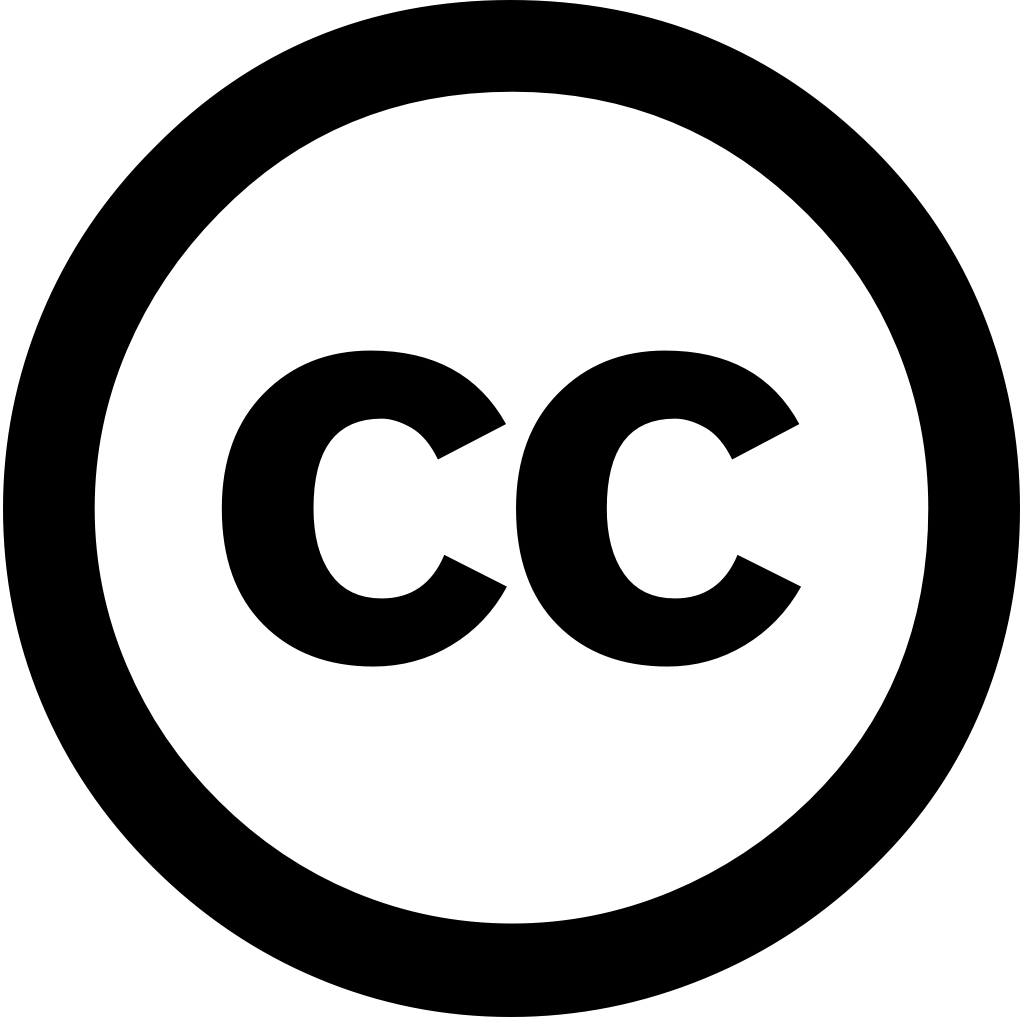
Published: Oct. 19, 2024
Language: Английский
Published: Oct. 19, 2024
Language: Английский
Cancer Medicine, Journal Year: 2025, Volume and Issue: 14(1)
Published: Jan. 1, 2025
ABSTRACT Purpose Caregivers in pediatric oncology need accurate and understandable information about their child's condition, treatment, side effects. This study assesses the performance of publicly accessible large language model (LLM)‐supported tools providing valuable reliable to caregivers children with cancer. Methods In this cross‐sectional study, we evaluated four LLM‐supported tools—ChatGPT (GPT‐4), Google Bard (Gemini Pro), Microsoft Bing Chat, SGE—against a set frequently asked questions (FAQs) derived from Children's Oncology Group Family Handbook expert input (In total, 26 FAQs 104 generated responses). Five experts assessed LLM responses using measures including accuracy, clarity, inclusivity, completeness, clinical utility, overall rating. Additionally, content quality was readability, AI disclosure, source credibility, resource matching, originality. We used descriptive analysis statistical tests Shapiro–Wilk, Levene's, Kruskal–Wallis H ‐tests, Dunn's post hoc for pairwise comparisons. Results ChatGPT shows high when by experts. also performed well, especially accuracy clarity responses, whereas Chat SGE had lower scores. Regarding disclosure being AI, it observed less which may have affected maintained balance between response clarity. most readable answered complexity. varied significantly ( p < 0.001) across all evaluations except inclusivity. Through our thematic free‐text comments, emotional tone empathy emerged as unique theme mixed feedback on expectations be empathetic. Conclusion can enhance caregivers' knowledge oncology. Each has strengths areas improvement, indicating careful selection based specific contexts. Further research is required explore application other medical specialties patient demographics, assessing broader applicability long‐term impacts.
