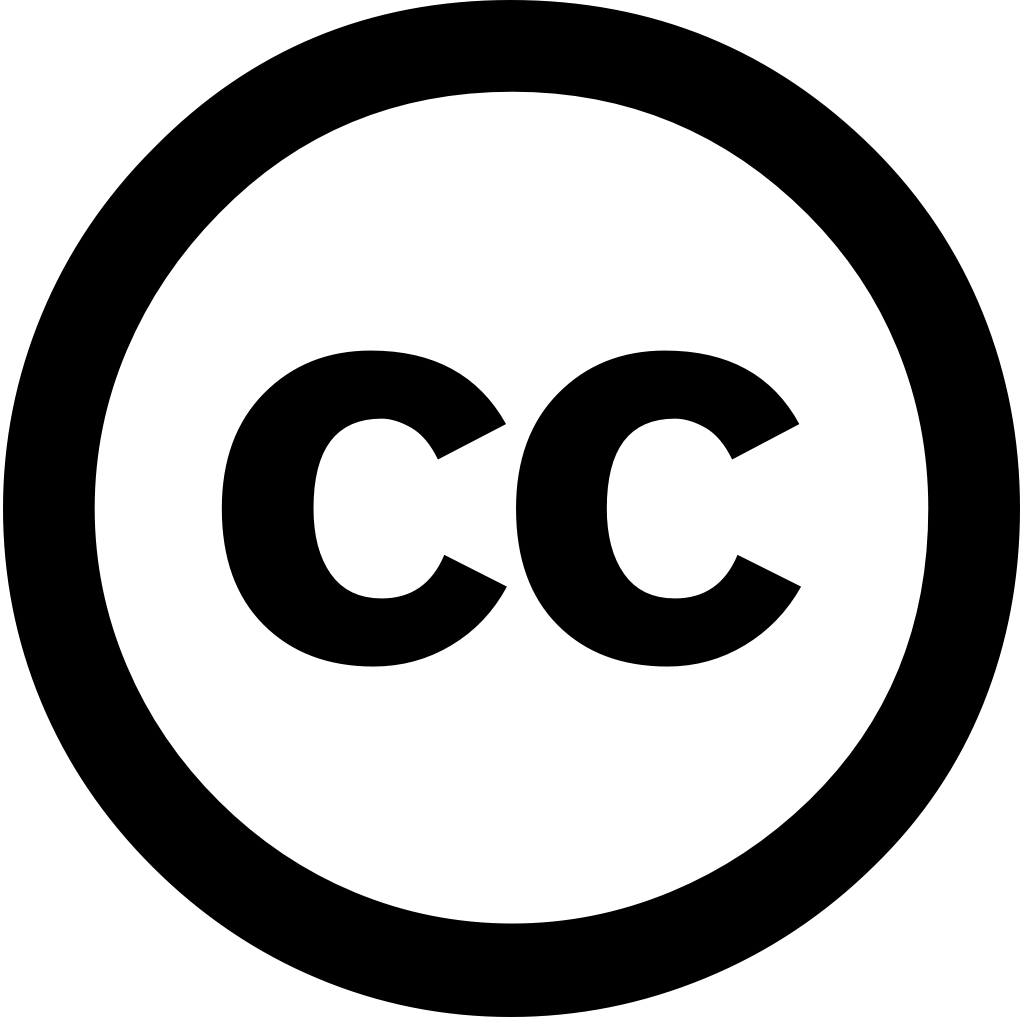
Ecological Informatics, Journal Year: 2024, Volume and Issue: 82, P. 102697 - 102697
Published: June 21, 2024
Soil heat flux (SHF) is a key component of the surface energy balance and driver soil physiochemical biological processes. Accurate estimation challenging due to variations in composition, overlying vegetation density phenology, highly variable environmental forcings. Existing SHF process-based data-driven methods have focused on midday landscape scale estimates that correspond satellite acquisitions, despite high variability displays at diurnal scales throughout growing season. Recently developed techniques emphasized pre-determined predictor variables, with model complexity selection not carefully evaluated, leaving gap our understanding what information required accurately predict SHF. Here we evaluated suite ensemble machine learning models quantify ability meteorological remote sensing data half-hourly temporal resolution season, producing comprehensive evaluation importance set composition for resolution. We compared this six semi-empirical found broadly outperformed existing capturing across season four agro-ecosystems (soybean, corn, sorghum, miscanthus). Crop-specific ML were able capture over 86% using only two pointing need careful sets identify synergistic combinations. pooled all crops captured almost 80% three predictors, demonstrating power generalizability these independent crop type. Shapley additive explanations (SHAP) used examine interpretability, providing insights into typically opaque modelling process interaction variables. Models trained fewer input variables tended display more linear interpretable feature attribution, motivating use interpretability as an important consideration parsimonious selection. These results provide robust demonstration sub-hourly seasons spanning wide range phenological variation unique agricultural systems. This study provides requirements performance, guiding future applications will take advantage next generation satellite-based observing systems, or in-situ proximal observations status conditions.
Language: Английский