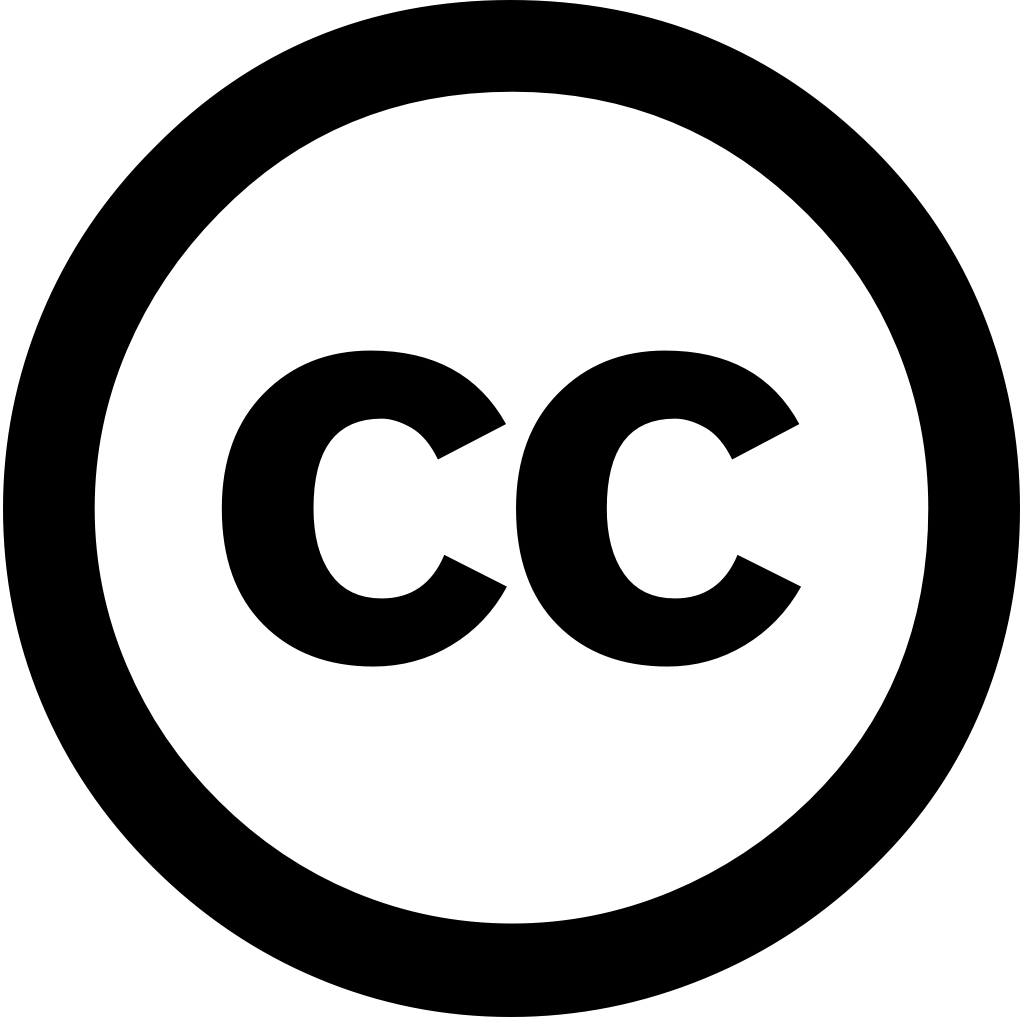
Ecological Informatics, Journal Year: 2025, Volume and Issue: unknown, P. 103087 - 103087
Published: Feb. 1, 2025
Language: Английский
Ecological Informatics, Journal Year: 2025, Volume and Issue: unknown, P. 103087 - 103087
Published: Feb. 1, 2025
Language: Английский
Published: Feb. 20, 2024
Eutrophication, a global concern, impacts water quality, ecosystems, and human health. It’s crucial to monitor algal blooms in freshwater reservoirs, as they indicate the trophic condition of waterbody through Chlorophyll-a (Chla) concentration. Traditional monitoring methods, however, are expen-sive time-consuming. Addressing this hindrance, we developed models using remotely sensed data from Sentinel-2 satellite for large-scale coverage, including its bands spectral indexes, estimate Chla concentration on 149 reservoirs Ceará, Brazil. Several machine learning were trained tested, k-nearest neighbours, random forests, extreme gradient boosting, least absolute shrinkage, group method handling (GMDH), sup-port vector models. A stepwise approach determined best subset input parameters. Using 70/30 split training testing datasets, best-performing model was GMDH, achieving an R2 0.91, MAPE 102.34%, RMSE 20.38 g/L, which values consistent with ones found literature. Nevertheless, predicted most sensitive red, green, near infra-red bands.
Language: Английский
Citations
4Energies, Journal Year: 2024, Volume and Issue: 17(14), P. 3580 - 3580
Published: July 21, 2024
Merging machine learning with the power of quantum computing holds great potential for data-driven decision making and development powerful models complex datasets. This area offers improving accuracy real-time prediction renewable energy production, such as solar irradiance forecasting. However, literature on this topic is sparse. Addressing knowledge gap, study aims to develop evaluate a neural network model up 3 h in advance. The proposed was compared Support Vector Regression, Group Method Data Handling, Extreme Gradient Boost classical models. framework could provide competitive results its competitors, considering forecasting intervals 5 120 min ahead, where it fourth best-performing paradigm. For ahead predictions, achieved second-best other approaches, reaching root mean squared error 77.55 W/m2 coefficient determination 80.92% global horizontal longer horizons suggest that may process spatiotemporal information from input dataset manner not attainable by current thus capacity predictive windows.
Language: Английский
Citations
3The Science of The Total Environment, Journal Year: 2024, Volume and Issue: 957, P. 177180 - 177180
Published: Nov. 23, 2024
Language: Английский
Citations
3Ecological Informatics, Journal Year: 2025, Volume and Issue: unknown, P. 103087 - 103087
Published: Feb. 1, 2025
Language: Английский
Citations
0