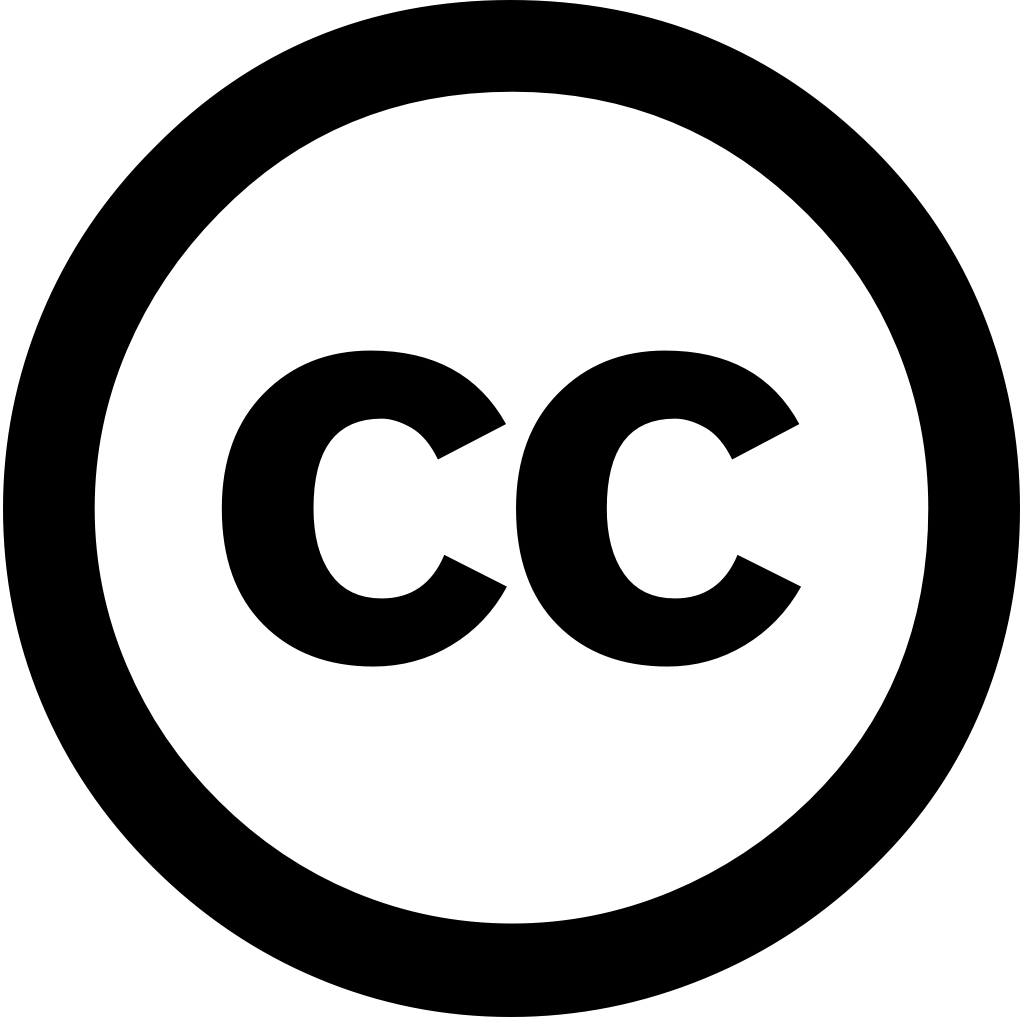
Complex & Intelligent Systems, Journal Year: 2025, Volume and Issue: 11(5)
Published: April 12, 2025
Language: Английский
Complex & Intelligent Systems, Journal Year: 2025, Volume and Issue: 11(5)
Published: April 12, 2025
Language: Английский
Renewable Energy, Journal Year: 2023, Volume and Issue: 206, P. 135 - 147
Published: Feb. 7, 2023
Language: Английский
Citations
40Heliyon, Journal Year: 2023, Volume and Issue: 9(2), P. e13287 - e13287
Published: Jan. 29, 2023
The objective of this study is to investigate and perform long-term forecasting both streamflow hydrological drought over Ethiopia. Observed precipitation data are collected from 17 stations 34 rainfall gauge forecast future 2026 2099. Streamflow performed using an artificial neural network (ANN) in conjunction with python software. 1973 2014 used train test the ANN model by 70 30% ratios, respectively. After training model, downscaled regional climate models (RCM) have been as input streamflow. Three RCM were downscale historical data. RACMO found a good downscaling for all selected stations. linear scaling bias correction technique results less than 2% error compared other alternative techniques. result indicates that tool areas having correlation between such Abbay, Awash, Baro, Omo Gibe, Tekeze river basins. But arid example Genale Dawa, Wabishebele, Rift Valley basins, not suitable because (precipitation) high variation output variable (streamflow). In areas, meteorological analysis better analysis. Finally, analyzed forecasted index (SDI). 2028, 2036, 2042, 2044, 2062, 2063 expected extreme years most basins Ethiopia future. This shows at least one each decade Therefore, extensive research needed develop effective early warning system, water resource management policy.