Language: Английский
Citations
3Journal of Multidisciplinary Healthcare, Journal Year: 2025, Volume and Issue: Volume 18, P. 223 - 238
Published: Jan. 1, 2025
The integration of large language models (LLMs) in healthcare has generated significant interest due to their potential improve diagnostic accuracy, personalization treatment, and patient care efficiency. This study aims conduct a comprehensive bibliometric analysis identify current research trends, main themes future directions regarding applications the sector. A systematic scan publications until 08.05.2024 was carried out from an important database such as Web Science.Using tools VOSviewer CiteSpace, we analyzed data covering publication counts, citation analysis, co-authorship, co- occurrence keywords thematic development map intellectual landscape collaborative networks this field. included more than 500 articles published between 2021 2024. United States, Germany Kingdom were top contributors highlights that neural network imaging, natural processing for clinical documentation, field general internal medicine, radiology, medical informatics, health services, surgery, oncology, ophthalmology, neurology, orthopedics psychiatry have seen growth over past two years. Keyword trend revealed emerging sub-themes research, artificial intelligence, ChatGPT, education, processing, management, virtual reality, chatbot, indicating shift towards addressing broader implications LLM application healthcare. use is expanding with academic interest. not only maps state but also identifies areas require further development. Continued advances are expected significantly impact applications, focus on increasing accuracy through advanced analytics.
Language: Английский
Citations
2AI, Journal Year: 2025, Volume and Issue: 6(1), P. 13 - 13
Published: Jan. 16, 2025
This study aimed to evaluate the potential of Large Language Models (LLMs) in healthcare diagnostics, specifically their ability analyze symptom-based prompts and provide accurate diagnoses. The focused on models including GPT-4, GPT-4o, Gemini, o1 Preview, GPT-3.5, assessing performance identifying illnesses based solely provided symptoms. Symptom-based were curated from reputable medical sources ensure validity relevance. Each model was tested under controlled conditions diagnostic accuracy, precision, recall, decision-making capabilities. Specific scenarios designed explore both general high-stakes tasks. Among models, GPT-4 achieved highest demonstrating strong alignment with reasoning. Gemini excelled requiring precise decision-making. GPT-4o Preview showed balanced performance, effectively handling real-time tasks a focus precision recall. though less advanced, proved dependable for highlights strengths limitations LLMs diagnostics. While such as exhibit promise, challenges privacy compliance, ethical considerations, mitigation inherent biases must be addressed. findings suggest pathways responsibly integrating into processes enhance outcomes.
Language: Английский
Citations
2Global Spine Journal, Journal Year: 2025, Volume and Issue: unknown
Published: Feb. 17, 2025
Study Design Comparative Analysis. Objectives The American College of Surgeons developed the 2022 Best Practice Guidelines to provide evidence-based recommendations for managing spinal injuries. This study aims assess concordance ChatGPT-4o and Gemini Advanced with ACS Guidelines, offering first expert evaluation these models in cord Methods Trauma Quality Program Practices Spine Injury were used create 52 questions based on key clinical recommendations. These grouped into informational (8), diagnostic (14), treatment (30) categories posed Google Advanced. Responses graded guidelines validated by a board-certified spine surgeon. Results ChatGPT was concordant 38 (73.07%) 36 (69.23%). Most non-concordant answers due insufficient information. disagreed 8 questions, 5 3. Both achieved 75% information; outperformed diagnostics (78.57% vs 71.43%), while had higher (73.33% 63.33%). Conclusions demonstrate potential as valuable assets injury management providing responses aligned current best practices. marginal differences rates suggest that neither model exhibits superior ability deliver guidelines. Despite LLMs increasing sophistication utility, existing limitations currently prevent them from being clinically safe practical trauma-based settings.
