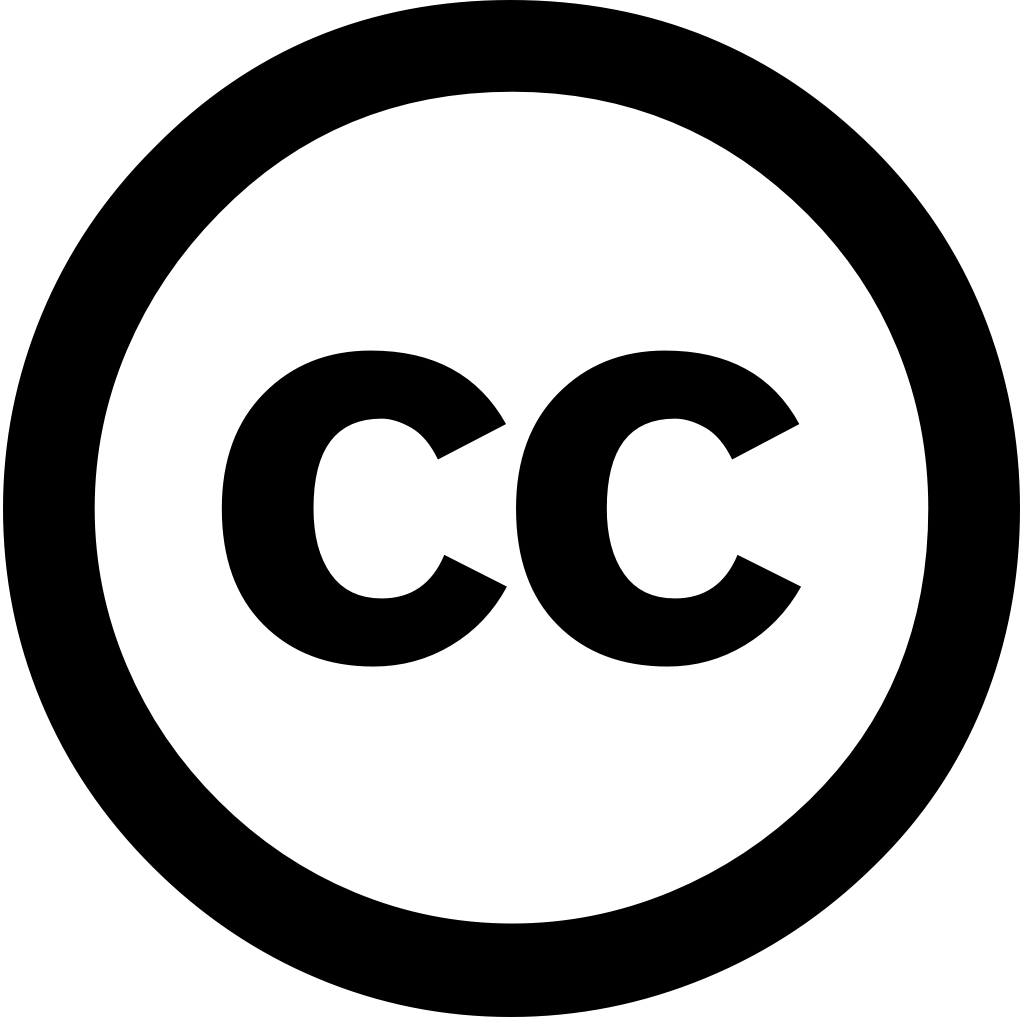
GeroScience, Journal Year: 2025, Volume and Issue: unknown
Published: May 15, 2025
Language: Английский
GeroScience, Journal Year: 2025, Volume and Issue: unknown
Published: May 15, 2025
Language: Английский
Diagnostics, Journal Year: 2025, Volume and Issue: 15(2), P. 153 - 153
Published: Jan. 10, 2025
Background: Alzheimer’s disease is a progressive neurological condition marked by decline in cognitive abilities. Early diagnosis crucial but challenging due to overlapping symptoms among impairment stages, necessitating non-invasive, reliable diagnostic tools. Methods: We applied information geometry and manifold learning analyze grayscale MRI scans classified into No Impairment, Very Mild, Moderate Impairment. Preprocessed images were reduced via Principal Component Analysis (retaining 95% variance) converted statistical manifolds using estimated mean vectors covariance matrices. Geodesic distances, computed with the Fisher Information metric, quantified class differences. Graph Neural Networks, including Convolutional Networks (GCN), Attention (GAT), GraphSAGE, utilized categorize levels graph-based representations of data. Results: Significant differences structures observed, increased variability stronger feature correlations at higher levels. distances between Impairment Mild (58.68, p<0.001) (58.28, are statistically significant. GCN GraphSAGE achieve perfect classification accuracy (precision, recall, F1-Score: 1.0), correctly identifying all instances across classes. GAT attains an overall 59.61%, variable performance Conclusions: Integrating geometry, learning, GNNs effectively differentiates AD stages from The strong indicates their potential assist clinicians early identification tracking progression.
Language: Английский
Citations
0GeroScience, Journal Year: 2025, Volume and Issue: unknown
Published: May 15, 2025
Language: Английский
Citations
0