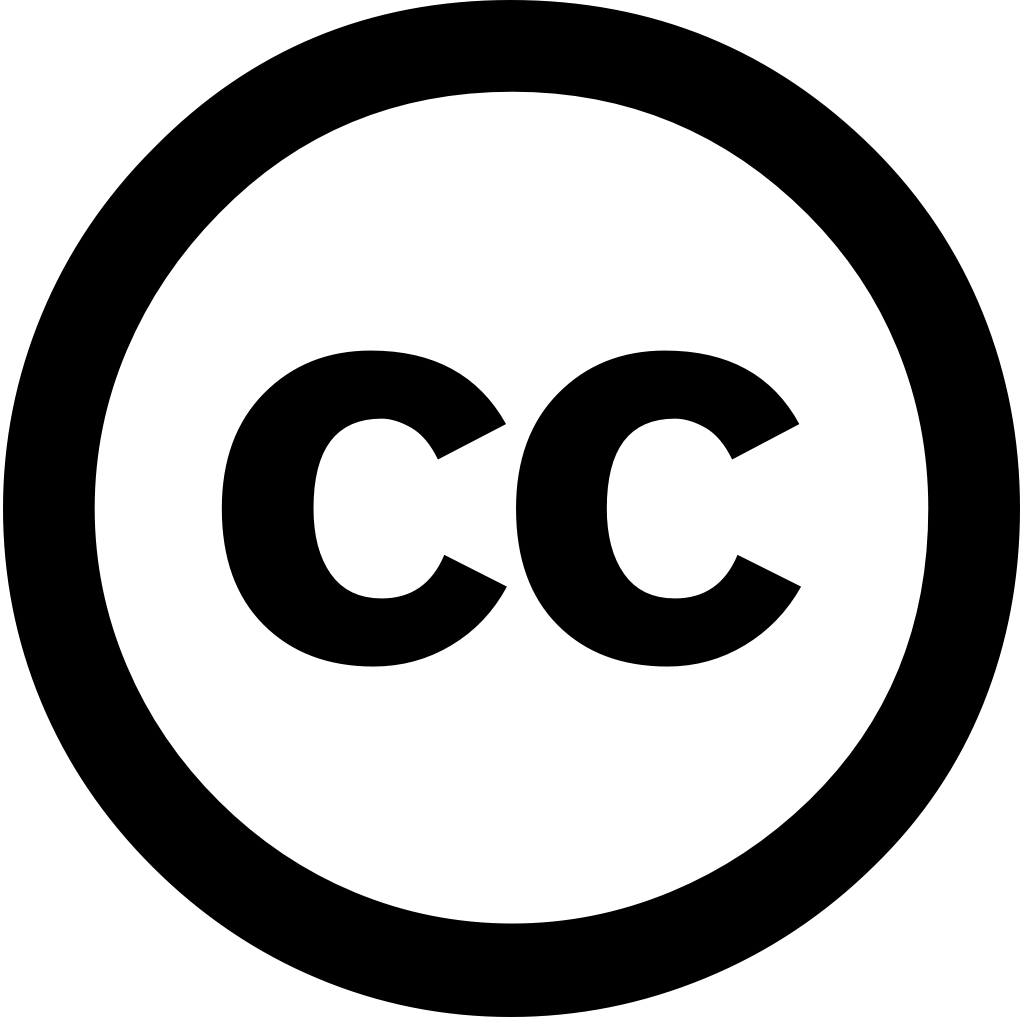
Fire, Journal Year: 2024, Volume and Issue: 7(11), P. 389 - 389
Published: Oct. 29, 2024
Fire detection is a critical task in environmental monitoring and disaster prevention, with traditional methods often limited their ability to detect fire smoke real time over large areas. The rapid identification of both indoor outdoor environments essential for minimizing damage ensuring timely intervention. In this paper, we propose novel approach by integrating vision transformer (ViT) the YOLOv5s object model. Our modified model leverages attention-based feature extraction capabilities ViTs improve accuracy, particularly complex where fires may be occluded or distributed across regions. By replacing CSPDarknet53 backbone ViT, able capture local global dependencies images, resulting more accurate under challenging conditions. We evaluate performance proposed using comprehensive Smoke Detection Dataset, which includes diverse real-world scenarios. results demonstrate that our outperforms baseline YOLOv5 variants terms precision, recall, mean average precision (mAP), achieving [email protected] 0.664 recall 0.657. ViT shows significant improvements detecting smoke, scenes backgrounds varying scales. findings suggest integration as offers promising real-time urban natural environments.
Language: Английский