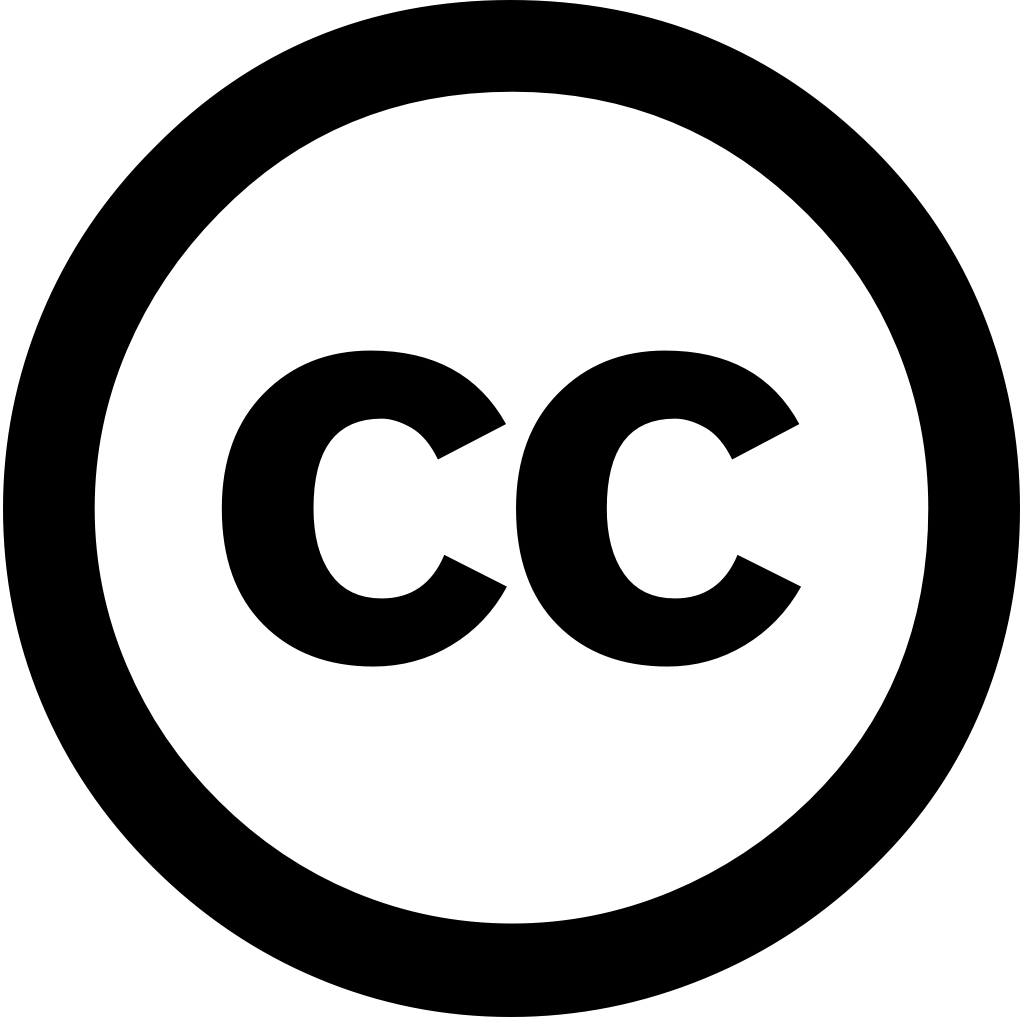
Scientific Reports, Journal Year: 2025, Volume and Issue: 15(1)
Published: April 9, 2025
Language: Английский
Scientific Reports, Journal Year: 2025, Volume and Issue: 15(1)
Published: April 9, 2025
Language: Английский
Scientific Reports, Journal Year: 2025, Volume and Issue: 15(1)
Published: Jan. 10, 2025
Abstract The hairiness of the leaves is an essential morphological feature within genus Vitis that can serve as a physical barrier. A high leaf hair density present on abaxial surface grapevine influences their wettability by repelling forces, thus preventing pathogen attack such downy mildew and anthracnose. Moreover, hairs favorable habitat may considerably affect abundance biological control agents. unavailability accurate efficient objective tools for quantifying makes study intricate challenging. Therefore, validated high-throughput phenotyping tool was developed established in order to detect quantify using images single discs convolution neural networks (CNN). We trained modified ResNet CNNs with minimalistic number efficiently classify area covered hairs. This approach achieved overall model prediction accuracy 95.41%. As final validation, 10,120 input from segregating F1 biparental population were used evaluate algorithm performance. CNN-based phenotypic results compared ground truth data received two experts revealed strong correlation R values 0.98 0.92 root-mean-square error 8.20% 14.18%, indicating performance consistent expert evaluations outperforms traditional manual rating. Additional validation between vs. non-expert six varieties showed non-experts contributed over- underestimation trait, absolute 0% 30% -5% -60%, respectively. Furthermore, panel 16 novice evaluators produced significant bias set varieties. Our provide clear evidence need hairiness.
