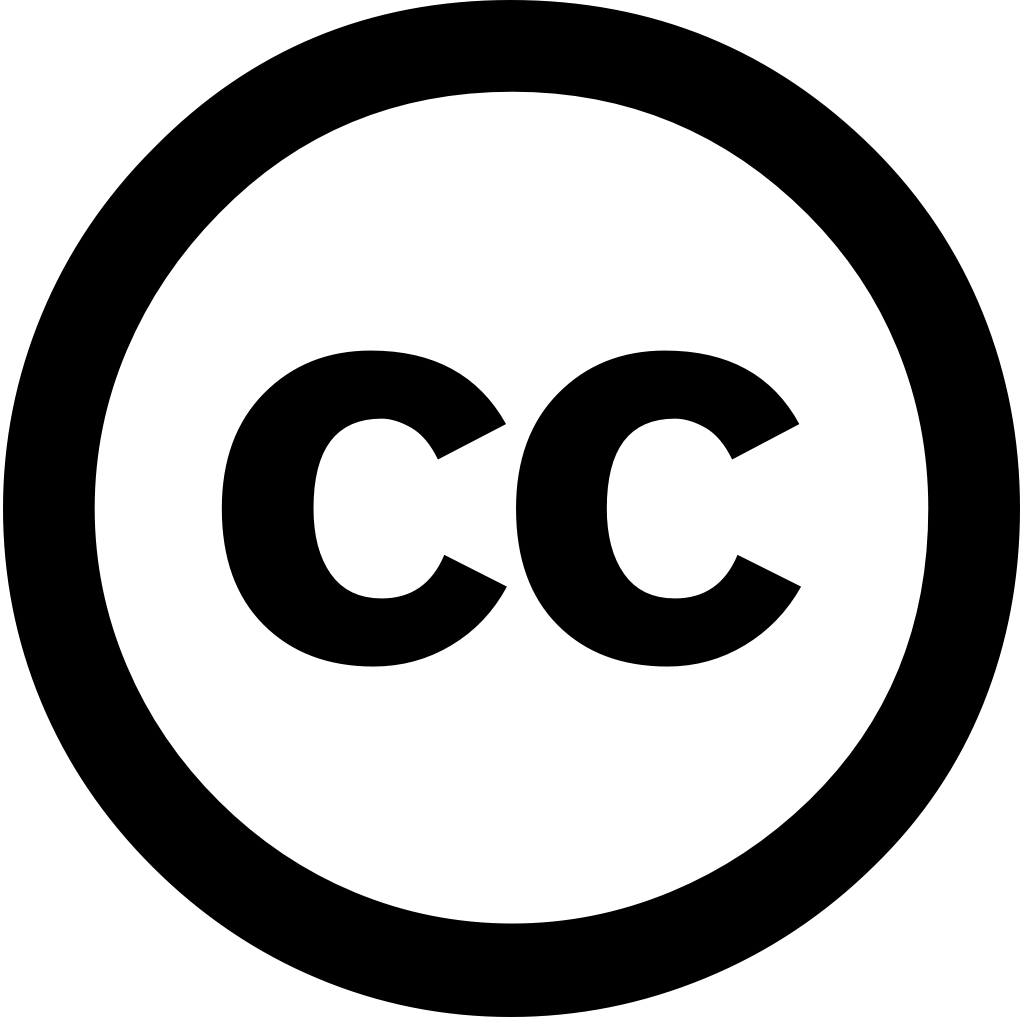
Scientific Reports, Journal Year: 2025, Volume and Issue: 15(1)
Published: April 9, 2025
Language: Английский
Scientific Reports, Journal Year: 2025, Volume and Issue: 15(1)
Published: April 9, 2025
Language: Английский
Scientific Reports, Journal Year: 2024, Volume and Issue: 14(1)
Published: July 11, 2024
Abstract Crop diseases can significantly affect various aspects of crop cultivation, including yield, quality, production costs, and loss. The utilization modern technologies such as image analysis via machine learning techniques enables early precise detection diseases, hence empowering farmers to effectively manage avoid the occurrence diseases. proposed methodology involves use modified MobileNetV3Large model deployed on edge device for real-time monitoring grape leaf disease while reducing computational memory demands ensuring satisfactory classification performance. To enhance applicability MobileNetV3Large, custom layers consisting two dense were added, each followed by a dropout layer, helped mitigate overfitting ensured that remains efficient. Comparisons among other models showed outperformed those with an average train test accuracy 99.66% 99.42%, precision, recall, F1 score approximately 99.42%. was (Nvidia Jetson Nano) using developed GUI app predicted from both saved data high confidence values. Grad-CAM visualization used identify represent areas convolutional neural network (CNN) decision-making process accuracy. This research contributes development plant devices, which have potential ability autonomous farming farmers, agronomists, researchers monitor efficiently effectively, positive impact global food security.
