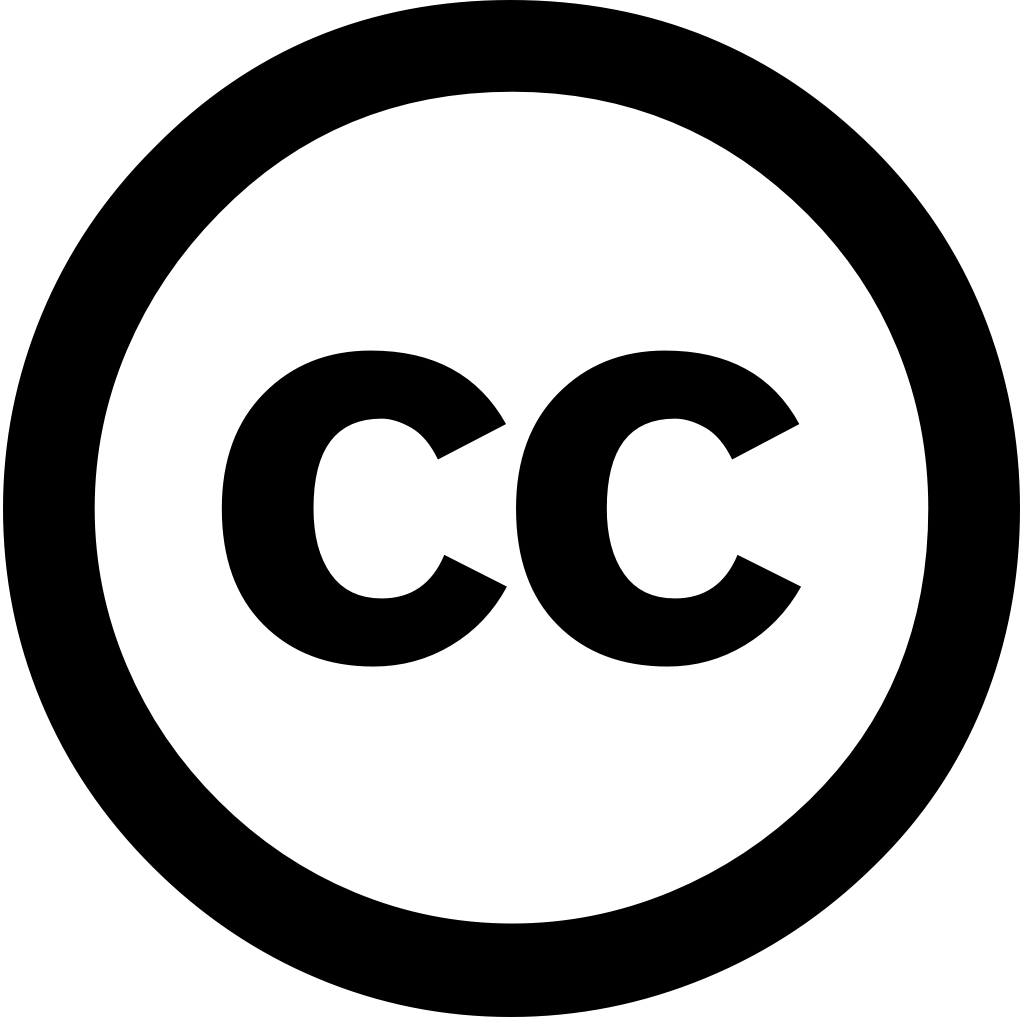
Revista Brasileira de Meteorologia, Journal Year: 2025, Volume and Issue: 40
Published: Jan. 1, 2025
Abstract The increased consumption of natural resources, such as water, has become a global concern. Consequently, determining information that can minimize water consumption, evapotranspiration, is increasingly necessary. This research evaluates the capacity Genetic Algorithms (GAs) in training and fine-tuning parameters Artificial Neural Networks (ANNs) (MLP-GA) to obtain daily values reference evapotranspiration (ETo) accordance with Penman-Monteith FAO-56 method. method employed estimate ETo at 14 weather stations Brazil. findings are assessed based on coefficient correlation (r), mean absolute error (MAE), root square (RMSE), percentage (MPE), contrasted Hargreaves-Samani, Jensen-Haise, Linacre, Benavides & Lopez, Hamon methods, along Multilayer Perceptron (MLP) neural network, which conventionally trained employs hyperparameter tuning techniques Grid Search (MLP-GRID) Random (MLP-RD). results show MLP-GA is, average, 12 times faster than MLP-RD 60 MLP-GRID, while achieving highest precision indices most regions, an r 0.99, MAE ranging from 0.11 mm 0.20 mm, RMSE between 0.14 0.27 MPE 2.49% 7.09%. These suggest generated achieve 92.91% 97.51% comparison confirms employing (GA) automate optimization model effective enhances network's predict ETo.
Language: Английский