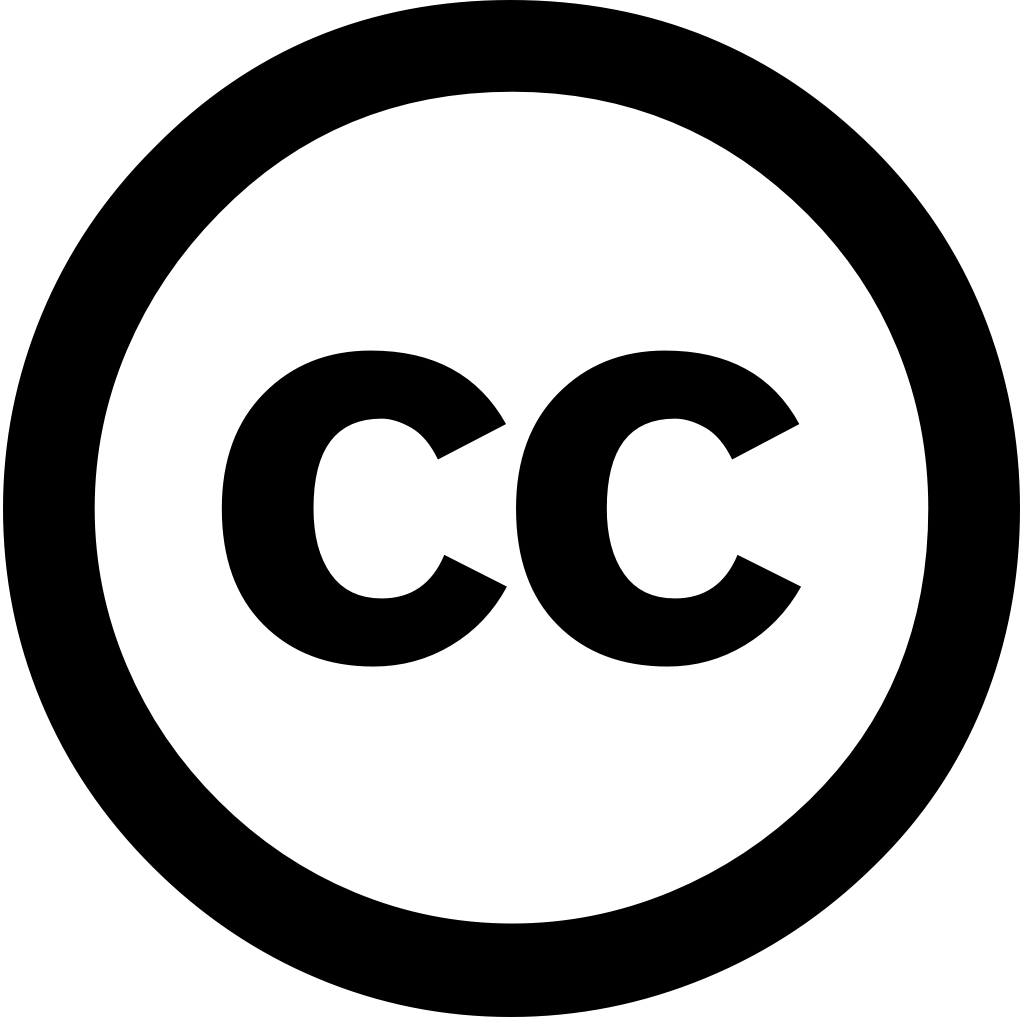
Research Square (Research Square), Journal Year: 2024, Volume and Issue: unknown
Published: Nov. 20, 2024
Language: Английский
Research Square (Research Square), Journal Year: 2024, Volume and Issue: unknown
Published: Nov. 20, 2024
Language: Английский
Journal of Medical Systems, Journal Year: 2025, Volume and Issue: 49(1)
Published: Feb. 18, 2025
Language: Английский
Citations
1International Journal of Medical Informatics, Journal Year: 2024, Volume and Issue: 192, P. 105654 - 105654
Published: Oct. 14, 2024
Language: Английский
Citations
6Frontiers in Artificial Intelligence, Journal Year: 2025, Volume and Issue: 7
Published: Jan. 14, 2025
Introduction Generating physician letters is a time-consuming task in daily clinical practice. Methods This study investigates local fine-tuning of large language models (LLMs), specifically LLaMA models, for letter generation privacy-preserving manner within the field radiation oncology. Results Our findings demonstrate that base without fine-tuning, are inadequate effectively generating letters. The QLoRA algorithm provides an efficient method intra-institutional LLMs with limited computational resources (i.e., single 48 GB GPU workstation hospital). fine-tuned LLM successfully learns oncology-specific information and generates institution-specific style. ROUGE scores generated summary reports highlight superiority 8B LLaMA-3 model over 13B LLaMA-2 model. Further multidimensional evaluations 10 cases reveal that, although has capacity to generate content beyond provided input data, it salutations, diagnoses treatment histories, recommendations further treatment, planned schedules. Overall, benefit was rated highly by experts (average score 3.4 on 4-point scale). Discussion With careful review correction, automated LLM-based significant practical value.
Language: Английский
Citations
0Postgraduate Medical Journal, Journal Year: 2025, Volume and Issue: unknown
Published: Feb. 17, 2025
A discharge summary is an important clinical document that summarizes a patient's information and relevant events occurred during hospitalization. It serves as detailed handover of the most recent updated medical case records to general practitioners, who continue longitudinal follow-up with patients in community future care providers. copy redacted/abbreviated form also usually given their caregivers so information, such diagnoses, medication changes, return advice, plans, clearly documented. However, reality, summaries are often written by junior physicians may be inexperienced or have lacked training this area, audits reveal poorly unclear, inaccurate, lack details. Therefore, article, we sought develop simple "DISCHARGED" framework outlines components derived from systematic search literature further discuss several pedagogical strategies for assessing writing.
