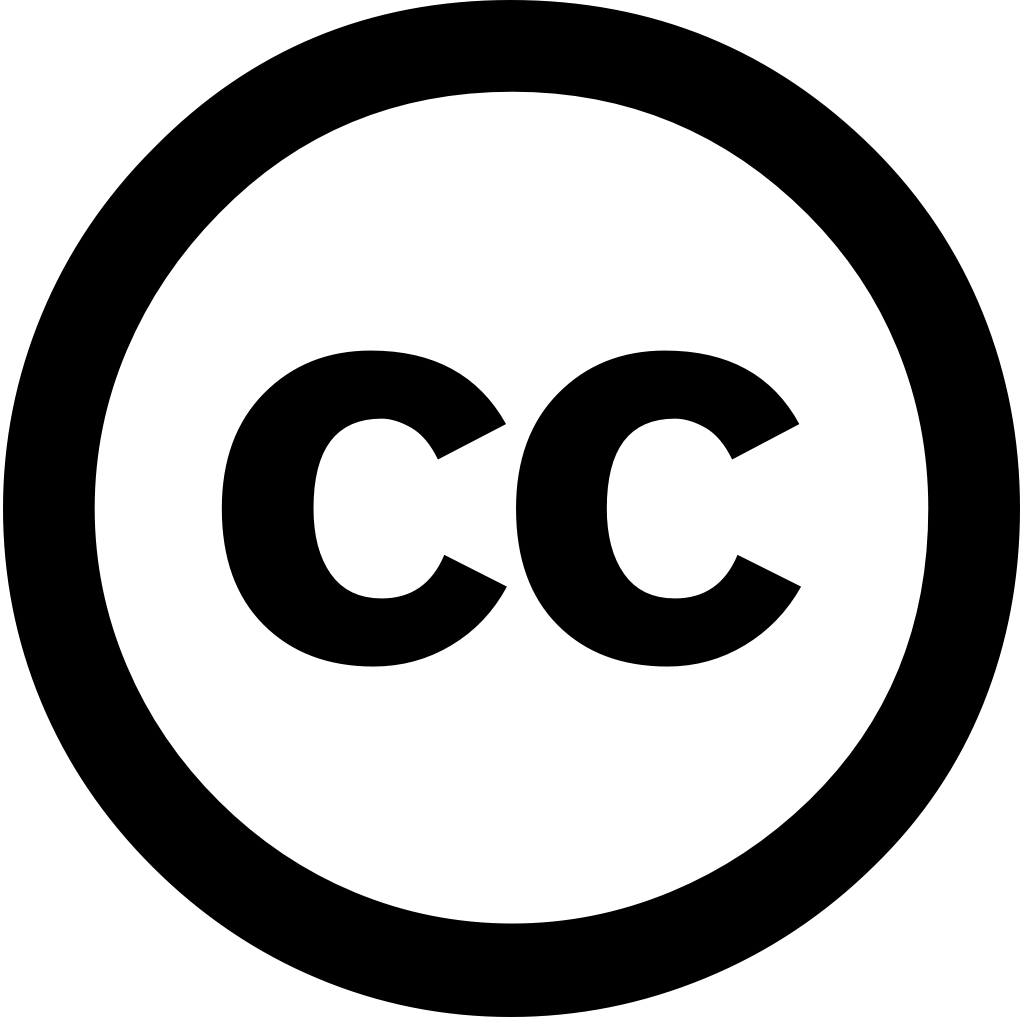
PeerJ Computer Science, Journal Year: 2024, Volume and Issue: 10, P. e2314 - e2314
Published: Sept. 18, 2024
Predicting Bitcoin prices is crucial because they reflect trends in the overall cryptocurrency market. Owing to market's short history and high price volatility, previous research has focused on factors influencing fluctuations. Although studies used sentiment analysis or diversified input features, this study's novelty lies its utilization of data classified into more than five major categories. Moreover, use spanning 2,000 days adds study. With extensive dataset, authors aimed predict across various timeframes using time series analysis. The incorporated a broad spectrum inputs, including technical indicators, from social media, news sources, Google Trends. In addition, study integrated macroeconomic on-chain transaction details, traditional financial asset data. primary objective was evaluate machine learning deep frameworks for prediction, determine optimal window sizes, enhance prediction accuracy by leveraging diverse features. Consequently, employing bidirectional long short-term memory (Bi-LSTM) yielded significant results even without excluding COVID-19 outbreak as black swan outlier. Specifically, size 3, Bi-LSTM achieved root mean squared error 0.01824, absolute 0.01213, percentage 2.97%, an R-squared value 0.98791. Additionally, ascertain importance gradient examined identify which variables specifically influenced results. Ablation test also conducted validate effectiveness validity proposed methodology provides varied examination formation, helping investors make informed decisions regarding Bitcoin-related investments, enabling policymakers legislate considering these factors.
Language: Английский