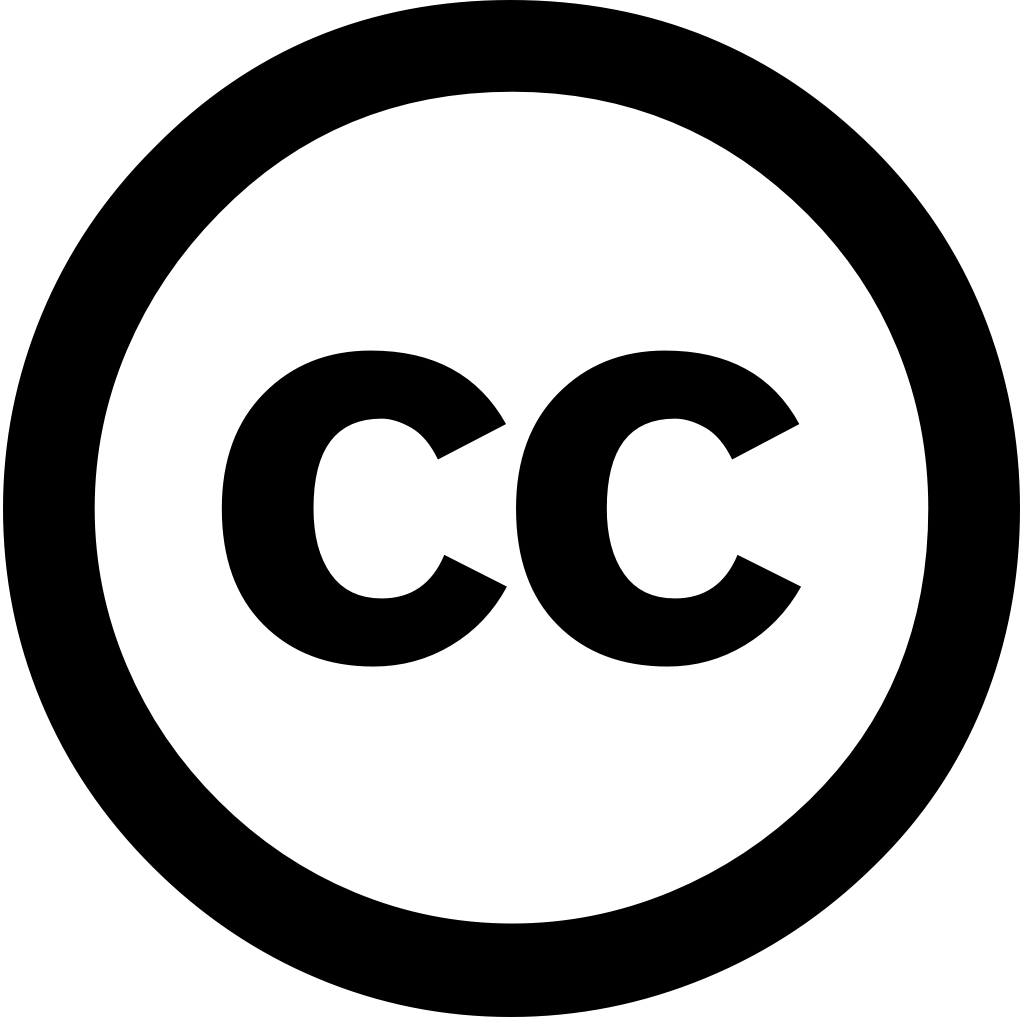
Computers in Human Behavior, Journal Year: 2024, Volume and Issue: unknown, P. 108546 - 108546
Published: Dec. 1, 2024
Language: Английский
Computers in Human Behavior, Journal Year: 2024, Volume and Issue: unknown, P. 108546 - 108546
Published: Dec. 1, 2024
Language: Английский
PNAS Nexus, Journal Year: 2025, Volume and Issue: 4(2)
Published: Feb. 1, 2025
Abstract Misinformation disrupts our information ecosystem, adversely affecting individuals and straining social cohesion democracy. Understanding what causes online (mis)information to (re)appear is crucial for fortifying ecosystem. We analyzed a large-scale Twitter (now “X”) dataset of about 2 million tweets across 123 fact-checked stories. Previous research suggested falsehood effect (false reappears more frequently) an ambiguity (ambiguous frequently). However, robust indicators their existence remain elusive. Using polynomial statistical modeling, we compared model, dual model. The data supported the model (13.76 times as likely null model), indicating both promote reappearance. evidence was stronger: 6.6 Various control checks affirmed effect, while less stable. Nonetheless, best-fitting explained <7% variance, that (i) dynamics are complex (ii) effects may play smaller role than previous has suggested. These findings underscore importance understanding (mis)information, though focus on stories limit generalizability full spectrum shared online. Even so, results can inform policymakers, journalists, media platforms, public in building resilient environment, also opening new avenues research, including source credibility, cross-platform applicability, psychological factors.
Language: Английский
Citations
0Trends in Cognitive Sciences, Journal Year: 2025, Volume and Issue: unknown
Published: Feb. 1, 2025
Language: Английский
Citations
0Mathematics, Journal Year: 2024, Volume and Issue: 12(12), P. 1806 - 1806
Published: June 11, 2024
Universities increasingly leverage social media platforms, especially Twitter, for news dissemination, audience engagement, and feedback collection. King Faisal University (KFU) is dedicated to enhancing its institutional identity (ID), grounded in environmental sustainability food security, encompassing nine critical areas. This study aims assess the impact of KFU’s Twitter interactions on public awareness using systematic analysis machine learning (ML) methods. The objectives are to: (1) Determine influence presence ID awareness; (2) create a dataset real-time interaction with content; (3) investigate Twitter’s role promoting across 9-ID domains changing over time; (4) utilize k-means clustering sentiment (TFIDF Word2vec) classify data similarities among domains; (5) apply categorization method process categorize tweets, facilitating assessment word meanings domains. also employs four ML models, including Logistic Regression (LR) Support Vector Machine (SVM), Random Forest (RF) model combined Word2vec achieving highest accuracy 100%. findings underscore value deepening understanding guiding development effective communication strategies.
Language: Английский
Citations
1Computers in Human Behavior, Journal Year: 2024, Volume and Issue: unknown, P. 108546 - 108546
Published: Dec. 1, 2024
Language: Английский
Citations
1