Language: Английский
Citations
28Water Resources Management, Journal Year: 2023, Volume and Issue: 37(11), P. 4271 - 4292
Published: July 22, 2023
Language: Английский
Citations
25Telematics and Informatics Reports, Journal Year: 2024, Volume and Issue: 16, P. 100173 - 100173
Published: Nov. 7, 2024
Language: Английский
Citations
12Computers & Industrial Engineering, Journal Year: 2024, Volume and Issue: 190, P. 110074 - 110074
Published: March 13, 2024
Language: Английский
Citations
11Water, Journal Year: 2024, Volume and Issue: 16(2), P. 289 - 289
Published: Jan. 15, 2024
Modeling and forecasting the river flow is essential for management of water resources. In this study, we conduct a comprehensive comparative analysis different models built monthly discharge Buzău River (Romania), measured in upper part river’s basin from January 1955 to December 2010. They employ convolutional neural networks (CNNs) coupled with long short-term memory (LSTM) networks, named CNN-LSTM, sparrow search algorithm backpropagation (SSA-BP), particle swarm optimization extreme learning machines (PSO-ELM). These are evaluated based on various criteria, including computational efficiency, predictive accuracy, adaptability training sets. The obtained applying CNN-LSTM stand out as top performers, demonstrating superior efficiency high especially when set containing data series 1984 (putting Siriu Dam operation) September 2006 (Model type S2). This research provides valuable guidance selecting assessing prediction models, offering practical insights scientific community real-world applications. findings suggest that Model S2 preferred choice forecast predictions due its speed accuracy. S (considering recorded 2006) recommended secondary option. S1 (with period 1955–December 1983) suitable other unavailable. study advances field by presenting precise these their respective strengths
Language: Английский
Citations
9The Journal of Supercomputing, Journal Year: 2025, Volume and Issue: 81(3)
Published: Feb. 10, 2025
Language: Английский
Citations
1Neural Networks, Journal Year: 2023, Volume and Issue: 165, P. 953 - 970
Published: July 5, 2023
This paper shows that time series forecasting Transformer (TSFT) suffers from severe over-fitting problem caused by improper initialization method of unknown decoder inputs, especially when handling non-stationary series. Based on this observation, we propose GBT, a novel two-stage framework with Good Beginning. It decouples the prediction process TSFT into two stages, including Auto-Regression stage and Self-Regression to tackle different statistical properties between input sequences. Prediction results serve as 'Good Beginning', i.e., better for inputs stage. We also Error Score Modification module further enhance capability in GBT. Extensive experiments seven benchmark datasets demonstrate GBT outperforms SOTA TSFTs (FEDformer, Pyraformer, ETSformer, etc.) many other models (SCINet, N-HiTS, only canonical attention convolution while owning less space complexity. is general enough couple these strengthen their capability. The source code available at: https://github.com/OrigamiSL/GBT.
Language: Английский
Citations
20Applied Energy, Journal Year: 2023, Volume and Issue: 353, P. 122092 - 122092
Published: Oct. 17, 2023
Language: Английский
Citations
20AIMS Mathematics, Journal Year: 2024, Volume and Issue: 9(4), P. 9419 - 9434
Published: Jan. 1, 2024
<abstract> <p>The adjusted precision of economic values is essential in the global economy. In recent years, researchers have increased their interest making accurate predictions this type time series; one reasons that characteristics series makes predicting a complicated task due to its non-linear nature. The evolution artificial neural network models enables us research suitability generated for other purposes, applying potential prediction with promising results. Specifically, field, application transformer assuming an innovative approach great To improve performance networks, work, empirical model decomposition (EMD) methodology was used as data preprocessing network. results confirmed better compared networks widely bidirectional long short term memory (BiLSTM), and (LSTM) using without EMD preprocessing, well comparison Transformer data, lower error all metrics used: root mean square (RMSE), (MSE), absolute percentage (MAPE), R-square (R<sup>2</sup>). Finding provides literature allows greater adjustment minimal preprocessing.</p> </abstract>
Language: Английский
Citations
6