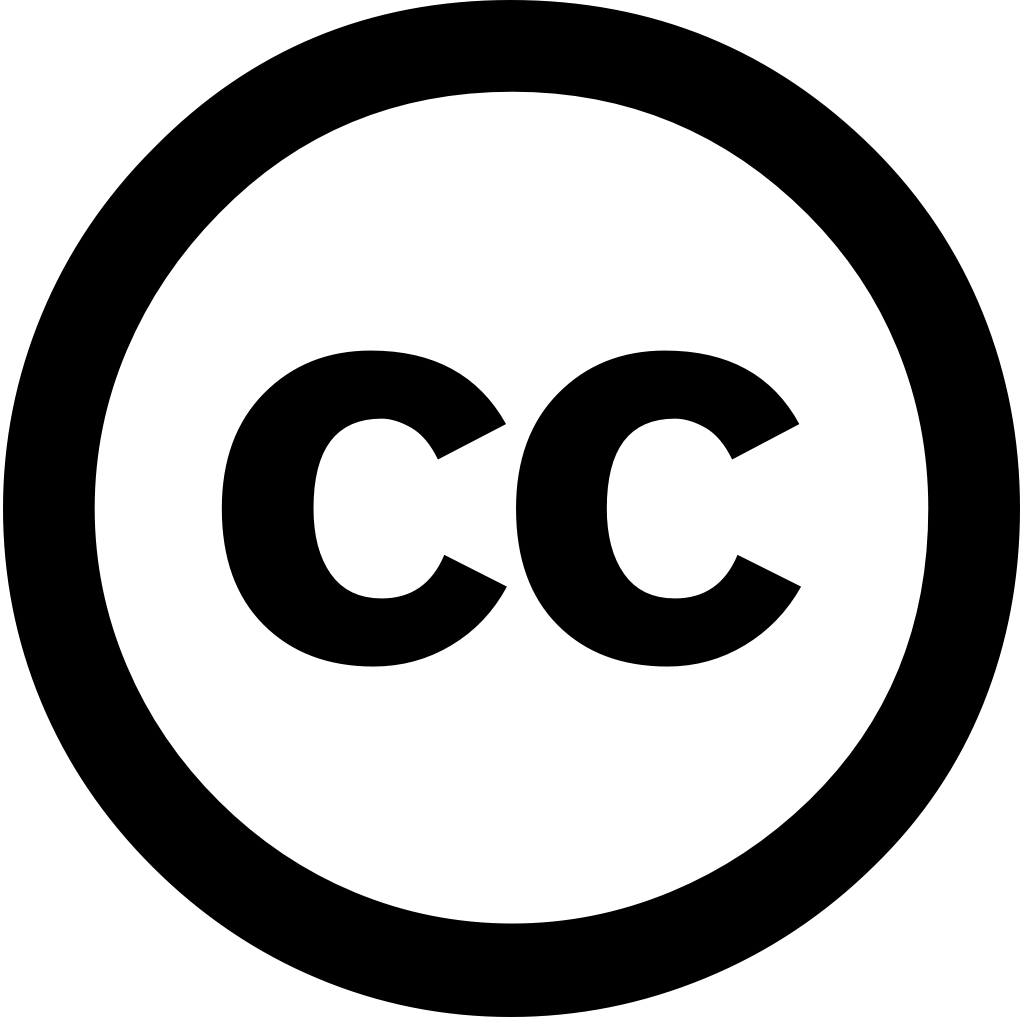
Published: Nov. 1, 2023
Machine learning force fields (MLFFs) have emerged as a new method for molecular simulation that combines the accuracy of ab initio approaches with computational efficiency classical fields. However, performance MLFFs in describing solvation configuration has yet to be explored. Here, we compare and contrast ANI-1ccx MLFF, GAFF field, dynamics (AIMD) simulating nine organic solutes acetonitrile solvents. We examine solvent-solute interaction described by these methods from four aspects: solute conformation landscape, shell structure, structure O-H⋯N hydrogen bond, first shell. For description, both yield minima agree density functional theory optimization rigid solutes. their results diverge flexible agrees better AIMD on location solvent than does. description bond formed between solute, generates stronger bonds shorter lengths, wider angles, longer lifetimes, agreeing DFT-optimized structure. also describes more frequent exchange molecules out GAFF. Our study demonstrates potential benefits utilizing MLFF solution-phase generating configurations.
Language: Английский