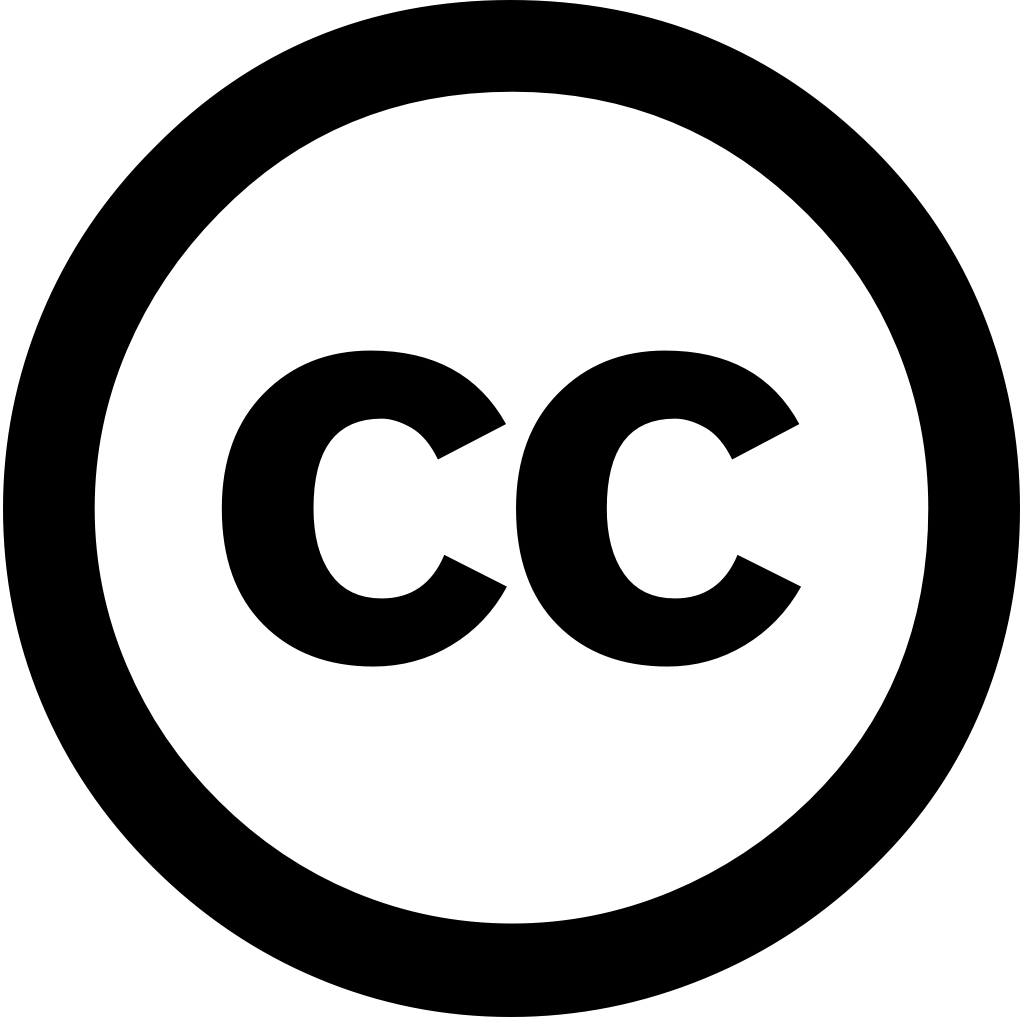
Neurospine, Journal Year: 2024, Volume and Issue: 21(3), P. 842 - 844
Published: Sept. 27, 2024
Language: Английский
Neurospine, Journal Year: 2024, Volume and Issue: 21(3), P. 842 - 844
Published: Sept. 27, 2024
Language: Английский
Global Spine Journal, Journal Year: 2025, Volume and Issue: unknown
Published: Jan. 12, 2025
Study Design Systematic review. Objective Artificial intelligence (AI) and deep learning (DL) models have recently emerged as tools to improve fracture detection, mainly through imaging modalities such computed tomography (CT) radiographs. This systematic review evaluates the diagnostic performance of AI DL in detecting cervical spine fractures assesses their potential role clinical practice. Methods A search PubMed/Medline, Embase, Scopus, Web Science was conducted for studies published between January 2000 July 2024. Studies that evaluated detection were included. Diagnostic metrics extracted included sensitivity, specificity, accuracy, area under curve. The PROBAST tool assessed bias, PRISMA criteria used study selection reporting. Results Eleven 2021 2024 demonstrated variable performance, with sensitivity ranging from 54.9% 100% specificity 72% 98.6%. Models applied CT generally outperformed those radiographs, convolutional neural networks (CNN) advanced architectures MobileNetV2 Vision Transformer (ViT) achieving highest accuracy. However, most lacked external validation, raising concerns about generalizability findings. Conclusions show significant improving particularly imaging. While these offer high further validation refinement are necessary before they can be widely integrated into should complement, rather than replace, human expertise workflows.
Language: Английский
Citations
0Asian Spine Journal, Journal Year: 2025, Volume and Issue: unknown
Published: Jan. 20, 2025
Ossification of the posterior longitudinal ligament (OPLL) is a significant spinal condition that can lead to severe neurological deficits.Recent advancements in machine learning (ML) and deep (DL) have led development promising tools for early detection diagnosis OPLL.This systematic review evaluated diagnostic performance ML DL models clinical implications OPLL detection.A was conducted following Preferred Reporting Items Systematic Reviews Meta-Analyses guidelines.PubMed/Medline Scopus databases were searched studies published between January 2000 September 2024.Eligible included those utilizing or using imaging data.All assessed risk bias appropriate tools.The key metrics, including accuracy, sensitivity, specificity, area under curve (AUC), analyzed.Eleven studies, comprising total 6,031 patients, included.The demonstrated high performance, with accuracy rates ranging from 69.6% 98.9% AUC values up 0.99.Convolutional neural networks random forest most used approaches.The overall moderate, concerns primarily related participant selection missing data.In conclusion, show great potential accurate OPLL, particularly when integrated techniques.However, ensure applicability, further research warranted validate these findings more extensive diverse populations.
Language: Английский
Citations
0Research Square (Research Square), Journal Year: 2025, Volume and Issue: unknown
Published: April 4, 2025
Language: Английский
Citations
0Neurospine, Journal Year: 2024, Volume and Issue: 21(3), P. 743 - 744
Published: Sept. 27, 2024
Language: Английский
Citations
0Neurospine, Journal Year: 2024, Volume and Issue: 21(3), P. 842 - 844
Published: Sept. 27, 2024
Language: Английский
Citations
0