Language: Английский
Citations
2BMC Medical Informatics and Decision Making, Journal Year: 2024, Volume and Issue: 24(1)
Published: Sept. 9, 2024
Language: Английский
Citations
10International Journal of Rheumatic Diseases, Journal Year: 2025, Volume and Issue: 28(1)
Published: Jan. 1, 2025
Language: Английский
Citations
1Published: Jan. 25, 2025
This study reports a comprehensive environmental scan of the generative AI (GenAI) infrastructure in national network for clinical and translational science across 36 institutions supported by CTSA Program led National Center Advancing Translational Sciences (NCATS) Institutes Health (NIH) at United States. Key findings indicate diverse range institutional strategies, with most organizations experimental phase GenAI deployment. The results underscore need more coordinated approach to governance, emphasizing collaboration among senior leaders, clinicians, information technology staff, researchers. Our analysis reveals that 53% identified data security as primary concern, followed lack clinician trust (50%) bias (44%), which must be addressed ensure ethical effective implementation technologies.
Language: Английский
Citations
1JMIR Medical Informatics, Journal Year: 2024, Volume and Issue: 12, P. e58478 - e58478
Published: Sept. 5, 2024
Abstract With the popularization of large language models (LLMs), strategies for their effective and safe usage in health care research have become increasingly pertinent. Despite growing interest eagerness among professionals scientists to exploit potential LLMs, initial attempts may yield suboptimal results due a lack user experience, thus complicating integration artificial intelligence (AI) tools into workplace routine. Focusing on with limited LLM this viewpoint article highlights discusses 6 easy-to-implement use cases practical relevance. These encompass customizing translations, refining text extracting information, generating comprehensive overviews specialized insights, compiling ideas cohesive narratives, crafting personalized educational materials, facilitating intellectual sparring. Additionally, we discuss general prompting precautions implementation AI biomedicine. various hurdles challenges, LLMs daily routines physicians researchers promises heightened productivity efficiency.
Language: Английский
Citations
5Journal of Educational Evaluation for Health Professions, Journal Year: 2025, Volume and Issue: 22, P. 4 - 4
Published: Jan. 16, 2025
The peer review process ensures the integrity of scientific research. This is particularly important in medical field, where research findings directly impact patient care. However, rapid growth publications has strained reviewers, causing delays and potential declines quality. Generative artificial intelligence, especially large language models (LLMs) such as ChatGPT, may assist researchers with efficient, high-quality reviews. explores integration LLMs into review, highlighting their strengths linguistic tasks challenges assessing validity, clinical medicine. Key points for include initial screening, reviewer matching, feedback support, review. implementing these purposes will necessitate addressing biases, privacy concerns, data confidentiality. We recommend using complementary tools under clear guidelines to not replace, human expertise maintaining rigorous standards.
Language: Английский
Citations
0Artificial Intelligence Surgery, Journal Year: 2025, Volume and Issue: 5(1), P. 116 - 25
Published: Feb. 20, 2025
Aim: Large language models (LLMs) like ChatGPT have transformed access to health information. For transgender individuals considering gender-affirming surgery (GAS), accurate and reliable information is essential for informed decision making. This study aimed quantitatively assess the use of among GAS its impact on their decision-making process. Methods: A cross-sectional survey was conducted in January 2024 Prolific. Participants included English-speaking U.S. users over 18 whose current gender differed from assigned at birth. The collected demographic information, evaluated interest GAS, examined interactions with ChatGPT. Descriptive statistics were used analysis. Results: 207 participants (average age 30.2 years), primarily identifying as non-binary (40.6%), men (29.5%), women (13%). Most expressed (89%). Primary sources online forums (24.6%), medical websites (21.3%), social media (17.4%). While many had (73%), few utilized it (6.7%). Among those who did, majority (70%) rated usefulness moderate slight, some reporting a positive influence making (40%). Trust ChatGPT’s highly by 80% participants. Conclusions: In our cohort, less commonly than websites. suggests that patients prefer platforms offer visual content, human interaction, relatability. These findings highlight importance guiding toward sources, such healthcare providers, reputable websites, academic literature, support
Language: Английский
Citations
0