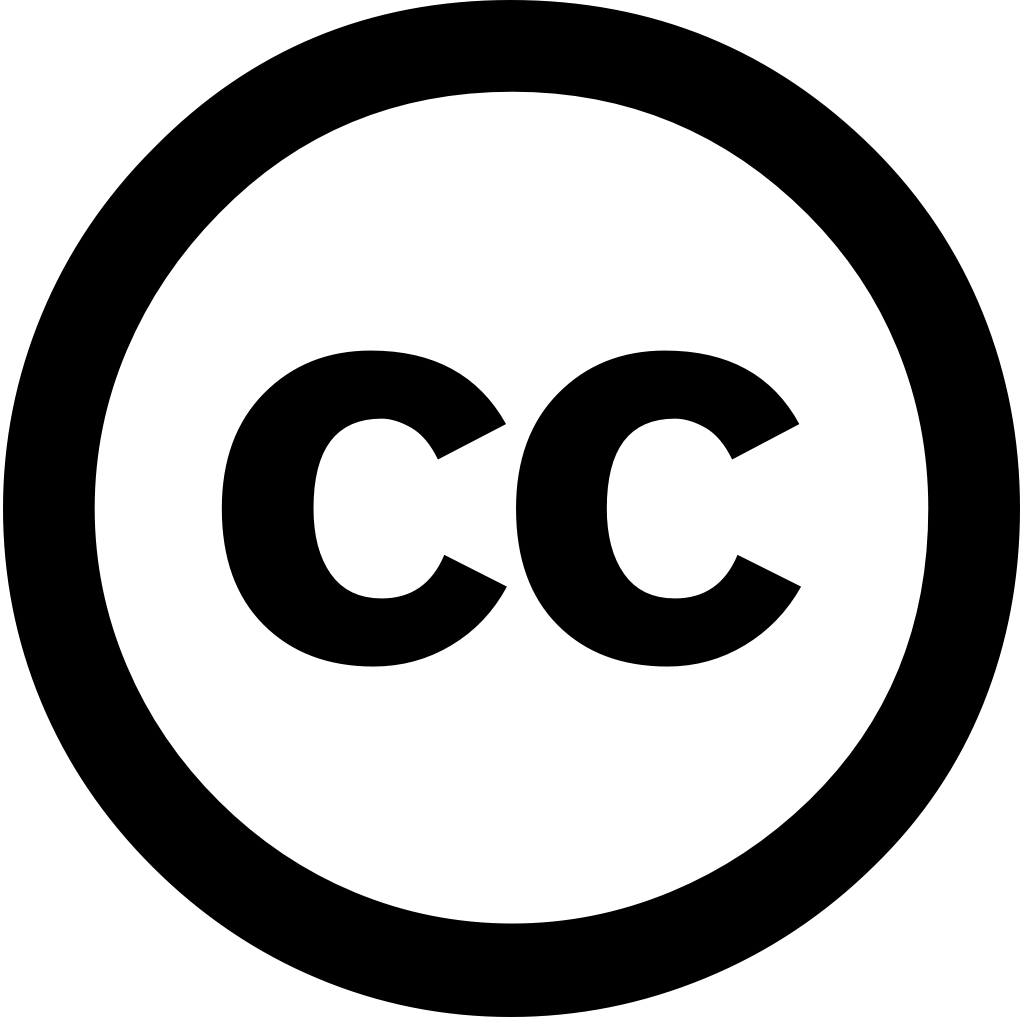
Research Square (Research Square), Journal Year: 2024, Volume and Issue: unknown
Published: July 4, 2024
Language: Английский
Research Square (Research Square), Journal Year: 2024, Volume and Issue: unknown
Published: July 4, 2024
Language: Английский
Published: May 25, 2024
The application of knowledge distillation to reduce hallucination in large language models represents a novel and significant advancement enhancing the reliability accuracy AI-generated content. research presented demonstrates efficacy transferring from high-capacity teacher model more compact student model, leading substantial improvements exact match notable reductions rates. methodology involved use temperature scaling, intermediate layer matching, comprehensive evaluation using MMLU benchmark, which assessed model’s performance across diverse set tasks. Experimental results indicated that distilled outperformed baseline generating accurate contextually appropriate responses while maintaining computational efficiency. findings underscore potential as scalable solution for improving robustness models, making them applicable real-world scenarios demand high factual accuracy. Future directions include exploring multilingual multi-modal distillation, integrating reinforcement learning, developing refined metrics further enhance performance.
Language: Английский
Citations
20Published: June 6, 2024
The increasing use of AI-generated content has highlighted the critical issue hallucinations, where models produce factually incorrect or misleading outputs. Addressing this challenge, a novel approach dynamically supplements federated search engine results in real-time to significantly reduce hallucinations and enhance response accuracy. methodology involves integrating data from multiple engines into responses generated by Mistral Large model, thereby providing more accurate contextually appropriate output. Comprehensive evaluation using Microsoft PromptBench dataset demonstrates substantial improvements accuracy, relevance, reduction hallucinations. Quantitative performance metrics, statistical analysis, detailed case studies confirm effectiveness dynamic supplementation approach. findings suggest significant implications for developing reliable AI applications across various domains, emphasizing potential hybrid systems that combine strengths large language information retrieval. Future research directions include refining triggering mechanisms, expanding sources, optimizing process further scalability.
Language: Английский
Citations
12Research Square (Research Square), Journal Year: 2024, Volume and Issue: unknown
Published: June 7, 2024
Language: Английский
Citations
10Published: Aug. 6, 2024
LLMs have demonstrated strong capabilities in generating human-like text and understanding complex linguistic patterns; however, they are prone to plausiblesounding information that is factually incorrect, known as hallucinations, which poses a significant challenge for applications requiring high accuracy reliability. The proposed methodologies, Sliding Generation Self-Checks, introduce novel techniques mitigate hallucinations through structured segmentation, iterative refinement, multi-step verification processes, enhancing the factual consistency of LLM outputs. technique improves contextual relevance by dividing input prompts into overlapping segments aggregating responses, while Self-Checks mechanism ensures internal rephrasing posing related questions, thereby reducing erroneous Comprehensive evaluations efficacy these integrated approaches, highlighting marked improvements reliability across various domains, emphasizing their potential deployment high-stakes environments where integrity crucial. This research contributes advancement AI technology, providing robust framework developing more trustworthy effective capable handling sensitive tasks.
Language: Английский
Citations
4Research Square (Research Square), Journal Year: 2024, Volume and Issue: unknown
Published: June 11, 2024
Language: Английский
Citations
3Research Square (Research Square), Journal Year: 2024, Volume and Issue: unknown
Published: July 4, 2024
Language: Английский
Citations
2