Language: Английский
Citations
17Artificial Intelligence Review, Journal Year: 2025, Volume and Issue: 58(3)
Published: Jan. 17, 2025
Abstract Plant diseases cause significant damage to agriculture, leading substantial yield losses and posing a major threat food security. Detection, identification, quantification, diagnosis of plant are crucial parts precision agriculture crop protection. Modernizing improving production efficiency significantly affected by using computer vision technology for disease diagnosis. This is notable its non-destructive nature, speed, real-time responsiveness, precision. Deep learning (DL), recent breakthrough in vision, has become focal point agricultural protection that can minimize the biases manually selecting spot features. study reviews techniques tools used automatic state-of-the-art DL models, trends DL-based image analysis. The techniques, performance, benefits, drawbacks, underlying frameworks, reference datasets more than 278 research articles were analyzed subsequently highlighted accordance with architecture deep models. Key findings include effectiveness imaging sensors like RGB, multispectral, hyperspectral cameras early detection. Researchers also evaluated various architectures, such as convolutional neural networks, transformers, generative adversarial language foundation Moreover, connects academic practical applications, providing guidance on suitability these models environments. comprehensive review offers valuable insights into current state future directions detection, making it resource researchers, academicians, practitioners agriculture.
Language: Английский
Citations
4Horticulturae, Journal Year: 2024, Volume and Issue: 10(3), P. 197 - 197
Published: Feb. 20, 2024
Artificial intelligence (AI) is revolutionizing approaches in plant disease management and phytopathological research. This review analyzes current applications future directions of AI addressing evolving agricultural challenges. Plant diseases annually cause 10–16% yield losses major crops, prompting urgent innovations. shows an aptitude for automated detection diagnosis utilizing image recognition techniques, with reported accuracies exceeding 95% surpassing human visual assessment. Forecasting models integrating weather, soil, crop data enable preemptive interventions by predicting spatial-temporal outbreak risks weeks advance at 81–95% precision, minimizing pesticide usage. Precision agriculture powered optimizes data-driven, tailored protection strategies boosting resilience. Real-time monitoring leveraging discerns pre-symptomatic anomalies from environmental early alerts. These highlight AI’s proficiency illuminating opaque patterns within increasingly complex data. Machine learning techniques overcome cognitive constraints discovering multivariate correlations unnoticed before. poised to transform in-field decision-making around prevention precision management. Overall, constitutes a strategic innovation pathway strengthen ecological health amidst climate change, globalization, intensification pressures. With prudent ethical implementation, AI-enabled tools promise next-generation phytopathology, enhancing resilience worldwide.
Language: Английский
Citations
10Scientific Reports, Journal Year: 2025, Volume and Issue: 15(1)
Published: Jan. 28, 2025
In agriculture, promptly and accurately identifying leaf diseases is crucial for sustainable crop production. To address this requirement, research introduces a hybrid deep learning model that combines the visual geometric group version 19 (VGG19) architecture features with transformer encoder blocks. This fusion enables accurate précised real-time classification of affecting grape, bell pepper, tomato plants. Incorporating blocks offers enhanced capability in capturing intricate spatial dependencies within images, promising agricultural sustainability food security. By providing farmers farming stakeholders reliable tool rapid disease detection, our facilitates timely intervention management practices, ultimately leading to improved yields mitigated economic losses. Through extensive comparative analyses on various datasets filed tests, proposed depth wise separable convolutional-TransNet (DSC-TransNet) has demonstrated higher performance terms accuracy (99.97%), precision (99.94%), recall (99.94), sensitivity F1-score AUC (0.98) Grpae leaves across different including pepper tomato. Furthermore, DSC layers enhances computational efficiency while maintaining expressive power, making it well-suited applications. The developed DSC-TransNet deployed NVIDIA Jetson Nano single board computer. contributes advancing field automated plant classification, addressing critical challenges modern agriculture promoting more efficient practices.
