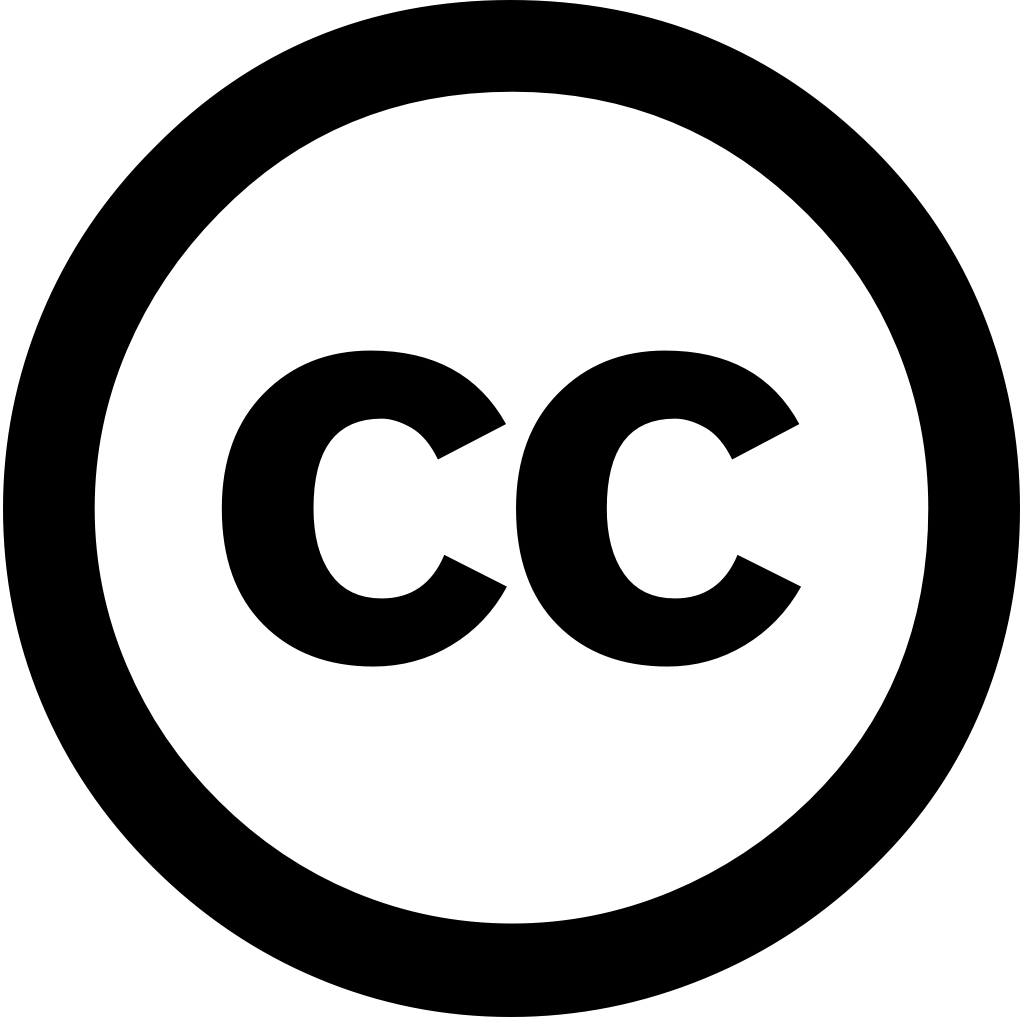
Lupus Science & Medicine, Journal Year: 2024, Volume and Issue: 11(2), P. e001311 - e001311
Published: Nov. 1, 2024
Objective Systemic lupus erythematosus (SLE) is an autoimmune disease characterised by a loss of immune tolerance, affecting multiple organs and significantly impairing patients’ health quality life. While hereditary elements are essential in the onset SLE, external environmental influences also significant. Currently, there few predictive models for SLE that takes into account impact occupational living exposures. Therefore, we collected basic information, background exposure data from patients with to construct model facilitates easier intervention. Methods We conducted study comparing 316 individuals diagnosed 851 healthy volunteers case–control design, collecting their history data. Subjects were randomly allocated training validation groups using 70/30 split. Using three-feature selection methods, constructed four multivariate logistic regression. Model performance clinical utility evaluated via receiver operating characteristic, calibration decision curves. Leave-one-out cross-validation further validated models. The best was used create dynamic nomogram, visually representing predicted relative risk onset. Results ForestMDG demonstrated strong ability, area under curve 0.903 (95% CI 0.880 0.925) set 0.851 0.809 0.894) set, as indicated evaluation. Calibration curves accurate results along practical value. confirmed had accuracy (0.8338). Finally, developed nomogram use, which accessible following link: https://yingzhang99321.shinyapps.io/dynnomapp/ . Conclusion created user-friendly predicting based on Trial registration number ChiCTR2000038187.
Language: Английский