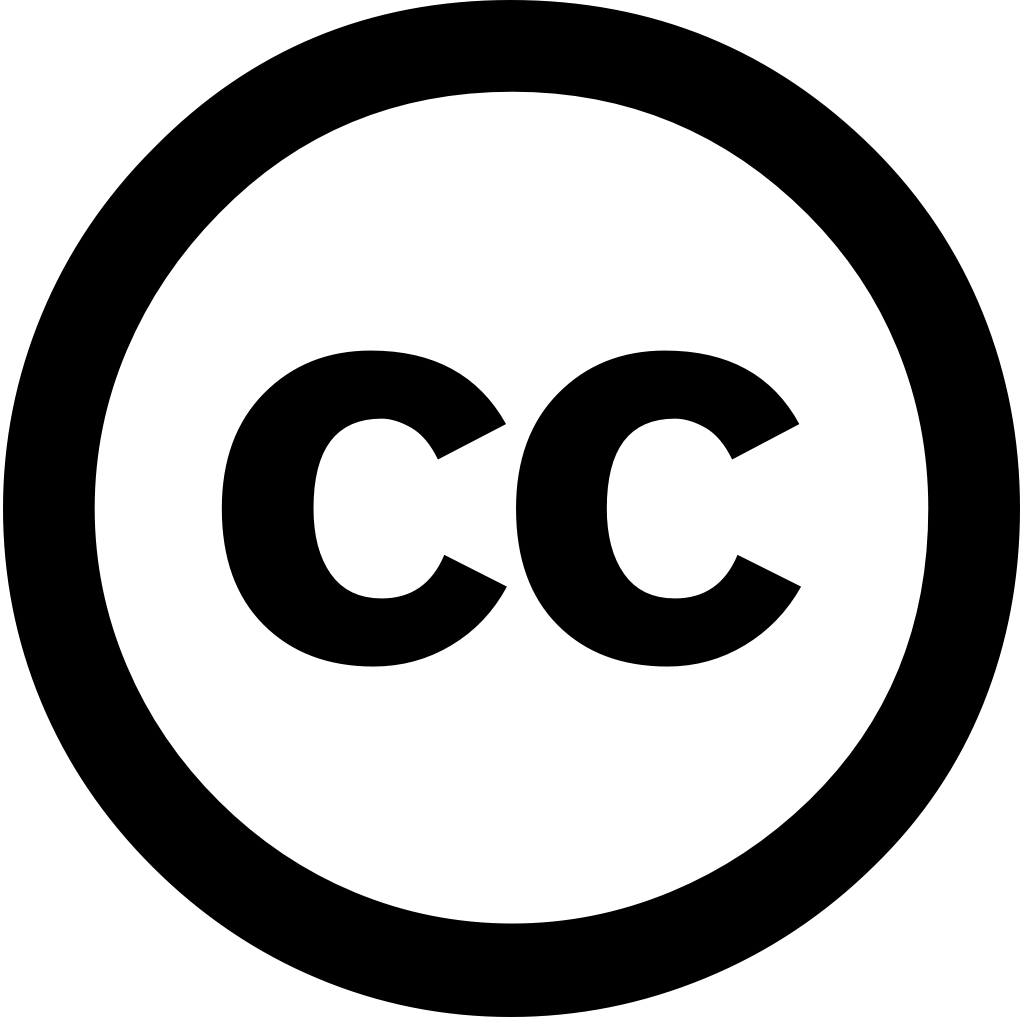
EJNMMI Physics, Journal Year: 2025, Volume and Issue: 12(1)
Published: April 16, 2025
Language: Английский
EJNMMI Physics, Journal Year: 2025, Volume and Issue: 12(1)
Published: April 16, 2025
Language: Английский
Seminars in Nuclear Medicine, Journal Year: 2025, Volume and Issue: unknown
Published: Jan. 1, 2025
This review aims to provide an up-to-date overview of the utility artificial intelligence (AI) in evaluating prostate-specific membrane antigen (PSMA) positron emission tomography (PET) scans for prostate cancer (PCa). A literature was conducted on Medline, Embase, Web Science, and IEEE Xplore databases. The search focused studies that utilizes AI evaluate PSMA PET scans. Original English language published from inception October 2024 were included, while case reports, series, commentaries, conference proceedings excluded. applications show promise automating detection metastatic disease anatomical segmentation also able predict response PSMA-based theragnostic aids tumor burden segmentation, improving radiotherapy planning. could differentiate intraprostatic PCa with higher histological grade extra-prostatic extension. has potential PCa, particularly detecting metastasis, measuring burden, high cancer, predicting treatment outcomes. Larger multicenter prospective are necessary validate enhance generalizability these models.
Language: Английский
Citations
3Medical Review, Journal Year: 2025, Volume and Issue: unknown
Published: Jan. 23, 2025
Abstract The diagnosis and prognosis of Prostate cancer (PCa) have undergone a significant transformation with the advent prostate-specific membrane antigen (PSMA)-targeted positron emission tomography (PET) imaging. PSMA-PET imaging has demonstrated superior performance compared to conventional methods by detecting PCa, its biochemical recurrence, sites metastasis higher sensitivity specificity. That now intersects rapid advances in artificial intelligence (AI) – including emergence generative AI. However, there are unique clinical challenges associated that still need be addressed ensure continued widespread integration into care research trials. Some those very wide dynamic range lesion uptake, benign uptake organs may adjacent disease, insufficient large datasets for training AI models, as well artifacts images. Generative e.g., adversarial networks, variational autoencoders, diffusion language models played crucial roles overcoming many such across various modalities, PET, computed tomography, magnetic resonance imaging, ultrasound, etc. In this review article, we delve potential role enhancing robustness utilization image analysis, drawing insights from existing literature while also exploring current limitations future directions domain.
Language: Английский
Citations
0Diagnostics, Journal Year: 2025, Volume and Issue: 15(7), P. 846 - 846
Published: March 26, 2025
Background: Approximately 50% of all oncological patients undergo radiation therapy, where personalized planning treatment relies on gross tumor volume (GTV) delineation. Manual delineation GTV is time-consuming, operator-dependent, and prone to variability. An increasing number studies apply artificial intelligence (AI) techniques automate such processes. Methods: To perform a systematic review comparing the performance AI models in delineations within body (thoracic cavity, esophagus, abdomen, pelvis, or soft tissue bone). A retrospective search five electronic databases was performed between January 2017 February 2025. Original research developing and/or validating algorithms delineating CT, MRI, PET were included. The Checklist for Artificial Intelligence Medical Imaging (CLAIM) Transparent Reporting multivariable prediction model Individual Prognosis Or Diagnosis statement checklist (TRIPOD) used assess risk, bias, reporting adherence. Results: After screening 2430 articles, 48 pooled diagnostic from use across different tumors topological areas ranged 0.62-0.92 dice similarity coefficient (DSC) 1.33-47.10 mm Hausdorff distance (HD). with highest DSC deployed an encoder-decoder architecture. Conclusions: demonstrate high level concordance clinicians Translation clinical settings requires building trust, improvement robustness results, testing prospective randomized controlled trials.
Language: Английский
Citations
0EJNMMI Physics, Journal Year: 2025, Volume and Issue: 12(1)
Published: April 16, 2025
Language: Английский
Citations
0