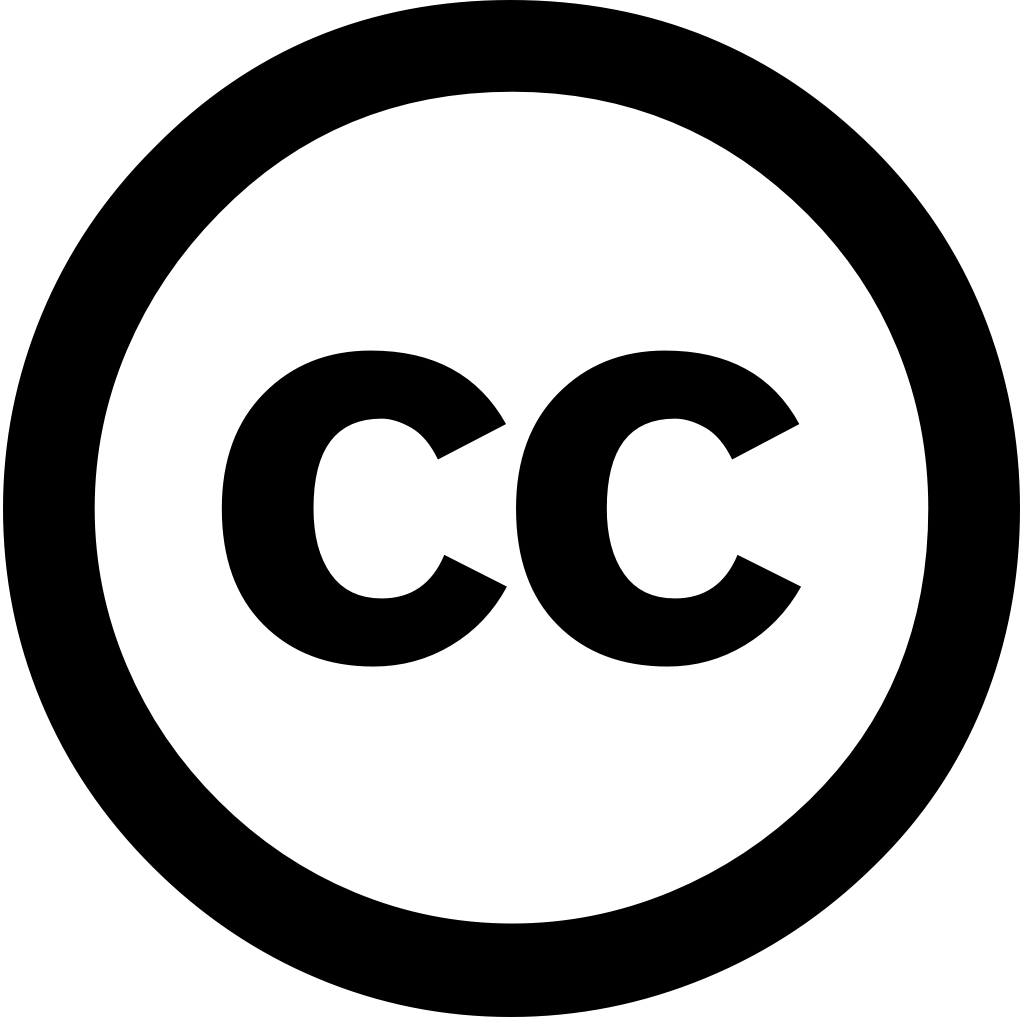
Published: Oct. 19, 2024
Language: Английский
Published: Oct. 19, 2024
Language: Английский
Published: Feb. 28, 2025
Language: Английский
Citations
0Journal of Clinical Medicine, Journal Year: 2025, Volume and Issue: 14(5), P. 1580 - 1580
Published: Feb. 26, 2025
Background/Objectives: Falls are the most common adverse in-hospital event, resulting in a considerable social and economic burden on individuals, their families, healthcare system. This study aims to develop implement an automatic coding system using large language models (LLMs) extract categorize free-text information (including location of fall any injury) from records. Methods: The used narrative description falls reported through Incident Reporting Risk Management Service Italian Local Health Authority Italy (name not disclosed as per research agreement). OpenAI application programming interface (API) was access generative pre-trained transformers (GPT) models, data falls, perform classification task. GPT-4-turbo were for Two independent reviewers manually coded information, representing gold standard Sensitivity, specificity, accuracy calculated evaluate performance Results: analysis included 187 records with event descriptions detailing 93 providing about presence or absence injury. showed excellent performance, sensitivity, values at least 0.913 detecting 0.953 Conclusions: GPT effectively extracted categorized even though text optimized GPT-based analysis. shows potential use LLMs clinical risk management research.
