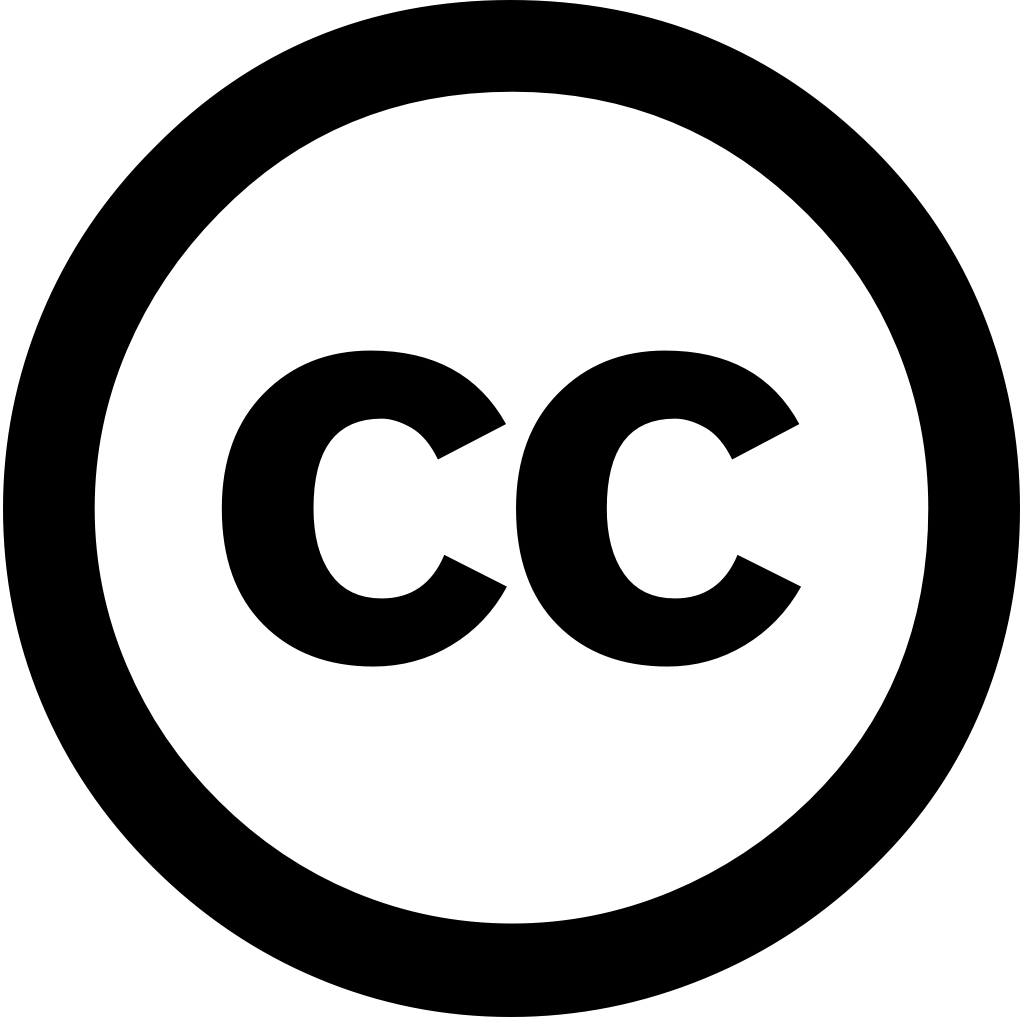
Applied Sciences, Journal Year: 2025, Volume and Issue: 15(8), P. 4471 - 4471
Published: April 18, 2025
The growing volume and complexity of gene expression data necessitate biologically meaningful statistically robust methods for feature selection to enhance the effectiveness disease diagnosis systems. present study addresses this challenge by proposing a pipeline that integrates RNA-seq preprocessing, differential analysis, Gene Ontology (GO) enrichment, ensemble-based machine learning. employs non-parametric Kruskal–Wallis test identify differentially expressed genes, followed dual enrichment analysis using both Fisher’s exact Kolmogorov–Smirnov across three GO categories: Biological Process (BP), Molecular Function (MF), Cellular Component (CC). Genes associated with terms found significant tests were used construct multiple subsets, including subsets based on individual categories, their union, intersection. Classification experiments random forest model, validated via 5-fold cross-validation, demonstrated derived from CC category union all categories achieved highest accuracy weighted F1-scores, exceeding 0.97 14 cancer types. In contrast, BP, MF, especially intersection exhibited lower performance. These results confirm discriminative power spatially localized annotations underscore value integrating statistical functional information into selection. proposed approach improves reliability biomarker discovery supports downstream analyses such as clustering biclustering, providing strong foundation developing precise diagnostic tools in personalized medicine.
Language: Английский