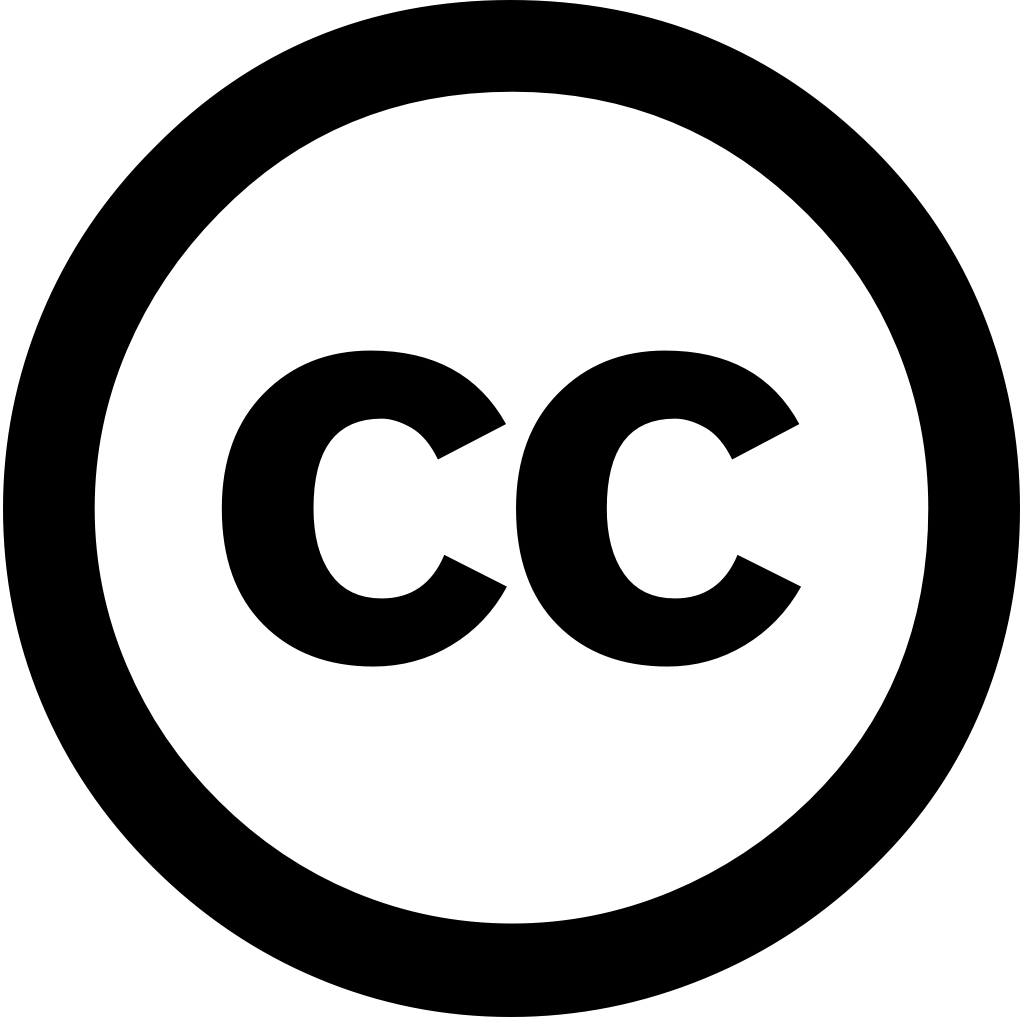
Computational and Structural Biotechnology Journal, Journal Year: 2024, Volume and Issue: 28, P. 9 - 15
Published: Dec. 26, 2024
Language: Английский
Computational and Structural Biotechnology Journal, Journal Year: 2024, Volume and Issue: 28, P. 9 - 15
Published: Dec. 26, 2024
Language: Английский
Medical Sciences, Journal Year: 2024, Volume and Issue: 12(4), P. 67 - 67
Published: Nov. 17, 2024
Quantum computing (QC) represents a paradigm shift in computational power, offering unique capabilities for addressing complex problems that are infeasible classical computers. This review paper provides detailed account of the current state QC, with particular focus on its applications within medicine. It explores fundamental concepts such as qubits, superposition, and entanglement, well evolution QC from theoretical foundations to practical advancements. The covers significant milestones where has intersected medical research, including breakthroughs drug discovery, molecular modeling, genomics, diagnostics. Additionally, key quantum techniques algorithms, machine learning (QML), quantum-enhanced imaging explained, highlighting their relevance healthcare. also addresses challenges field, hardware limitations, scalability, integration clinical environments. Looking forward, discusses potential quantum–classical hybrid systems emerging innovations hardware, suggesting how these advancements may accelerate adoption research practice. By synthesizing reliable knowledge presenting it through comprehensive lens, this serves valuable reference researchers interested transformative
Language: Английский
Citations
8International Journal of Human-Computer Interaction, Journal Year: 2025, Volume and Issue: unknown, P. 1 - 19
Published: Jan. 8, 2025
The advancement of artificial intelligence technology has enabled chatbots to mimic human conversations the point being nearly indistinguishable from humans. This study investigates impact chatbot proactivity on user experience, considering task types and characteristics. Experiments were conducted using proactive non-proactive for business-related non-business-related tasks, evaluating characteristics (age, gender, AI experience level, education personality traits) (perceived usefulness, perceived ease use, satisfaction, trust). results showed that positively evaluated in terms trust, but no significant difference was found use compared chatbots. Additionally, traits (extraversion, agreeableness) significantly influenced experience. highlights critical role providing satisfying interaction experiences emphasizes importance designing
Language: Английский
Citations
1JMIR Cancer, Journal Year: 2025, Volume and Issue: 11, P. e66633 - e66633
Published: Feb. 18, 2025
This Viewpoint proposes a robust framework for developing medical chatbot dedicated to radiotherapy education, emphasizing accuracy, reliability, privacy, ethics, and future innovations. By analyzing existing research, the evaluates performance identifies challenges such as content bias, system integration. The findings highlight opportunities advancements in natural language processing, personalized learning, immersive technologies. When designed with focus on ethical standards large model–based chatbots could significantly impact education health care delivery, positioning them valuable tools developments globally.