Language: Английский
Citations
0SSRN Electronic Journal, Journal Year: 2025, Volume and Issue: unknown
Published: Jan. 1, 2025
Language: Английский
Citations
0Biology, Journal Year: 2025, Volume and Issue: 14(1), P. 99 - 99
Published: Jan. 19, 2025
Early detection of plant diseases is crucial for agro-holdings, farmers, and smallholders. Various neural network architectures training methods have been employed to identify optimal solutions disease classification. However, research applying one-shot or few-shot learning approaches, based on similarity determination, the plantdisease classification domain remains limited. This study evaluates different loss functions used in learning, including Contrastive, Triplet, Quadruplet, SphereFace, CosFace, ArcFace, alongside various backbone networks, such as MobileNet, EfficientNet, ConvNeXt, ResNeXt. Custom datasets real-life images, comprising over 4000 samples across 68 classes diseases, pests, their effects, were utilized. The experiments evaluate standard transfer approaches two function. Results demonstrate superiority cosine-based Siamese networks embedding extraction Effective model organization are determined. Additionally, impact data normalization tested, generalization ability models assessed using a special dataset consisting 400 images difficult-to-identify cases.
Language: Английский
Citations
0Deleted Journal, Journal Year: 2025, Volume and Issue: 3(1), P. 8 - 14
Published: Jan. 24, 2025
Plants are essential for human survival. However, diseases affecting plant leaves can lead to significant reductions in crop yield and economic losses. Detecting these early is crucial agriculture. To overcome limitations, machine learning has been employed automate the identification of leaf diseases. By analysing features such as colour, intensity, shape, models classify into specific categories, offering faster more accurate results than conventional approaches. Various ML techniques used identify leaves, with deep gaining attention its ability perform advanced feature extraction. CNNs have become a highly effective tool disease identification, thanks their automatically extract from images achieve high classification accuracy. Their hierarchical structure enables them detect simple patterns initial layers progressively learn complex deeper layers, capturing intricate details symptoms. Additionally, process large datasets multiple accurately, even limited labelled data, by leveraging pre-trained through transfer learning.
Language: Английский
Citations
0Research Square (Research Square), Journal Year: 2025, Volume and Issue: unknown
Published: Feb. 5, 2025
Language: Английский
Citations
0IGI Global eBooks, Journal Year: 2025, Volume and Issue: unknown, P. 267 - 296
Published: Feb. 7, 2025
Automated plant disease detection using computer vision has transformed agriculture by addressing challenges in health management, productivity, and sustainability. This chapter explores advancements from traditional methods to AI-enhanced deep learning multi-modal imaging, enabling early detection, real-time processing, precise interventions. Applications like precision agriculture, IoT integration, data-driven decision-making foster eco-friendly practices resource efficiency. Despite such as data quality, scalability, accessibility, future innovations collection, sustainable hardware, collaboration promise shape resilient agricultural systems. By aligning technology with sustainability, automated supports food security, environmental conservation, the evolution of modern farming practices.
Language: Английский
Citations
0Communications in computer and information science, Journal Year: 2025, Volume and Issue: unknown, P. 81 - 92
Published: Jan. 1, 2025
Language: Английский
Citations
0Agronomy, Journal Year: 2025, Volume and Issue: 15(3), P. 582 - 582
Published: Feb. 26, 2025
The conventional hand-picking of tea buds is inefficient and leads to inconsistent quality. Innovations in bud identification automated grading are essential for enhancing industry competitiveness. Key breakthroughs include detection accuracy lightweight model deployment. Traditional image recognition struggles with variable weather conditions, while high-precision models often too bulky mobile applications. This study proposed a YOLOV5 model, which was tested on three types across different scenarios. It incorporated convolutional network compact feature extraction layer, significantly reduced parameter computation. achieved 92.43% precision 87.25% mean average (mAP), weighing only 4.98 MB improving by 6.73% 2.11% reducing parameters 2 141.02 compared YOLOV5n6 YOLOV5l6. Unlike networks that detected single or dual grades, this offered refined advantages both size, making it suitable embedded devices limited resources. Thus, the YOLOV5n6_MobileNetV3 enhanced supported intelligent harvesting research technology.
Language: Английский
Citations
0Plants, Journal Year: 2025, Volume and Issue: 14(5), P. 733 - 733
Published: Feb. 27, 2025
In rice, infections caused by Pantoea ananatis or Enterobacter asburiae closely resemble the bacterial blight induced Xanthomonas oryzae pv. oryzae, yet they differ in drug resistance and management strategies. This study explores potential of combining hyperspectral imaging (HSI) with machine learning for rapid accurate detection rice symptoms various pathogens. One-dimensional convolutional neural networks (1DCNNs) were employed to construct a classification model, integrating spectral preprocessing techniques feature selection algorithms comparison. To enhance model robustness mitigate overfitting due limited samples, generative adversarial (GANs) utilized augment dataset. The results indicated that 1DCNN after using uninformative variable elimination (UVE), achieved an accuracy 86.11% F1 score 0.8625 on five-class However, dominance mixed samples negatively impacted performance. After removing mixed-infection attained 97.06% 0.9703 four-class dataset, demonstrating high across different pathogen-induced infections. Key bands identified at 420–490 nm, 610–670 780–850 910–940 facilitating pathogen differentiation. presents precise, non-destructive approach plant disease detection, offering valuable insights into prevention precision agriculture.
Language: Английский
Citations
0Lecture notes in electrical engineering, Journal Year: 2025, Volume and Issue: unknown, P. 489 - 497
Published: Jan. 1, 2025
Language: Английский
Citations
0