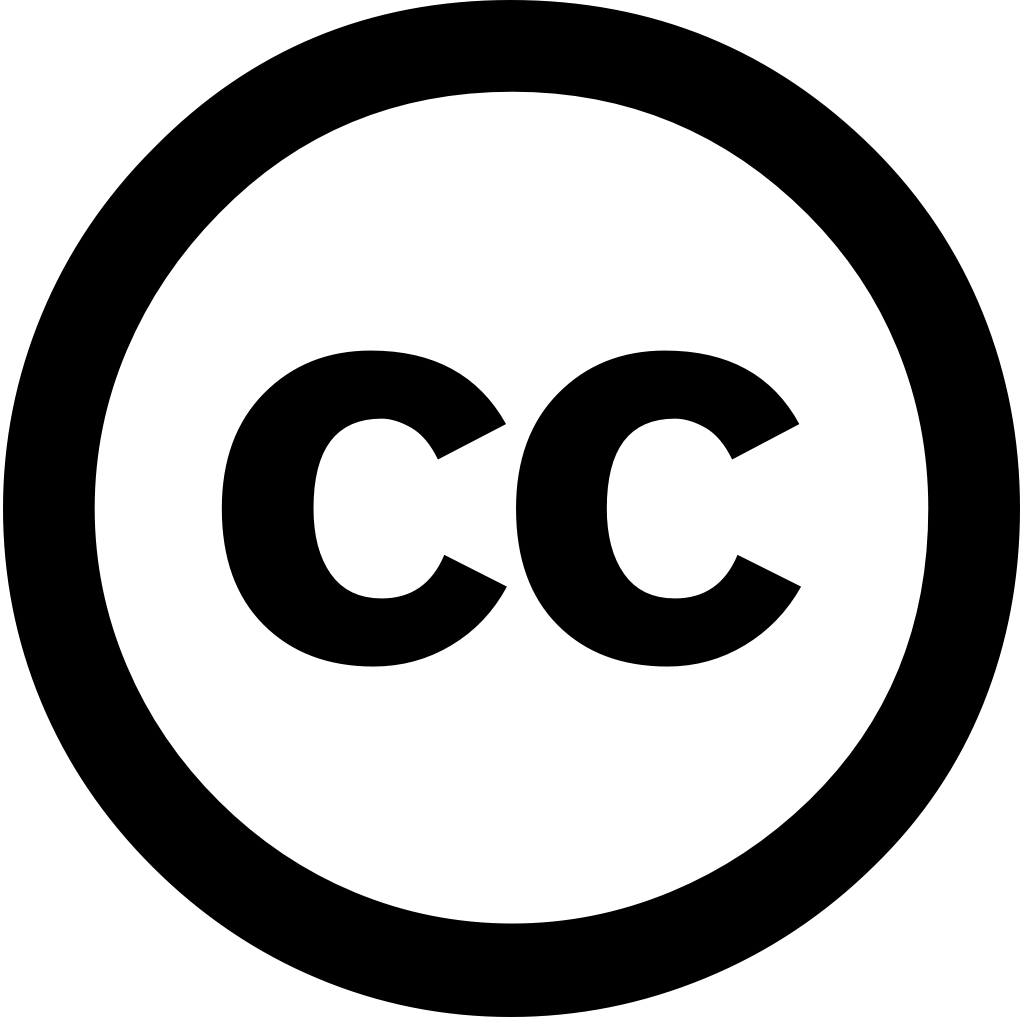
IET Radar Sonar & Navigation, Journal Year: 2024, Volume and Issue: unknown
Published: Nov. 6, 2024
Abstract Automation and self‐sufficiency in the complex environment of modern electronic warfare (EW) are critical necessary issues intelligence support systems to detect real‐time accurate threat radars. The task these is search, discover, analyse, identify parameters radar signals. However, recognition pulse repetition interval (PRI) modulation challenging natural environments due destructive factors, including missing pulses (MP), spurious (SP), large outliers (LO) (caused by antenna scanning), which lead noisy sequences PRI variation patterns. current article examines effects factors on recognising signals using deep convolutional neural networks (DCNNs). uses simulations based actual generate data consider with different percentages. number images obtained applying sum for each range (with percentages) considered 30,000. It common six types modulation. Then, DCNN models, VGG16, ResNet50V2, InceptionV3, Xception, MobileNetV2, trained transfer learning method. simulation results show that accuracy training testing models decreases significantly increase percentage factors. Also, model type performance have been investigated, shown some more resistant destruction retain accuracy. Finally, this analysis shows improve network (DNN) techniques face changes caused it pay attention apply appropriate strategies.
Language: Английский