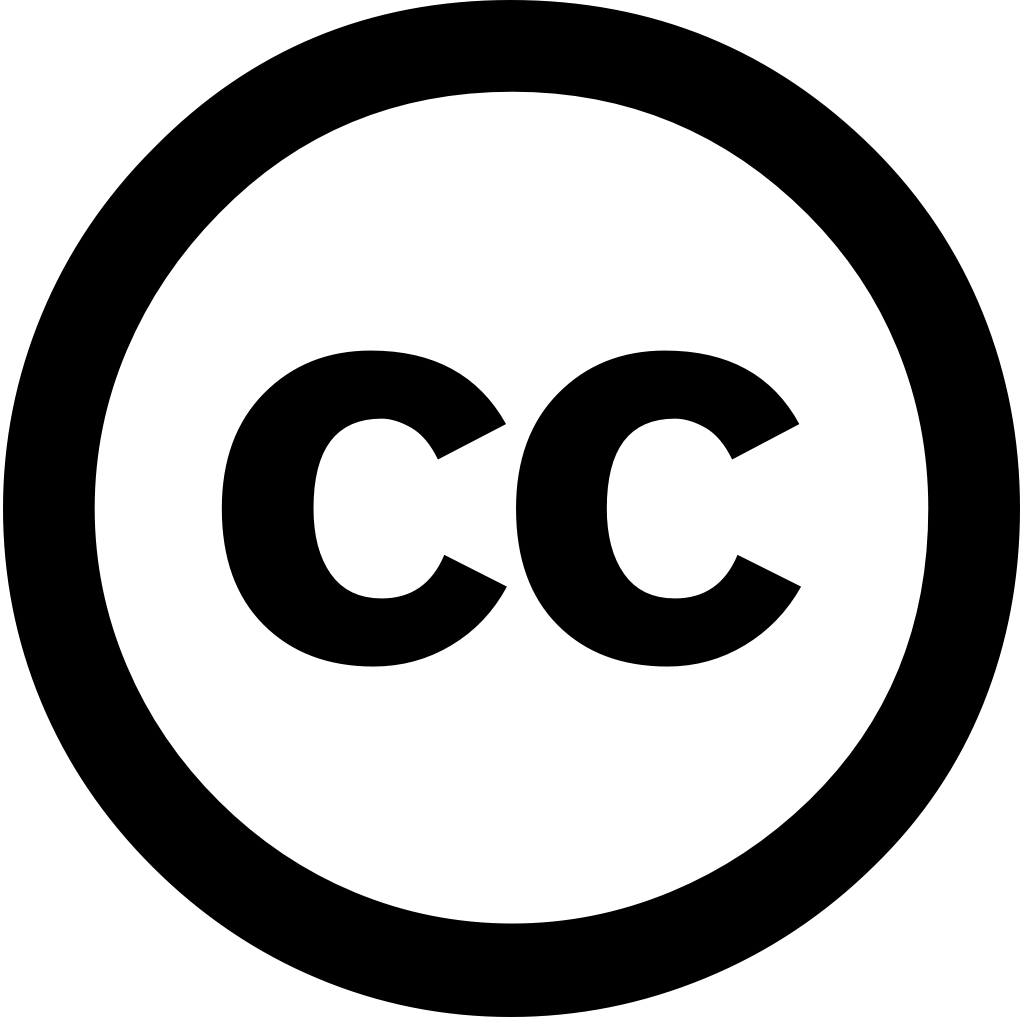
Scientific Reports, Journal Year: 2025, Volume and Issue: 15(1)
Published: Jan. 18, 2025
In recent years, deep neural networks (DNNs) have demonstrated remarkable performance in pathology applications, potentially even outperforming expert pathologists due to their ability learn subtle features from large datasets. One complication preparing digital datasets for DNN tasks is the variation tinctorial qualities. A common way address this perform stain normalization on images. study, we show that a well-trained model trained one batch of histological slides failed generalize another prepared at different time same tissue blocks, when methods were applied. This study used sample data previously reported was able identify patients with early-stage non-small cell lung cancer (NSCLC) whose tumors did and not metastasize, high accuracy, based training then testing images H&E stained primary tumor sections processed time. obtained new series histologic adjacent recuts blocks lab but We found either slides/images unable predict progression other (AUCcross-batch = 0.52 - 0.53 compared AUCsame-batch 0.74 0.81). The failure improve difference corrections made through traditional color-tuning or help Cycle Generative Adversarial Network (CycleGAN) process. highlights need develop an entirely process collect consistent microscopy can be both train allow general application predictive algorithms.
Language: Английский