with specific focus on barriers preventing translation from pilot studies into routine clinical practice and strategies for successful implementation. Laryngology has seen an increasing number proof-of-concept demonstrating AI's ability enhance diagnostics, treatment planning, patient outcomes. Despite these advancements, few tools have been successfully adopted settings. Effective implementation requires application established science frameworks early design phase. Additional factors required AI applications include addressing needs, fostering diverse interdisciplinary teams, ensuring scalability without compromising model performance. Governance, epistemic, ethical considerations must also be continuously incorporated throughout project lifecycle ensure safe, responsible, equitable use technologies. While hold significant promise advancing its remains limited. Achieving meaningful will require a shift toward practical solutions that prioritize clinicians' patients' usability, sustainability, alignment workflows.
Language: Английский
Citations
0Computers in Biology and Medicine, Journal Year: 2025, Volume and Issue: 189, P. 109925 - 109925
Published: March 12, 2025
Language: Английский
Citations
0Sensors, Journal Year: 2025, Volume and Issue: 25(6), P. 1803 - 1803
Published: March 14, 2025
Imaging technologies based on vector optical fields hold significant potential in the biomedical field, particularly for non-invasive scattering imaging of anisotropic biological tissues. However, dynamic and nature tissues poses severe challenges to propagation reconstruction due light scattering. To address this, we propose a deep learning-based polarization-resolved restoration method aimed at achieving efficient accurate from speckle patterns generated after passing through time-varying media. By innovatively leveraging two orthogonal polarization components fields, our approach significantly enhances robustness media, benefiting additional information dimension vectorial powerful learning capacity neural network. For first time, hybrid network model is designed that integrates convolutional networks (CNN) with Transformer architecture capturing local global features image, enabling adaptive dynamically patterns. The experimental results demonstrate exhibits excellent generalization capabilities reconstructing behind This study not only provides an solution but also advances application environments integration technologies.
Language: Английский
Citations
0Journal of Biomedical Informatics, Journal Year: 2025, Volume and Issue: unknown, P. 104823 - 104823
Published: April 1, 2025
Language: Английский
Citations
0Computational Biology and Chemistry, Journal Year: 2025, Volume and Issue: 118, P. 108473 - 108473
Published: April 12, 2025
Language: Английский
Citations
0Journal of Biomedical Informatics, Journal Year: 2025, Volume and Issue: 166, P. 104819 - 104819
Published: April 16, 2025
Language: Английский
Citations
0Electronic Research Archive, Journal Year: 2025, Volume and Issue: 33(4), P. 2366 - 2390
Published: Jan. 1, 2025
Language: Английский
Citations
0Frontiers in Neuroinformatics, Journal Year: 2025, Volume and Issue: 19
Published: May 2, 2025
Introduction Alzheimer’s disease is a progressive neurodegenerative disorder challenging early diagnosis and treatment. Recent advancements in deep learning algorithms applied to multimodal brain imaging offer promising solutions for improving diagnostic accuracy predicting progression. Method This narrative review synthesizes current literature on applications using neuroimaging. The process involved comprehensive search of relevant databases (PubMed, Embase, Google Scholar ClinicalTrials.gov ), selection pertinent studies, critical analysis findings. We employed best-evidence approach, prioritizing high-quality studies identifying consistent patterns across the literature. Results Deep architectures, including convolutional neural networks, recurrent transformer-based models, have shown remarkable potential analyzing neuroimaging data. These models can effectively structural functional modalities, extracting features associated with pathology. Integration multiple modalities has demonstrated improved compared single-modality approaches. also promise predictive modeling, biomarkers forecasting Discussion While approaches show great potential, several challenges remain. Data heterogeneity, small sample sizes, limited generalizability diverse populations are significant hurdles. clinical translation these requires careful consideration interpretability, transparency, ethical implications. future AI neurodiagnostics looks promising, personalized treatment strategies.
Language: Английский
Citations
0Discover Oncology, Journal Year: 2025, Volume and Issue: 16(1)
Published: May 8, 2025
Cancer remains one of the deadliest diseases globally, significantly impacting patients' quality life. Addressing rising incidence cancer deaths necessitates innovative approaches such as nanomedicine and artificial intelligence (AI). The convergence AI represents a transformative frontier in healthcare, promising unprecedented advancements diagnosis, treatment, patient management. This narrative review explores distinct applications oncology, alongside their synergistic potential. Nanomedicine leverages nanoparticles for targeted drug delivery, enhancing therapeutic efficacy while minimizing adverse effects. Concurrently, algorithms facilitate early detection, personalized treatment planning, predictive analytics, thereby optimizing clinical outcomes. Emerging integrations these technologies could transform care by facilitating precise, personalized, adaptive strategies. synthesizes current research, highlights individual applications, discusses emerging oncology. goal is to provide comprehensive understanding how cutting-edge can collaboratively improve prognosis.
Language: Английский
Citations
0