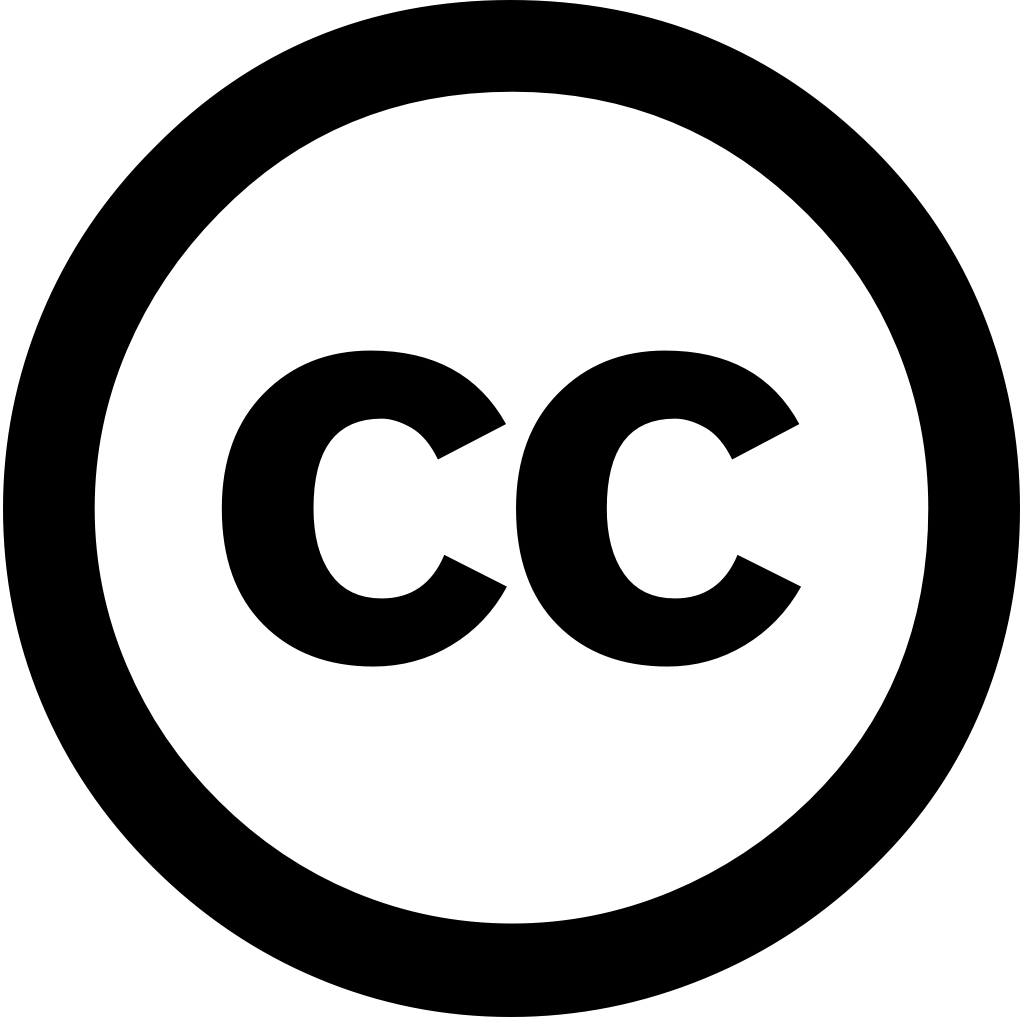
npj Computational Materials, Journal Year: 2025, Volume and Issue: 11(1)
Published: April 24, 2025
Abstract For neural network potentials (NNPs) to gain widespread use, researchers must be able trust model outputs. However, the blackbox nature of networks and their inherent stochasticity are often deterrents, especially for foundation models trained over broad swaths chemical space. Uncertainty information provided at time prediction can help reduce aversion NNPs. In this work, we detail two uncertainty quantification (UQ) methods. Readout ensembling, by finetuning readout layers an ensemble models, provides about uncertainty, while quantile regression, replacing point predictions with distributional predictions, within underlying training data. We demonstrate our approach MACE-MP-0 model, applying UQ a series finetuned models. The uncertainties produced methods demonstrated distinct measures which quality NNP output judged.
Language: Английский