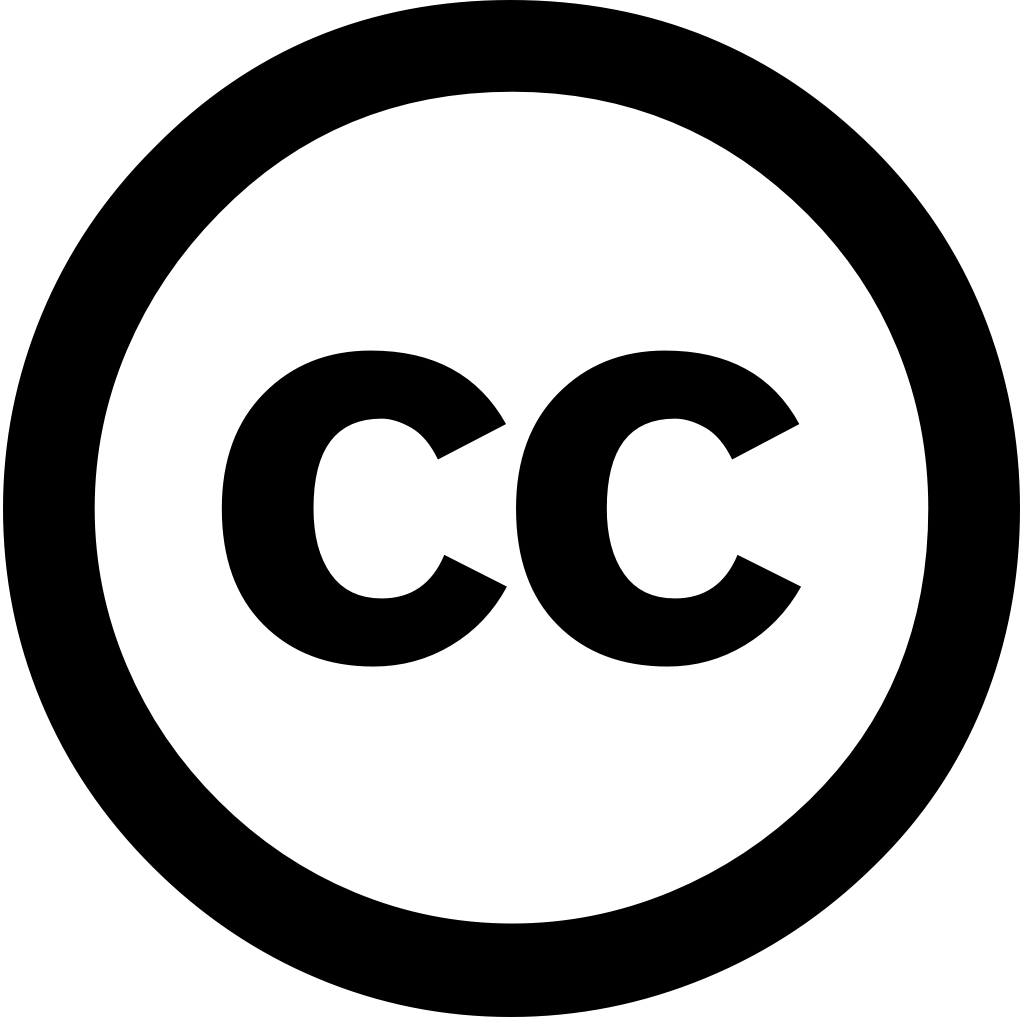
Published: March 11, 2024
H-Bonding catalysts (HBCs) has gained widespread success in improving the selectivity of organic synthesis. The complexity potentially engaging noncovalent interactions have imposed significant challenges deciphering contributing factors these catalytic reactions. Herein, we present use interpretable machine learning on HBCs applied poly(disulfide) synthesis, wherein epiquinine-derived thiourea enable simultaneous regioselectivity control and rate acceleration over otherwise unselective already rapid polymerization. A training set 28 with variation phenyl substituents were synthesized tested to gather experimental observables: apparent (kp) (Pss). Considering limited data size, feature-sensitive XGBoost algorithm for supervised learning. Upon screening 64 relevant descriptors, a reasonable fitting observables was established kp (R2 = 0.76) Pss 0.91), key catalyst features necessary achieving high reaction rates deconvoluted. model suggests that sterically hindered, heavy atom-containing or strongly electro-withdrawing groups HBC adversely affect rate. Substituents enhancing electrostatic potential aromatic N atom are beneficial regioregularity, while presence ortho-substituents ring is unfavorable.
Language: Английский