Language: Английский
Citations
1IEEE Journal of Selected Topics in Applied Earth Observations and Remote Sensing, Journal Year: 2024, Volume and Issue: 17, P. 7260 - 7277
Published: Jan. 1, 2024
Globally, pests and plant diseases severely threaten forestry agriculture. Plant protection could be substantially enhanced by using non-contact, extremely effective, reasonably priced techniques for identifying tracking across large geographic areas. Precision agriculture is the study of other technologies, such as hyperspectral remote sensing (RS), to increase cultivation instead traditional agricultural methods with less negative environmental effects. In this work, we proposed a novel deep-learning architecture optimization algorithm crop leaf disease recognition. initial step, multilevel contrast enhancement technique better visual on leaves cotton wheat. After that, three residual block self-attention mechanisms named 3-RBNet Self, 5-RBNet 9-RBNet Self. models are trained images later extracted deep features from layer. The 5-RBNET Self 9-RBNET performed well in terms accuracy precision rate; therefore, did not consider 3-RBNET next process. dragonfly best feature selection applied improve classification performance further reduce computational cost. method evaluated two publically available images, Cotton, Wheat, EuroSAT datasets. For both crops, obtained maximum 98.60 93.90%, respectively, whereas EuroSAT, an 83.10%. Compared results recent techniques, shows improved rate.
Language: Английский
Citations
5Frontiers in Plant Science, Journal Year: 2024, Volume and Issue: 15
Published: May 17, 2024
Tomato is one of the most popular and important food crops consumed globally. The quality quantity yield by tomato plants are affected impact made various kinds diseases. Therefore, it essential to identify these diseases early so that possible reduce occurrences effect on improve overall crop support farmers. In past, many research works have been carried out applying machine learning techniques segment classify leaf images. However, existing learning-based classifiers not able detect new types more accurately. On other hand, deep with swarm intelligence-based optimization enhance classification accuracy, leading effective accurate detection This paper proposes a method for harnessing power an ensemble model in sample dataset plants, containing images pertaining nine different introduces exponential moving average function temporal constraints enhanced weighted gradient optimizer integrated into fine-tuned Visual Geometry Group-16 (VGG-16) Neural Architecture Search Network (NASNet) mobile training methods providing improved accuracy. used consists 10,000 categorized classes validating additional 1,000 reserved testing model. results analyzed thoroughly benchmarked performance metrics, thus proving proposed approach gives better terms loss, precision, recall, receiver operating characteristic curve, F1-score values 98.7%, 4%, 97.9%, 98.6%, 99.97%, respectively.
Language: Английский
Citations
5Lecture notes in networks and systems, Journal Year: 2025, Volume and Issue: unknown, P. 235 - 248
Published: Jan. 1, 2025
Language: Английский
Citations
0Hybrid Advances, Journal Year: 2025, Volume and Issue: unknown, P. 100440 - 100440
Published: March 1, 2025
Language: Английский
Citations
0Journal of Agricultural and Food Chemistry, Journal Year: 2025, Volume and Issue: unknown
Published: April 10, 2025
Paeonol is the main active ingredient in Chinese medicinal herb peony root bark. To discover biorational natural product-based fungicides, 25 paeonol derivatives (5a-y) containing a pyrazole ring were designed and synthesized by ingeniously introducing five-membered heterocyclic ring, their structures well characterized 1H NMR, 13C high-resolution mass spectrometer (HRMS), m.p. The stereochemical configurations of six target compounds, 5c, 5f, 5g, 5o, 5s, 5v, unambiguously subjected to single-crystal X-ray diffraction. Furthermore, we evaluated bioactivities these compounds as anti-oomycete antifungal agents against two serious agricultural diseases, Phytophthora capsici Fusarium graminearum. Among all tested (1) nine 5b-d, 5r, 5u, 5w displayed promising P. capsici, with EC50 values ranging from 22.1 34.5 mg/L, protective effect vivo further confirmed above results. (2) Six 5k, 5m, 5t, 5w, 5x F. graminearum, 38.2 86.0 mg/L. Moreover, some interesting results structure-activity relationships also observed. this study pave way for design, synthesis, development potential botanical crop protection.
Language: Английский
Citations
0Published: Aug. 6, 2024
Artificial intelligence (AI) is revolutionizing plant sciences by enabling precise species identification, early disease diagnosis, crop yield prediction, and precision agriculture optimization. AI uses machine learning image recognition to aid ecological research biodiversity conservation. It plays a crucial role in breeding accelerating the development of resilient, high-yielding crops with desirable traits. models using climate soil data contribute sustainable food security. In phenotyping, automates measurement analysis characteristics, enhancing our understanding growth. Ongoing aims improve models’ robustness interpretability while addressing privacy algorithmic biases. Interdisciplinary collaboration essential fully harness AI’s potential for sustainable, food-secure future.
Language: Английский
Citations
4