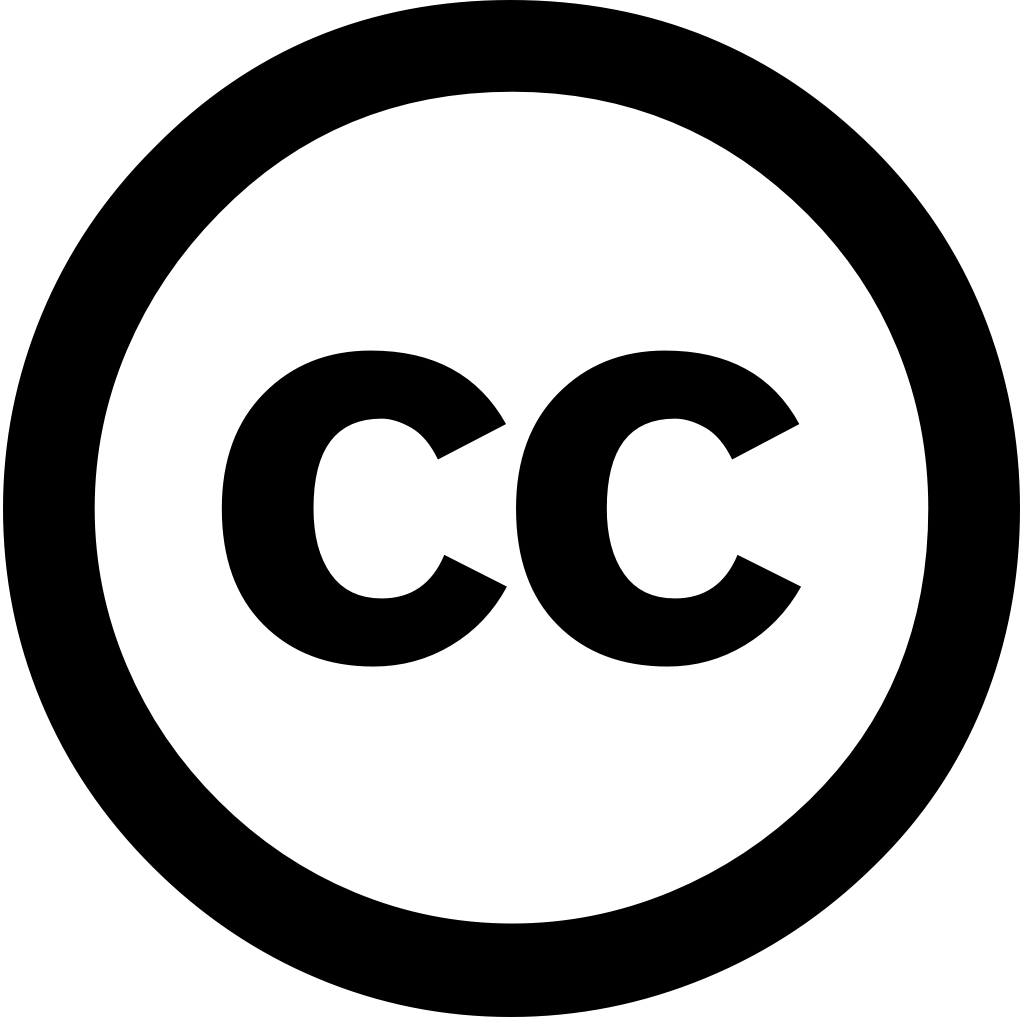
Health Science Reports, Journal Year: 2025, Volume and Issue: 8(4)
Published: April 1, 2025
ABSTRACT Background and Aims Bangladesh is confronting an unprecedented threat from dengue, worsened by climate change. Understanding its trends pinpointing climatic risk factors vital for effective intervention control. This study aimed to compare the artificial neural network (ANN) eXtreme gradient boosting (XGBoost) models identify best model predicting dengue incidence determining key in Bangladesh. Methods Monthly data were obtained Directorate General of Health Services, while collected NASA website. Exploratory analysis, as well ANN XGBoost models, performed analyze data. Results From 2000 2023, reported 565,890 cases, with 2023 having highest outbreak at 79,598 cases. Precipitation, ranging 0 mm 1012.50 (mean = 181.58 mm), showed significant variability, recorded August 2017. Relative humidity had a mean 75.99%, wind speed averaged 10.11 m/s. outperformed other achieving lowest root square error (918.83) absolute (479.44). The identified precipitation factor influencing incidence, followed relative speed. Conclusion study's findings, which incorporate predictive modeling, offer valuable insights preventing controlling epidemics early warning systems To mitigate impact future outbreaks, such precipitation, humidity, should be closely monitored, alongside proactive measures enhanced surveillance.
Language: Английский