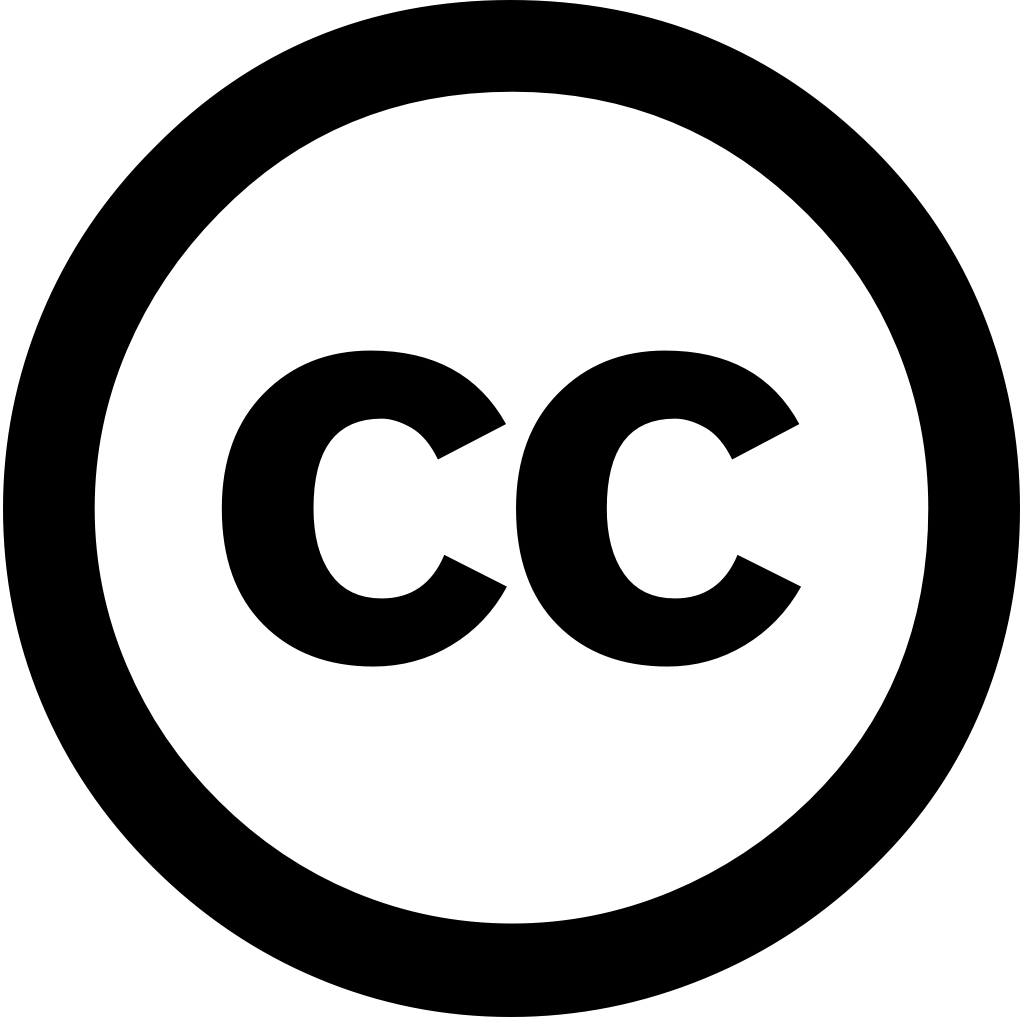
Peer Community Journal, Journal Year: 2025, Volume and Issue: 5
Published: April 9, 2025
It is vital to understand the genomic basis of differences in ecologically important traits if we are impact global change on biodiversity and enhance our ability for targeted intervention. This study explores potential a novel genome-wide association (GWAS) approach identifying loci underlying quantitative polygenic natural populations, based phenotypic population means allele frequency data as obtained e.g. by PoolSeq approaches. Extensive genetic forward simulations demonstrate that generally effective oligogenic moderately relatively insensitive low heritability. However, applicability limited highly architectures pronounced structure. The required sample size moderate with very good results being already few dozen populations scored. When combined machine learning feature selection, method performs well predicting means. efficiency method, particularly when using pooled sequencing bulk phenotyping, makes GWAS studies more accessible research genomics. Moreover, direct comparison individual GWAS, proposed performed constistently better regard number true positive identified prediction accuracy. Overall, this highlights promise popGWAS dissecting complex populations.
Language: Английский