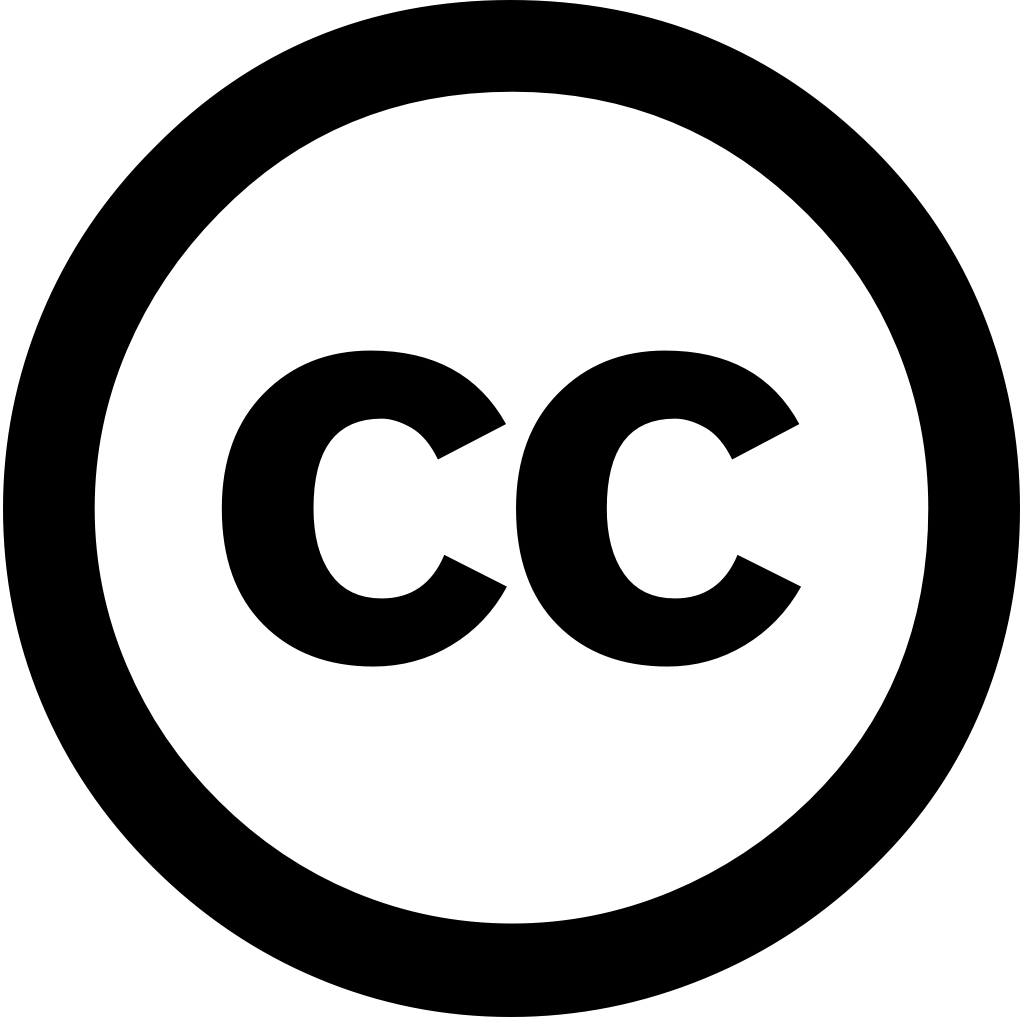
BMC Nursing, Journal Year: 2025, Volume and Issue: 24(1)
Published: April 7, 2025
Neonatal nurses in high-risk Intensive Care Units (NICUs) navigate complex, time-sensitive clinical decisions where accuracy and judgment are critical. Generative artificial intelligence (AI) has emerged as a supportive tool, yet its integration raises concerns about impact on nurses' decision-making, professional autonomy, organizational workflows. This study explored how neonatal experience integrate generative AI examining influence nursing practice, dynamics, cultural adaptation Saudi Arabian NICUs. An interpretive phenomenological approach, guided by Complexity Science, Normalization Process Theory, Tanner's Clinical Judgment Model, was employed. A purposive sample of 33 participated semi-structured interviews focus groups. Thematic analysis used to code interpret data, supported an inter-rater reliability 0.88. Simple frequency counts were included illustrate the prevalence themes but not quantitative measures. Trustworthiness ensured through reflexive journaling, peer debriefing, member checking. Five emerged: (1) Decision-Making, 93.9% reported that AI-enhanced required human validation; (2) Professional Practice Transformation, with 84.8% noting evolving role boundaries workflow changes; (3) Organizational Factors, 97.0% emphasized necessity infrastructure, training, policy integration; (4) Cultural Influences, 87.9% highlighting AI's alignment family-centered care; (5) Implementation Challenges, 90.9% identified technical barriers strategies. can support effectiveness depends structured reliable culturally sensitive implementation. These findings provide evidence-based insights for policymakers healthcare leaders ensure enhances expertise while maintaining safe, patient-centered care.
Language: Английский