Language: Английский
Citations
1Algorithms, Journal Year: 2025, Volume and Issue: 18(3), P. 156 - 156
Published: March 9, 2025
Medical decision-making is increasingly integrating quantum computing (QC) and machine learning (ML) to analyze complex datasets, improve diagnostics, enable personalized treatments. While QC holds the potential accelerate optimization, drug discovery, genomic analysis as hardware capabilities advance, current implementations remain limited compared classical in many practical applications. Meanwhile, ML has already demonstrated significant success medical imaging, predictive modeling, decision support. Their convergence, particularly through (QML), presents opportunities for future advancements processing high-dimensional healthcare data improving clinical outcomes. This review examines foundational concepts, key applications, challenges of these technologies healthcare, explores their synergy solving problems, outlines directions quantum-enhanced decision-making.
Language: Английский
Citations
1Informatics in Medicine Unlocked, Journal Year: 2025, Volume and Issue: unknown, P. 101618 - 101618
Published: Jan. 1, 2025
Language: Английский
Citations
0Journal of Radiation Research and Applied Sciences, Journal Year: 2025, Volume and Issue: 18(2), P. 101353 - 101353
Published: Feb. 13, 2025
Language: Английский
Citations
0Humanities and Social Sciences Communications, Journal Year: 2025, Volume and Issue: 12(1)
Published: March 6, 2025
Language: Английский
Citations
0Journal of Multidisciplinary Healthcare, Journal Year: 2025, Volume and Issue: Volume 18, P. 1629 - 1638
Published: March 1, 2025
This study aimed to assess and compare the performance of ChatGPT iFlytek Spark, two AI-powered large language models (LLMs), in generating clinical recommendations aligned with expert consensus on Enhanced Recovery After Surgery (ERAS)-guided ankle fracture treatment. aims determine applicability reliability AI supporting ERAS protocols for optimized patient outcomes. A qualitative comparative analysis was conducted using 35 structured questions derived from Expert Consensus Optimizing Ankle Fracture Treatment Protocols under Principles. Questions covered preoperative preparation, intraoperative management, postoperative pain control rehabilitation, complication management. Responses Spark were independently evaluated by experienced trauma orthopedic specialists based relevance, consistency consensus, depth reasoning. demonstrated higher alignment (29/35 questions, 82.9%), particularly comprehensive perioperative recommendations, detailed medical rationales, treatment plans. However, discrepancies noted blood pressure management antiemetic selection. 22/35 (62.9%), but responses often more generalized, less clinically detailed, occasionally inconsistent best practices. Agreement between observed 23/35 (65.7%), generally exhibiting greater specificity, timeliness, precision its recommendations. LLMs, ChatGPT, show promise decision-making ERAS-guided While provided accurate contextually relevant responses, inconsistencies highlight need further refinement, validation, integration. Spark's lower conformity suggests potential differences training data underlying algorithms, underscoring variability AI-generated advice. To optimize AI's role care, future research should focus enhancing guidelines, improving model transparency, integrating physician oversight ensure safe effective applications.
Language: Английский
Citations
0LatIA, Journal Year: 2025, Volume and Issue: 3, P. 328 - 328
Published: March 29, 2025
Artificial intelligence (AI) has revolutionized language learning by enabling personalized and adaptive education; however, these advancements also raise ethical privacy concerns, including algorithmic bias, data security risks, a lack of transparency in AI-driven decision-making. This study examines challenges, focusing on fairness, linguistic diversity, the balance between automated human instruction, with goal proposing guidelines for responsible adoption AI education. Through literature review comparative analysis, risks AI-powered tools were explored, assessing bias detection algorithms, frameworks, privacy-preserving techniques to identify best practices. The findings indicate that tend exhibit biases disadvantage underrepresented groups, raising concerns about fairness while exposing due inadequate measures. Implementing frameworks incorporate fairness-aware explainable models, robust protection mechanisms enhances user trust security. Therefore, addressing issues is essential ensuring integration education, where hybrid approach combining instruction emerges as most solution. Lastly, future research should focus regulatory compliance models strengthen ethics
Language: Английский
Citations
0Open Research Europe, Journal Year: 2025, Volume and Issue: 5, P. 65 - 65
Published: April 17, 2025
Language: Английский
Citations
0