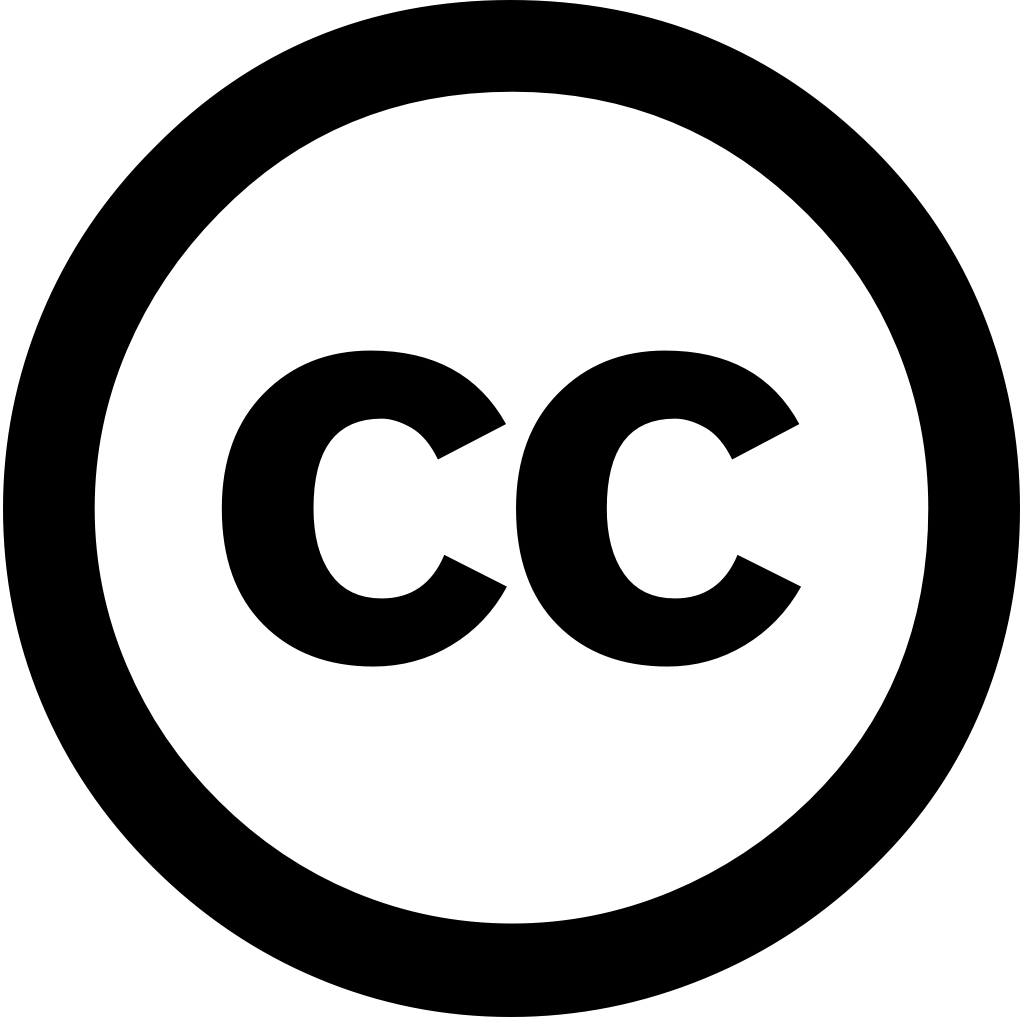
Atmosphere, Journal Year: 2025, Volume and Issue: 16(4), P. 398 - 398
Published: March 30, 2025
Solar radiation is one of the most abundant energy sources in world and a crucial parameter that must be researched developed for sustainable projects future generations. This study evaluates performance different machine learning methods solar prediction Konya, Turkey, region with high potential. The analysis based on hydro-meteorological data collected from NASA/POWER, covering period 1 January 1984 to 31 December 2022. compares Long Short-Term Memory (LSTM), Bidirectional LSTM (Bi-LSTM), Gated Recurrent Unit (GRU), GRU (Bi-GRU), LSBoost, XGBoost, Bagging, Random Forest (RF), General Regression Neural Network (GRNN), Support Vector Machines (SVM), Artificial Networks (MLANN, RBANN). variables used include temperature, relative humidity, precipitation, wind speed, while target variable radiation. dataset was divided into 75% training 25% testing. Performance evaluations were conducted using Mean Absolute Error (MAE), Root Square (RMSE), coefficient determination (R2). results indicate Bi-LSTM models performed best test phase, demonstrating superiority deep learning-based approaches prediction.
Language: Английский