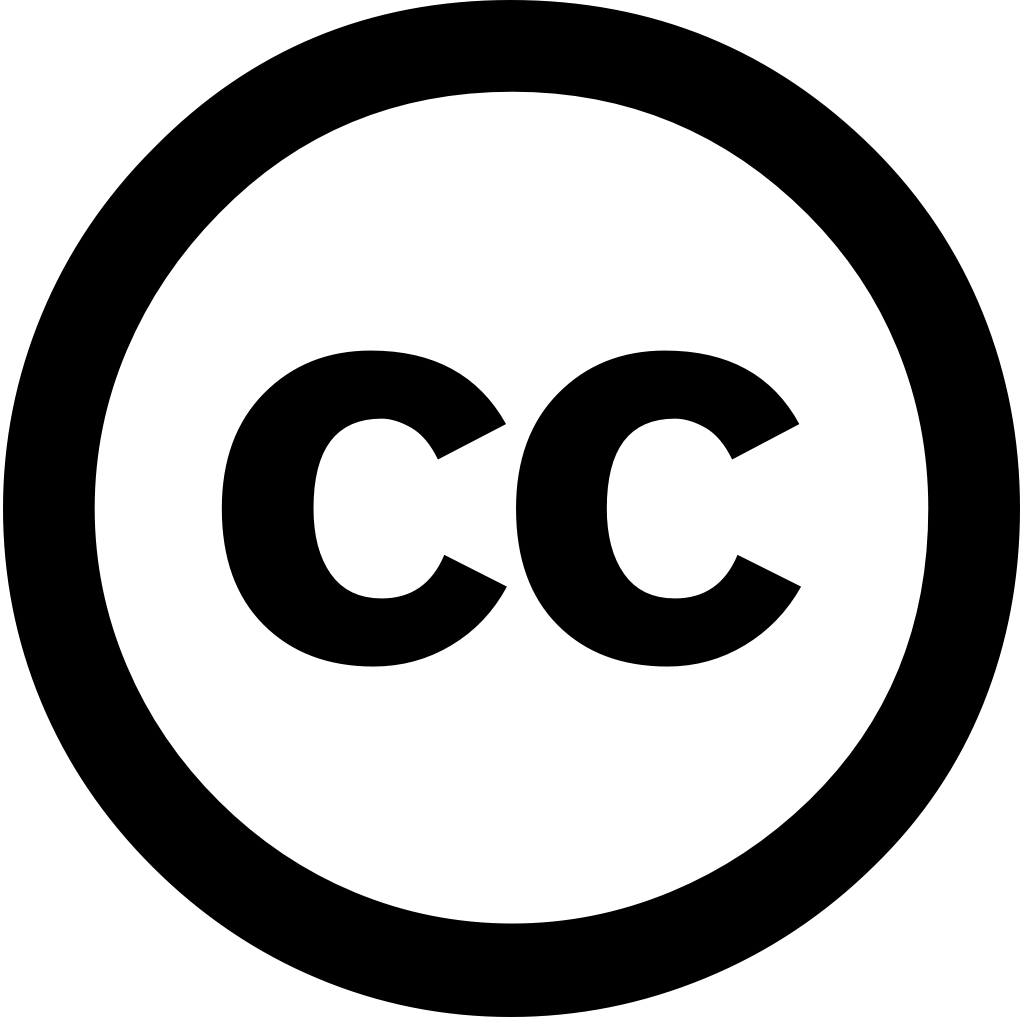
Ain Shams Engineering Journal, Journal Year: 2024, Volume and Issue: unknown, P. 103086 - 103086
Published: Oct. 1, 2024
Language: Английский
Ain Shams Engineering Journal, Journal Year: 2024, Volume and Issue: unknown, P. 103086 - 103086
Published: Oct. 1, 2024
Language: Английский
Renewable and Sustainable Energy Reviews, Journal Year: 2024, Volume and Issue: 196, P. 114366 - 114366
Published: March 15, 2024
Language: Английский
Citations
21Renewable and Sustainable Energy Reviews, Journal Year: 2024, Volume and Issue: 201, P. 114609 - 114609
Published: June 10, 2024
Language: Английский
Citations
17Renewable and Sustainable Energy Reviews, Journal Year: 2025, Volume and Issue: 214, P. 115547 - 115547
Published: Feb. 28, 2025
Language: Английский
Citations
2Renewable and Sustainable Energy Reviews, Journal Year: 2024, Volume and Issue: 197, P. 114408 - 114408
Published: April 3, 2024
Language: Английский
Citations
7Buildings, Journal Year: 2024, Volume and Issue: 14(9), P. 2748 - 2748
Published: Sept. 2, 2024
In response to increasing global temperatures and energy demands, optimizing buildings’ efficiency, particularly in hot climates, is an urgent challenge. While current research often relies on conventional estimation methods, there has been a decrease the efforts dedicated leveraging AI-based methodologies as technology advances. This implies dearth of multiparameter examinations AI-driven extreme case studies. For this reason, study aimed enhance performance residential buildings climates Dubai Riyadh by integrating Building Information Modeling (BIM) Machine Learning (ML). Detailed BIM models typical villa these regions were created using Revit, incorporating conventional, modern, green building envelopes (BEs). These served basis for simulations conducted with Green Studio (GBS) Insight, focusing crucial features such floor area, external internal walls, windows, flooring, roofing, orientation, infiltration, daylighting, more. To predict Energy Use Intensity (EUI), four ML algorithms, namely, Gradient Boosting (GBM), Random Forest (RF), Support Vector (SVM), Lasso Regression (LR), employed. GBM consistently outperformed others, demonstrating superior prediction accuracy R2 0.989. indicates that model explains 99% variance EUI, highlighting its effectiveness capturing relationships between consumption. Feature importance analysis (FIA) revealed roofs (29% scenarios (DS) 40% (RS)), walls (19% DS 29% RS), windows (15% 9% RS) have most impact Additionally, explored potential optimization, cavity RS double brick VIP insulation DS. The findings paper should be interpreted light certain limitations but they underscore combining sustainable design, offering actionable insights enhancing efficiency climates.
