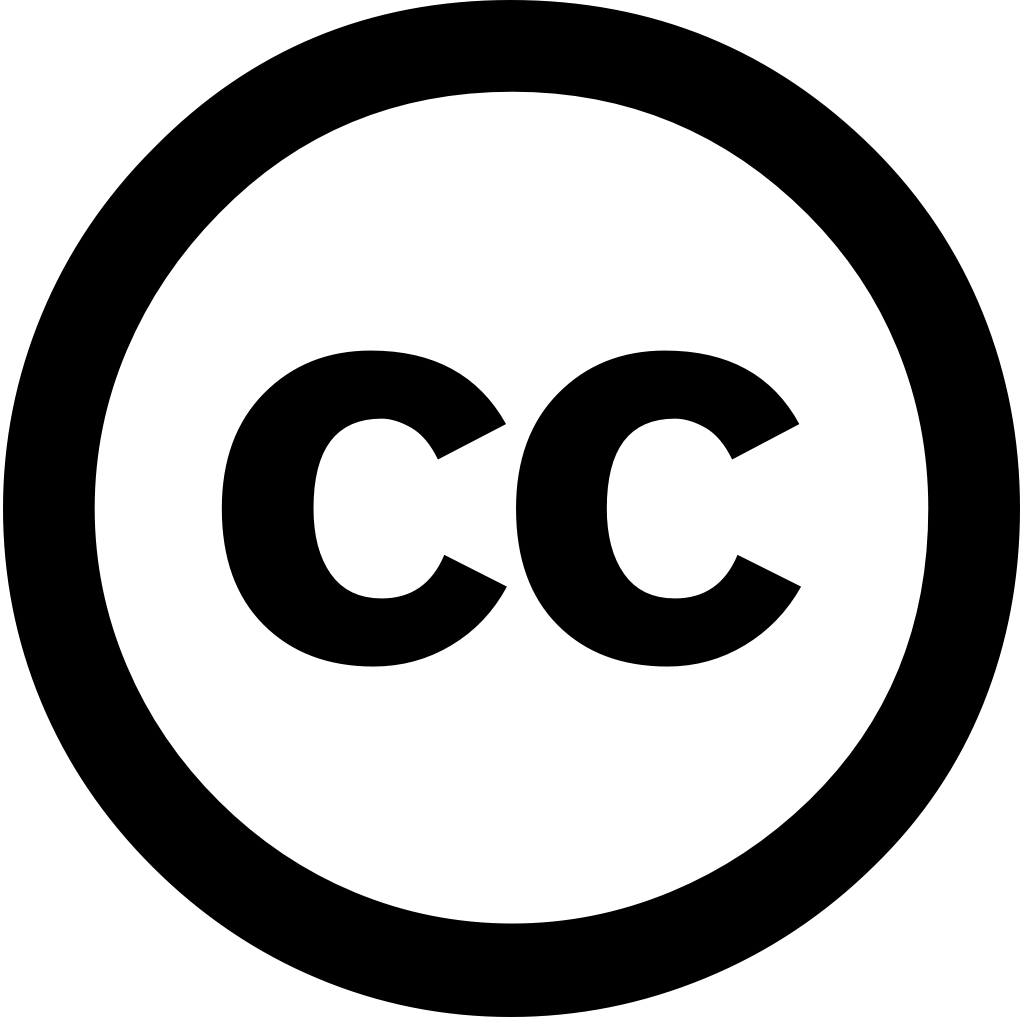
Atmosphere, Journal Year: 2024, Volume and Issue: 15(12), P. 1461 - 1461
Published: Dec. 7, 2024
The inherent uncertainties in traditional hydrological models present significant challenges for accurately simulating runoff. Combining machine learning with is an essential approach to enhancing the runoff modeling capabilities of models. However, research on impact mixed simulation capability limited. Therefore, this study uses model Simplified Daily Hydrological Model (SIMHYD) and Long Short Term Memory (LSTM) construct two coupled models: a direct coupling dynamically improved predictive validity hybrid model. These were evaluated using US CAMELS dataset assess combination methods capabilities. results indicate that both compared individual models, combined forecasting dynamic prediction effectiveness (DPE) demonstrating optimal capability. Compared LSTM, showed median increase 12.8% Nash Sutcliffe efficiency (NSE) daily during validation period, 12.5% SIMHYD. In addition, LSTM model, high flow increased by 23.6%, SIMHYD, it 28.4%. At same time, stability low was significantly improved. performance testing involving varying training period lengths, DPE trained 12 years exhibited best performance, showing 3.5% 1.5% NSE periods 6 18 years, respectively.
Language: Английский