Language: Английский
Citations
0Published: Jan. 1, 2025
Language: Английский
Citations
0Journal of the American Academy of Orthopaedic Surgeons, Journal Year: 2025, Volume and Issue: unknown
Published: March 18, 2025
Purpose: Artificial intelligence (AI) has been increasingly studied within medical education and clinical practice. At present, it remains uncertain if AI is being used to write personal statements (PSs) for orthopaedic surgery residency applications. Our purpose was analyze PS that were submitted our institution determine the rate of utilization these texts. Methods: Four groups created comparison: 100 before release ChatGTP (PRE-PS), after Chat Generative Pre-Trained Transformers introduction (POST-PS), 10 AI-generated (AI-PS), hybrid (H-PS), which contained both human-generated text. For each four groups, detection software (GPT-Zero) quantify percentage text, “mixed” In addition, provided level confidence (highly confident, moderately uncertain) with respect “final verdict” versus Results: The text in PRE-PS, POST-PS, H-PS, AI-PS 94%, 93%, 28%, 0% respectively. All 200 (100%) program had a final verdict “human” >90%. By contrast, all (H-PS groups) “AI.” Verdict group 100%. Conclusion: Orthopaedic applicants do not appear, at be using create included their (GPTZero) appears able accurately detect PSs Considering increasing role development software, future investigations should endeavor explore results change over time. Similar journals, guidelines established pertain use on postgraduate training Level Evidence: V—Nonclinical.
Language: Английский
Citations
0BMC Medical Education, Journal Year: 2025, Volume and Issue: 25(1)
Published: March 19, 2025
To assess the ability of General Practice (GP) Trainees to detect AI-generated hallucinations in simulated clinical practice, ChatGPT-4o was utilized. The were categorized into three types based on accuracy answers and explanations: (1) correct with incorrect or flawed explanations, (2) explanations that contradict factual evidence, (3) explanations. This multi-center, cross-sectional survey study involved 142 GP Trainees, all whom undergoing Specialist Training volunteered participate. evaluated consistency ChatGPT-4o, as well Trainees' response time, accuracy, sensitivity (d'), tendencies (β). Binary regression analysis used explore factors affecting identify errors generated by ChatGPT-4o. A total 137 participants included, a mean age 25.93 years. Half unfamiliar AI, 35.0% had never it. ChatGPT-4o's overall 80.8%, which slightly decreased 80.1% after human verification. However, for professional practice (Subject 4) only 57.0%, verification, it dropped further 44.2%. 87 identified, primarily occurring at application evaluation levels. detecting these 55.0%, (d') 0.39. Regression revealed shorter times (OR = 0.92, P 0.02), higher self-assessed AI understanding 0.16, 0.04), more frequent use 10.43, 0.01) associated stricter error detection criteria. concluded trainees faced challenges identifying errors, particularly scenarios. highlights importance improving literacy critical thinking skills ensure effective integration medical education.
Language: Английский
Citations
0Journal of Children s Orthopaedics, Journal Year: 2025, Volume and Issue: unknown
Published: April 15, 2025
Objective: To evaluate the performance of three large language models in answering questions regarding pediatric developmental dysplasia hip. Methods: We formulated 18 open-ended clinical both Chinese and English established a gold standard set answers to benchmark responses models. These were presented ChatGPT-4o, Gemini, Claude 3.5 Sonnet. The evaluated by two independent reviewers using 5-point scale. average score, rounded nearest whole number, was taken as final score. A score 4 or 5 indicated an accurate response, whereas 1, 2, 3 inaccurate response. Results: raters demonstrated high level agreement scoring answers, with weighted Kappa coefficients 0.865 for ( p < 0.001) 0.875 0.001). No significant differences observed among terms accuracy when questions, rates 83.3%, 77.8%, 77.8% Sonnet, Gemini = 1), 72.2% 0.761). In addition, there no difference same model between settings. Conclusions: Large demonstrate delivering information on hip, maintaining consistent across English, which suggests their potential utility medical support tools. Level evidence: II.
Language: Английский
Citations
0IntechOpen eBooks, Journal Year: 2024, Volume and Issue: unknown
Published: Dec. 3, 2024
The digitalization of healthcare systems, particularly through Electronic Health Records (EHRs), presents both opportunities and challenges. This chapter delves into the transformative potential integrating Artificial Intelligence (AI) with advanced cybersecurity measures in EHR systems. impressive capabilities AI models data management, predictive analytics, automation are explored for their role enhancing patient outcomes streamlining operations. study addresses critical issues, including breaches ransomware, emphasizing necessity encryption, multi-factor authentication, continuous monitoring. It examines how AI-driven threat detection automated incident response can proactively safeguard sensitive data, also highlighting challenges that may appear integration systems along addressing need robust interoperability standards comprehensive governance frameworks to mitigate cyber threats. discussion extends toward future vision includes innovation strategic investment create a more efficient, secure, patient-centric environment. analysis highlights synergistic revolutionizing overall quality delivery.
Language: Английский
Citations
2Research Square (Research Square), Journal Year: 2024, Volume and Issue: unknown
Published: Nov. 20, 2024
Language: Английский
Citations
0