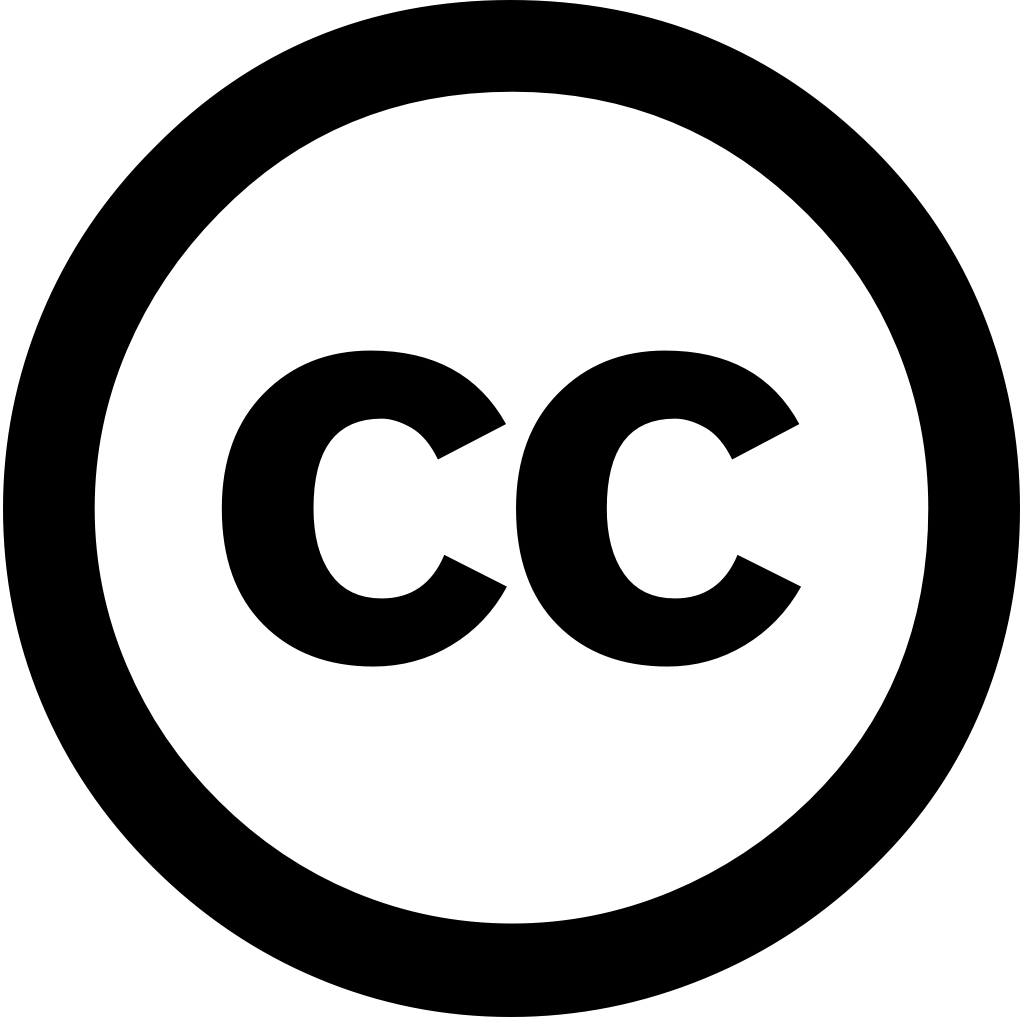
IEEE Access, Journal Year: 2024, Volume and Issue: 12, P. 172358 - 172367
Published: Jan. 1, 2024
Language: Английский
IEEE Access, Journal Year: 2024, Volume and Issue: 12, P. 172358 - 172367
Published: Jan. 1, 2024
Language: Английский
Medical Physics, Journal Year: 2025, Volume and Issue: unknown
Published: May 19, 2025
Abstract Background Microvascular invasion (MVI) is strongly associated with the prognosis of patients hepatocellular carcinoma (HCC). Purpose To evaluate value Transformer models Sonazoid contrast‐enhanced ultrasound (CEUS) in preoperative prediction MVI. Methods This retrospective study included 164 HCC patients. Deep learning features and radiomic were extracted from arterial Kupffer phase images, alongside collection clinicopathological parameters. Normality was assessed using Shapiro–Wilk test. The Mann‒Whitney U ‐test least absolute shrinkage selection operator algorithm applied to screen features. Transformer, radiomic, clinical for MVI constructed logistic regression. Repeated random splits followed a 7:3 ratio, model performance evaluated over 50 iterations. area under receiver operating characteristic curve (AUC, 95% confidence interval [CI]), sensitivity, specificity, accuracy, positive predictive (PPV), negative (NPV), decision curve, calibration used models. DeLong test compare between Bonferroni method control type I error rates arising multiple comparisons. A two‐sided p ‐value < 0.05 considered statistically significant. Results In training set, diagnostic arterial‐phase (AT) Kupffer‐phase (KT) better than that (Clin) ( 0.0001). validation both AT KT outperformed Clin terms 0.05). AUC (95% CI) 0.821 (0.72–0.925) an accuracy 80.0%, 0.859 (0.766–0.977) 70.0%. Logistic regression analysis indicated tumor size = 0.016) alpha‐fetoprotein (AFP) 0.046) independent predictors Conclusions CEUS have potential effectively identifying MVI‐positive preoperatively.
Language: Английский
Citations
0medRxiv (Cold Spring Harbor Laboratory), Journal Year: 2024, Volume and Issue: unknown
Published: Oct. 10, 2024
Abstract Importance Many clinically significant health conditions are frequently underreported, underdiagnosed or recorded only in unstructured textual records, yet they contain critical information for patient assessment, care and prognosis. Objective To determine whether deep learning-based natural language processing employed named entity recognition can effectively identify conditions, such as incontinence, falls, mobility limitations loneliness electronic records. The identified were further used to predict all-cause mortality. Design This cohort study utilized records from public primary, secondary, tertiary, long-term home 2010 2022, providing up 12 years of follow-up. task limitations, was implemented using Google’s Bidirectional Encoder Representations Transformers learning model pre-trained the Finnish language. Diagnostic codes incontinence falls collected comparisons. Setting Retrospective across Central Finland wellbeing services county. Participants Structured summary data 10.6 million free-text entries 102,525 patients aged 50 80 at baseline. Exposure Incontinence, considered exposures. Main Outcomes Measures performance models evaluated by precision, recall F1 scores benchmarked against human ratings. Cox regression assess compare NER- diagnostic code-identified onsets predicting Results demonstrated excellent with recall, precision 0.86, 0.88, 0.87 falls; 0.84, 0.78, 0.81 incontinence; 0.85 0.91, loneliness, respectively. Compared codes, greater numbers (31987 vs 4090) (7059 3873) yielded hazard ratios: 1.31 1.04 1.99 0.65 incontinence. Conclusions Relevance Deep reliably medical presenting new opportunities use clinical vulnerable apply method research applications. KEY POINTS Question Can (NLP) (EHRs)? Findings results this demonstrate that a deep-learning NLP EHR data. approach also improved mortality prediction compared available codes. Meaning approaches could be underreported textural data, enabling identification at-risk patients.
Language: Английский
Citations
0IEEE Access, Journal Year: 2024, Volume and Issue: 12, P. 172358 - 172367
Published: Jan. 1, 2024
Language: Английский
Citations
0