Language: Английский
Citations
0Journal of Psychosexual Health, Journal Year: 2025, Volume and Issue: unknown
Published: March 17, 2025
Large language model (LLM) chatbots have demonstrated significant capability in patient education by offering accessible, consistent, and personalized information. Their ability to interact real-time adapt responses based on user input makes them valuable tools enhancing knowledge engagement. Sexual developing countries faces substantial challenges. Sociocultural barriers, limited access comprehensive educational resources, stigmatization surrounding sexual health contribute inadequate education. Traditional methods often fail reach remote or underserved populations, there is a general shortage of qualified educators resources. Chatbots present promising solution these They can offer anonymous, culturally sensitive information health, overcoming barriers related stigma privacy. While LLM hold potential improve countries, their implementation must be carefully managed address challenges such as ensuring accuracy cultural sensitivity. There dearth research Hence, unmet need the reliability information, maintaining sensitivity, assessing engagement, integration with traditional methods, exploring long-term impact improving knowledge.
Language: Английский
Citations
0Informatics, Journal Year: 2025, Volume and Issue: 12(1), P. 33 - 33
Published: March 20, 2025
Background/Objectives: With advancements in Large Language Models (LLMs), counseling chatbots are becoming essential tools for delivering scalable and accessible mental health support. Traditional evaluation scales, however, fail to adequately capture the sophisticated capabilities of these systems, such as personalized interactions, empathetic responses, memory retention. This study aims design a robust comprehensive scale, Comprehensive Evaluation Scale LLM-Powered Counseling Chatbots (CES-LCC), using eDelphi method address this gap. Methods: A panel 16 experts psychology, artificial intelligence, human-computer interaction, digital therapeutics participated two iterative rounds. The process focused on refining dimensions items based qualitative quantitative feedback. Initial validation, conducted after assembling final version involved 49 participants CES-LCC evaluate an LLM-powered chatbot Self-Help Plus (SH+), Acceptance Commitment Therapy-based intervention stress management. Results: features 27 grouped into nine dimensions: Understanding Requests, Providing Helpful Information, Clarity Relevance Responses, Quality, Trust, Emotional Support, Guidance Direction, Memory, Overall Satisfaction. real-world validation revealed high internal consistency (Cronbach’s alpha = 0.94), although minor adjustments required specific dimensions, Responses. Conclusions: fills critical gap chatbots, offering standardized tool assessing their multifaceted capabilities. While preliminary results promising, further research is needed validate scale across diverse populations settings.
