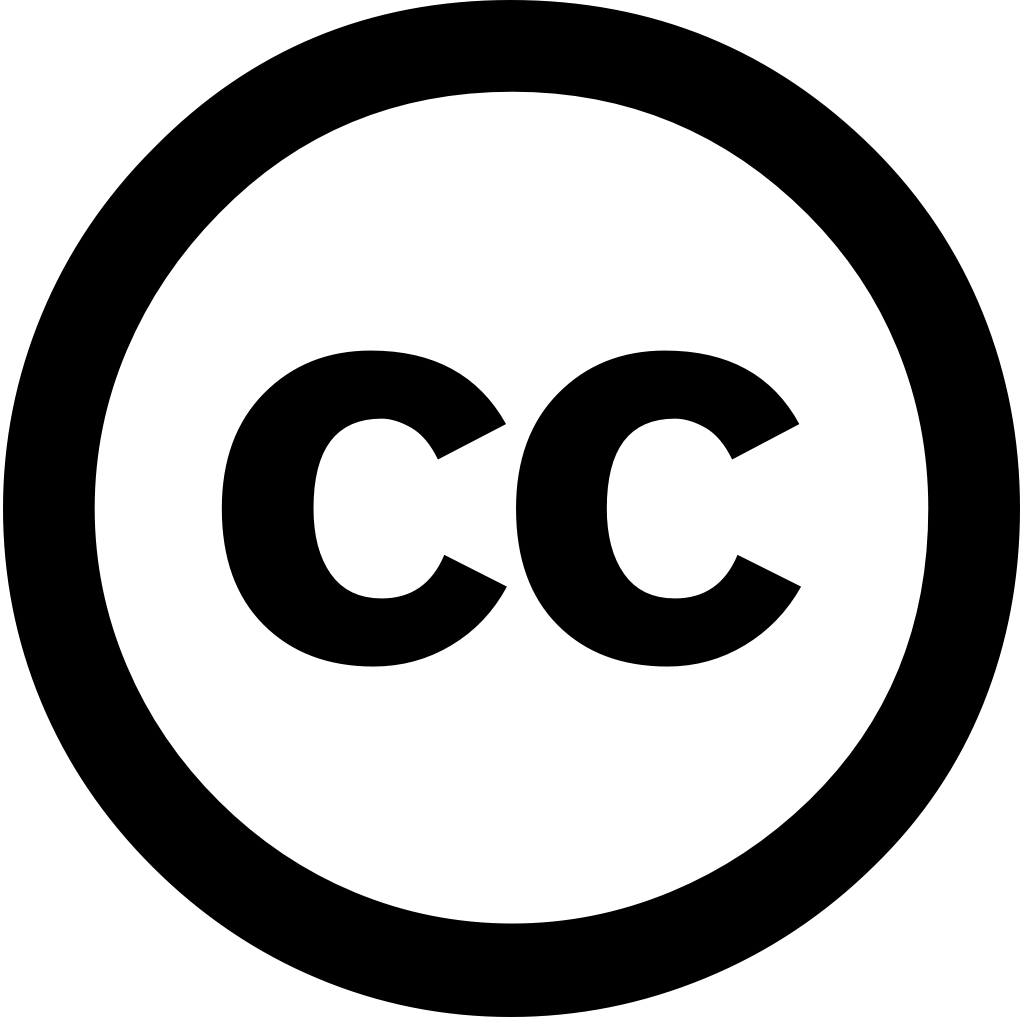
E3S Web of Conferences, Journal Year: 2023, Volume and Issue: 466, P. 01015 - 01015
Published: Jan. 1, 2023
Due to the strong volatility of PV power, grid-connected may have an impact on safe and stable operation power system, so accurate prediction is great significance maintenance system. In order improve accuracy photovoltaic ultra-short-term method was studied by combining Aquila Optimizer (AO) algorithm, Variational Mode Decomposition (VMD), Random Forest (RF) Informer model. Firstly, VMD parameters are optimized AO reduce adverse effects human-set accuracy; used decompose original series into multiple sub-sequences complexity series; then, RF feature selection screen out meteorological features relevance for each sub-sequence further dimensions model runtime ensure effectiveness input features. Finally, deeply mine potential time subsequence prediction, predicted values superimposed reconstructed obtain final results. The simulation results show that in this paper has high accuracy, compared with Informer, MAE reduced 49.14% RMSE 47.64%.
Language: Английский