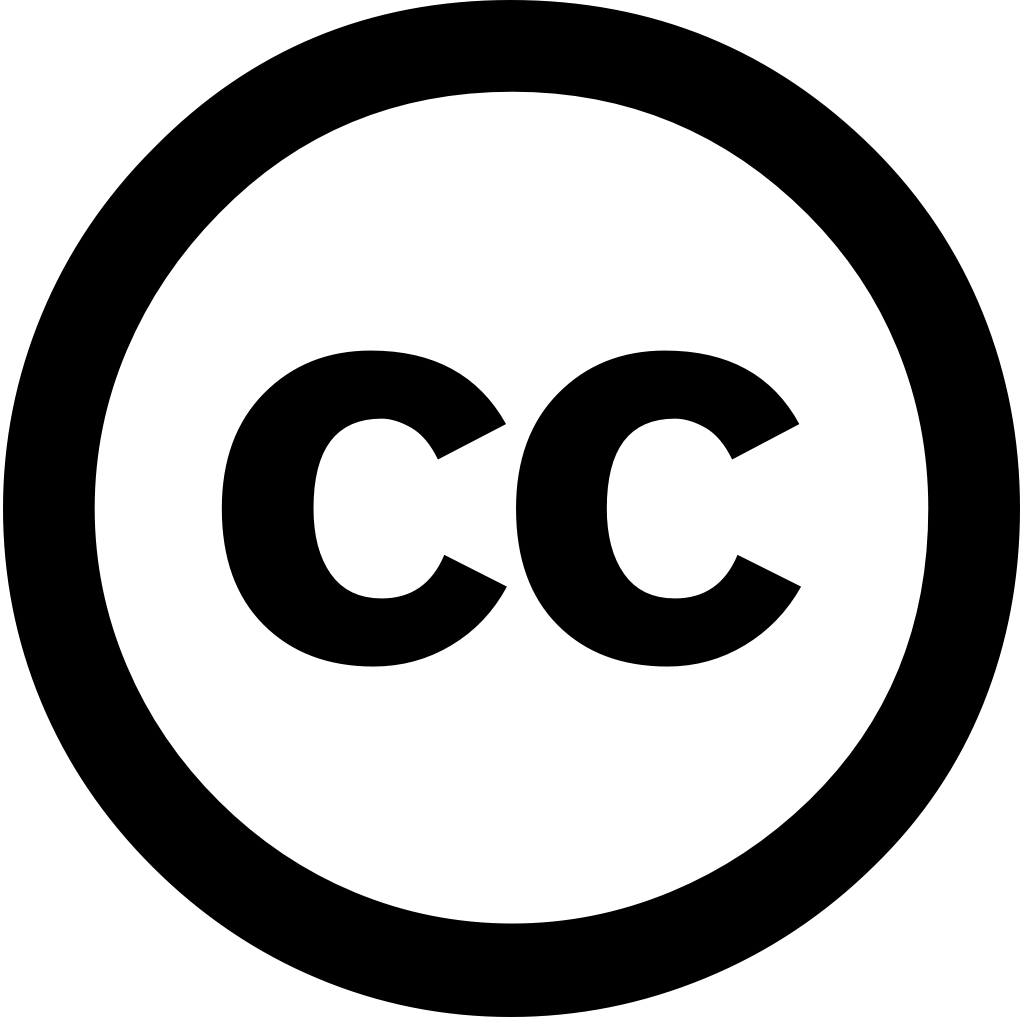
Scientific Reports, Journal Year: 2025, Volume and Issue: 15(1)
Published: March 13, 2025
The segmentation of liver margins in computed tomography (CT) images presents significant challenges due to the complex anatomical variability liver, with critical implications for medical diagnostics and treatment planning. In this study, we leverage a substantial dataset over 4,200 abdominal CT images, meticulously annotated by expert radiologists from Taleghani Hospital Kermanshah, Iran. Now made available research community, serves as rich resource enhancing validating various neural network models. We employed two advanced deep models, U-Net Detectron2, tasks. terms Mask Intersection Union (Mask IoU) metric, achieved an IoU 0.903, demonstrating high efficacy simpler cases. contrast, Detectron2 outperformed 0.974, particularly excelling accurately delineating boundaries cases where appears segmented into distinct regions within images. This highlights Detectron2's potential handling variations that pose other Our findings not only provide robust comparative analysis these models but also establish framework further enhancements imaging initiative aims just refine margin detection facilitate development automated systems diagnosing diseases, future applications extending methodologies organs, potentially transforming landscape computational healthcare.
Language: Английский