Language: Английский
Citations
0Frontiers in Public Health, Journal Year: 2025, Volume and Issue: 13
Published: March 25, 2025
Background Effectively addressing physical inactivity and the delayed development of fundamental movement skills in preschool children aged 3–6 years. Methods We assembled an interdisciplinary team experts to systematically validate LEG program indicators using Delphi method. This study thoroughly meticulously explored insights field identify necessary program’s role promoting sustainable activity preschoolers 3–6. Using a 5-point Likert scale Wilcoxon statistical techniques, this examines dynamic consensus among elucidates potential differences their views. Results After three rounds surveys, O1, O2, O3 reached all rounds. T5, I5, I14, C11, C12 disagreed due disciplinary differences, while C18 C22 cultural differences. Finally, achieved on objectives, seven tasks, 17 indicators, 25 content areas. The results strongly convey experts’ positive perceptions Conclusion indicates that LEG-structured curriculum we developed are scientific reliable, aligning with mental understanding fosters in-depth integration early childhood education education, providing foundation for enhancing
Language: Английский
Citations
0Systems, Journal Year: 2025, Volume and Issue: 13(4), P. 281 - 281
Published: April 10, 2025
The integration of large language models (LLMs) into remote healthcare has the potential to revolutionize medication management by enhancing communication, improving adherence, and supporting clinical decision-making. This study aims explore role LLMs in management, focusing on their impact. paper comprehensively reviews existing literature, medical LLM cases, commercial applications healthcare. It also addresses technical, ethical, regulatory challenges related use artificial intelligence (AI) this context. review methodology includes analyzing studies applications, comparing impact, identifying gaps for future research development. reveals that have shown significant communication between patients providers, adherence monitoring, decision-making management. Compared traditional reminder systems, AI systems a 14% higher rate rates pilot studies. However, there are notable challenges, including data privacy concerns, system issues, ethical dilemmas AI-driven decisions such as bias transparency. Overall, offers comprehensive analysis both transformative key be addressed. provides insights policymakers, researchers optimizing
Language: Английский
Citations
0Arthroscopy The Journal of Arthroscopic and Related Surgery, Journal Year: 2024, Volume and Issue: unknown
Published: Dec. 1, 2024
Language: Английский
Citations
2Published: July 7, 2024
Language: Английский
Citations
1European Heart Journal - Digital Health, Journal Year: 2024, Volume and Issue: 5(6), P. 712 - 724
Published: Sept. 12, 2024
Abstract Aims This study aims to assess the feasibility and impact of implementation ChatGLM for real-world data (RWD) extraction in hospital settings. The primary focus this research is on effectiveness ChatGLM-driven compared with that manual processes associated electronic source repository (ESDR) system. Methods results researchers developed ESDR system, which integrates ChatGLM, case report forms (eCRFs), health records. LLaMA (Large Language Model Meta AI) model was also deployed compare accuracy free-text forms. A single-centre retrospective cohort served as a pilot case. Five eCRF 63 subjects, including discharge medication, were evaluated. Data collection involved medical prescription records collected from 13 departments. ChatGLM-assisted process an estimated efficiency improvement 80.7% transcription time. initial input 99.59%, 77.13%, 43.86%. challenges use prompt design, output consistency, verification, integration information systems. Conclusion main contribution validate tools address interoperability transparency using RWD Chinese
Language: Английский
Citations
1Frontiers in Artificial Intelligence, Journal Year: 2024, Volume and Issue: 7
Published: Aug. 16, 2024
Introduction Radiologists frequently lack direct patient contact due to time constraints. Digital medical interview assistants aim facilitate the collection of health information. In this paper, we propose leveraging conversational agents realize a assistant history taking, while at same offering patients opportunity ask questions on examination. Methods MIA, digital assistant, was developed using person-based design approach, involving opinions and expert knowledge during development with specific use case in collecting information before mammography MIA consists two modules: module question answering (Q&A). To ensure interoperability clinical systems, HL7 FHIR store exchange results collected by interaction. The system evaluated according an existing evaluation framework that covers broad range aspects related technical quality agent including usability, but also accessibility security. Results Thirty-six recruited from Swiss hospitals (Lindenhof group Inselspital, Bern) organizations conducted usability test. favorably received participants, who particularly noted clarity communication. However, there is room for improvement perceived conversation, provided, protection privacy. Q&A achieved precision 0.51, recall 0.87 F-Score 0.64 based 114 asked participants. Security require improvements. Conclusion applied process described paper can provide best practices future assistants. application standardized helped saving ensures comparability results.
Language: Английский
Citations
0