Language: Английский
Citations
6Cleaner Energy Systems, Journal Year: 2024, Volume and Issue: 10, P. 100167 - 100167
Published: Dec. 10, 2024
Language: Английский
Citations
6Alexandria Engineering Journal, Journal Year: 2023, Volume and Issue: 82, P. 377 - 388
Published: Oct. 15, 2023
This paper introduces a metamaterial energy harvester that is compact, highly efficient, and capable of operating at wide angles. The proposed design has an outer ring resonator housing inverted T-shaped resonators, it can operate two distinct frequencies, 3.2 GHz 5.4 GHz. structure's impedance carefully designed to align with free space, ensuring efficient capture incident electromagnetic power minimal reflection. enables the resistor load receive in most manner. Based on simulation findings, exhibits notably higher conversion efficiency around 97 %. To ensure accuracy reliability outcomes, we fabricated 3x3 cell array conducted experimental tests within anechoic chamber. results exhibit strong correlation. Existing metamaterial-based harvesting designs frequently confront size, absorption band, polarization sensitivity limitations. Our compact distinguished by its ability accomplish near-unity greater efficiencies desired frequency bands. makes ideal option for systems wireless sensor networks prioritizing size.
Language: Английский
Citations
16Energy, Journal Year: 2024, Volume and Issue: 301, P. 131642 - 131642
Published: May 12, 2024
Language: Английский
Citations
5Renewable and Sustainable Energy Reviews, Journal Year: 2024, Volume and Issue: 199, P. 114532 - 114532
Published: May 17, 2024
Language: Английский
Citations
5Advances in computational intelligence and robotics book series, Journal Year: 2025, Volume and Issue: unknown, P. 467 - 482
Published: March 13, 2025
Artificial intelligence (AI) and machine learning (ML) transform building design by optimizing energy consumption reducing costs. These technologies, integrated with Building Information Modeling (BIM) platforms like Revit, enable precise predictions, real-time optimization, renewable integration, achieving notable savings, such as up to 37% in HVAC systems. This review explores methodologies for applying AI design, including data extraction, simulation, validation using tools Autodesk Insight 360. It addresses challenges availability ethical concerns while highlighting AI's potential enhance efficiency sustainability. Future research suggests developing hybrid models, incorporating cognitive principles, leveraging emerging technologies IoT blockchain advance sustainable practices redefine processes.
Language: Английский
Citations
0