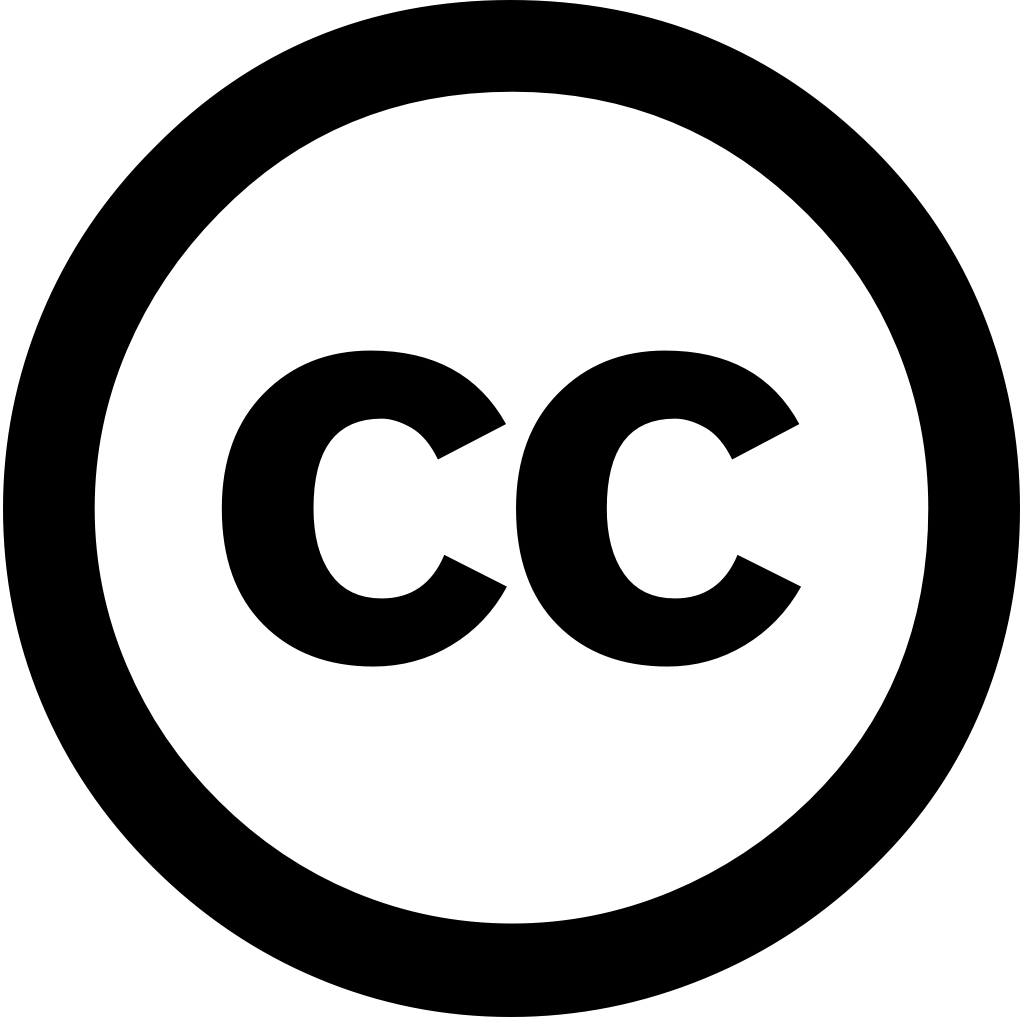
Case Studies in Construction Materials, Journal Year: 2025, Volume and Issue: 22, P. e04473 - e04473
Published: March 3, 2025
Language: Английский
Case Studies in Construction Materials, Journal Year: 2025, Volume and Issue: 22, P. e04473 - e04473
Published: March 3, 2025
Language: Английский
Journal of Water Process Engineering, Journal Year: 2024, Volume and Issue: 58, P. 104789 - 104789
Published: Jan. 17, 2024
Language: Английский
Citations
69Engineering Applications of Artificial Intelligence, Journal Year: 2024, Volume and Issue: 133, P. 108573 - 108573
Published: May 11, 2024
Understanding the effects of extreme floods on critical infrastructures such as bridges is paramount for ensuring safety and resilient design in face climate change events. This study develops robust computational predictive modeling tools assessing impacts hydraulic response structural resilience bridges. A fluid dynamic (CFD) model utilizing RANS equations k-ω Shear Stress Transport (SST) simulating supercritical flows adopted to compute hydrodynamic pressures water levels bridge piers cylindrical rectangular shapes during a flood event. The CFD validated based case data obtained from Haj Omran Bridge, built Khorramabad River Iran. numerical simulations consider hydrological conditions exclude geotechnical parameters abutment damages. results are evaluated well-established guidelines. Machine learning techniques, including Extreme Gradient Boosting (XGBoost), Random Forest (RF), Support Vector Regression (SVR), optimized with Grid Search Cross-Validation (GSCV), enhance accuracy pressure forecasting at piers. XGBoost exhibits superior performance (R2 = 0.908, RMSE 0.0279, E 3.41%) compared RF SVR models. All estimated by falls within ±6 percent error lines, highlighting model's robustness out-of-range prediction. Additionally, an Long Short-Term Memory (LSTM) effectively predict free surface flow profiles (i.e. depth) over 0.937 0.083), demonstrating its potential practical applications depth predictions infrastructures. proposed methodological framework outlined this can facilitate bridges, enabling assessment
Language: Английский
Citations
42Frontiers in Built Environment, Journal Year: 2024, Volume and Issue: 10
Published: Feb. 9, 2024
Seawalls are critical defence infrastructures in coastal zones that protect hinterland areas from storm surges, wave overtopping and soil erosion hazards. Scouring at the toe of sea defences, caused by wave-induced accretion bed material imposes a significant threat to structural integrity infrastructures. Accurate prediction scour depths is essential for appropriate efficient design maintenance structures, which serve mitigate risks failure through scouring. However, limited guidance predictive tools available estimating scouring sloping structures. In recent years, Artificial Intelligence Machine Learning (ML) algorithms have gained interest, although they underpin robust models many engineering applications, such yet be applied prediction. Here we develop present ML-based predicting seawall. Four ML algorithms, namely, Random Forest (RF), Gradient Boosted Decision Trees (GBDT), Neural Networks (ANNs), Support Vector Regression (SVMR) utilised. Comprehensive physical modelling measurement data utilised validate models. A Novel framework feature selection, importance, hyperparameter tuning adopted pre- post-processing steps In-depth statistical analyses proposed evaluate performance The results indicate minimum 80% accuracy across all tested this study overall, SVMR produced most accurate predictions with Coefficient Determination ( r 2 ) 0.74 Mean Absolute Error (MAE) value 0.17. algorithm also offered computationally among tested. methodological can datasets rapid assessment facilitating model-informed decision-making.
