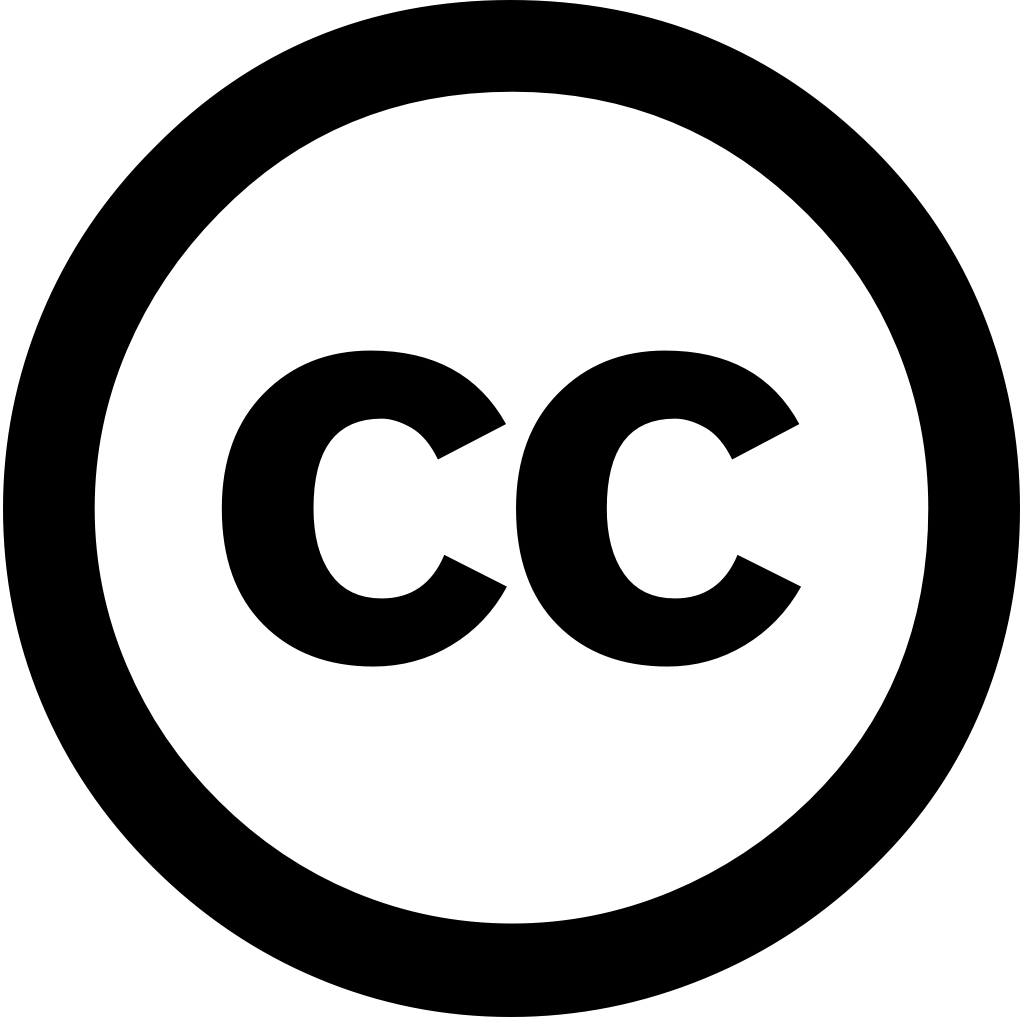
Watershed Ecology and the Environment, Journal Year: 2025, Volume and Issue: unknown
Published: May 1, 2025
Language: Английский
Watershed Ecology and the Environment, Journal Year: 2025, Volume and Issue: unknown
Published: May 1, 2025
Language: Английский
Journal of Hydrology, Journal Year: 2024, Volume and Issue: 641, P. 131767 - 131767
Published: Aug. 3, 2024
Language: Английский
Citations
7Water Research, Journal Year: 2024, Volume and Issue: 268, P. 122779 - 122779
Published: Nov. 9, 2024
Language: Английский
Citations
5Geothermics, Journal Year: 2025, Volume and Issue: 130, P. 103323 - 103323
Published: March 16, 2025
Language: Английский
Citations
0Biology, Journal Year: 2025, Volume and Issue: 14(5), P. 520 - 520
Published: May 8, 2025
Freshwater ecosystems are increasingly threatened by climate change and anthropogenic activities, necessitating innovative scalable monitoring solutions. Artificial intelligence (AI) has emerged as a transformative tool in aquatic biodiversity research, enabling automated species identification, predictive habitat modeling, conservation planning. This systematic review follows the PRISMA framework to analyze AI applications freshwater studies. Using structured literature search across Scopus, Web of Science, Google Scholar, we identified 312 relevant studies published between 2010 2024. categorizes into assessment, ecological risk evaluation, strategies. A bias assessment was conducted using QUADAS-2 RoB 2 frameworks, highlighting methodological challenges, such measurement inconsistencies model validation. The citation trends demonstrate exponential growth AI-driven with leading contributions from China, United States, India. Despite growing use this field, also reveals several persistent including limited data availability, regional imbalances, concerns related generalizability transparency. Our findings underscore AI’s potential revolutionizing but emphasize need for standardized methodologies, improved integration, interdisciplinary collaboration enhance insights efforts.
Language: Английский
Citations
0Watershed Ecology and the Environment, Journal Year: 2025, Volume and Issue: unknown
Published: May 1, 2025
Language: Английский
Citations
0