Language: Английский
Citations
25Scientific Reports, Journal Year: 2024, Volume and Issue: 14(1)
Published: March 29, 2024
Abstract The consumption of water constitutes the physical health most living species and hence management its purity quality is extremely essential as contaminated has to potential create adverse environmental consequences. This creates dire necessity measure, control monitor water. primary contaminant present in Total Dissolved Solids (TDS), which hard filter out. There are various substances apart from mere solids such potassium, sodium, chlorides, lead, nitrate, cadmium, arsenic other pollutants. proposed work aims provide automation estimation through Artificial Intelligence uses Explainable (XAI) for explanation significant parameters contributing towards potability impurities. XAI transparency justifiability a white-box model since Machine Learning (ML) black-box unable describe reasoning behind ML classification. models Logistic Regression, Support Vector (SVM), Gaussian Naive Bayes, Decision Tree (DT) Random Forest (RF) classify whether drinkable. representations force plot, test patch, summary dependency plot decision generated SHAPELY explainer explain features, prediction score, feature importance justification estimation. RF classifier selected yields optimum Accuracy F1-Score 0.9999, with Precision Re-call 0.9997 0.998 respectively. Thus, an exploratory analysis indicators associated their significance. emerging research at vision addressing future well.
Language: Английский
Citations
24Journal of Hydrology, Journal Year: 2025, Volume and Issue: unknown, P. 132905 - 132905
Published: Feb. 1, 2025
Language: Английский
Citations
2Engineering Applications of Artificial Intelligence, Journal Year: 2024, Volume and Issue: 133, P. 108156 - 108156
Published: March 6, 2024
Ground settlement prediction during mechanized tunneling is of paramount importance and remains a challenging research topic. Typically, two paradigms are existing: physics-driven approach utilizing numerical simulation models for prediction, data-driven employing machine learning techniques to learn mappings between influencing factors the settlement. To integrate advantages both approaches assimilate data from different sources, we propose multi-fidelity deep operator network (DeepONet) framework, leveraging recently developed methods. The presented framework comprises components: low-fidelity subnet that captures fundamental ground patterns obtained finite element simulations, high-fidelity learns nonlinear correlation real engineering monitoring data. A pre-processing strategy causality adopted consider spatio-temporal characteristics tunnel excavation. results show proposed method can effectively capture physical information provided by simulations accurately fit measured (R2 around 0.9) as well. Notably, even when dealing with very limited noisy (with 50% error), model robust, achieving satisfactory R2>0.8. In comparison, R2 score pure simulation-based only 0.2. utilization transfer significantly reduces training time 20 min within 30 s, showcasing potential our real-time construction.
Language: Английский
Citations
14Ocean Engineering, Journal Year: 2024, Volume and Issue: 311, P. 118756 - 118756
Published: Aug. 13, 2024
Language: Английский
Citations
13Journal of Water Process Engineering, Journal Year: 2024, Volume and Issue: 58, P. 104778 - 104778
Published: Jan. 8, 2024
Language: Английский
Citations
10Results in Engineering, Journal Year: 2025, Volume and Issue: unknown, P. 104254 - 104254
Published: Feb. 1, 2025
Language: Английский
Citations
1Ocean Modelling, Journal Year: 2025, Volume and Issue: unknown, P. 102510 - 102510
Published: Feb. 1, 2025
Language: Английский
Citations
1