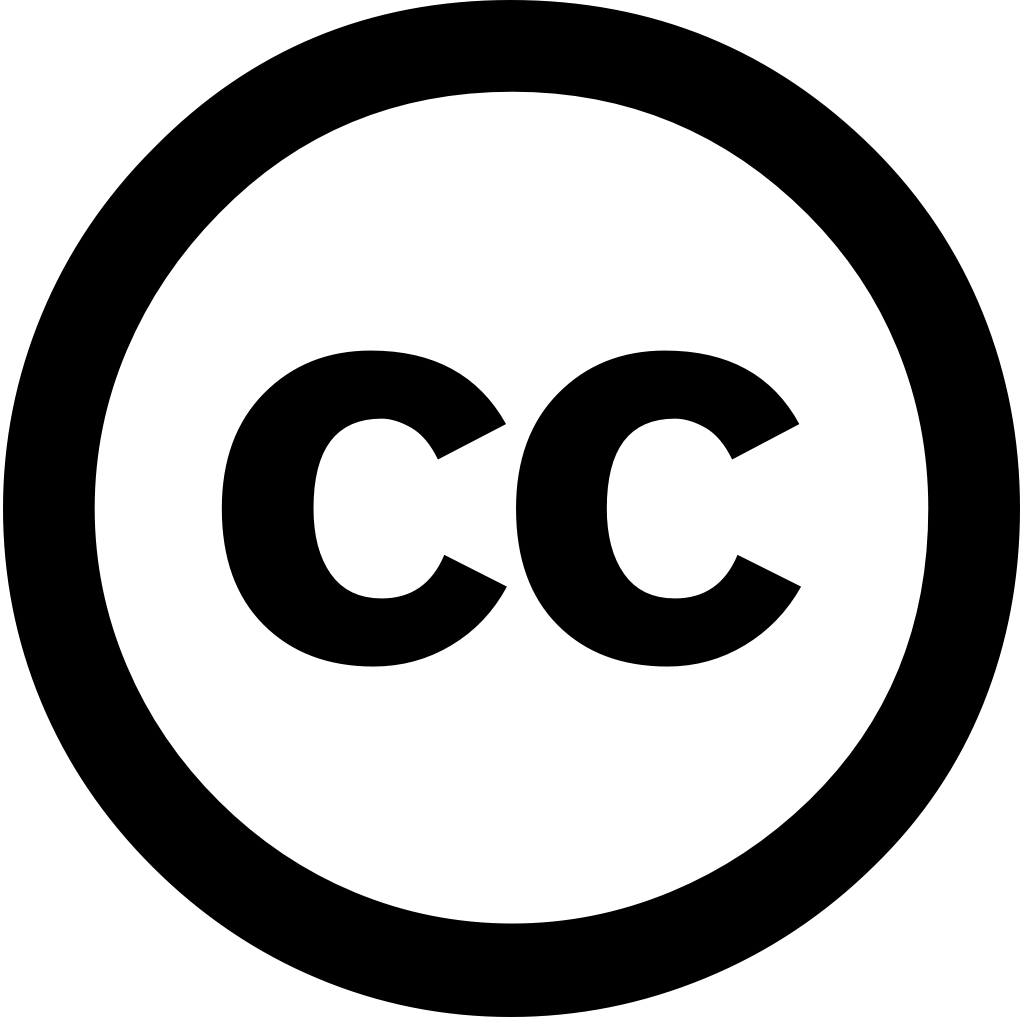
Biomedicines, Journal Year: 2024, Volume and Issue: 12(11), P. 2485 - 2485
Published: Oct. 29, 2024
Lymphoma is one of the most common cancers worldwide, categorized into Hodgkin lymphoma and non-Hodgkin lymphoma.
Language: Английский
Biomedicines, Journal Year: 2024, Volume and Issue: 12(11), P. 2485 - 2485
Published: Oct. 29, 2024
Lymphoma is one of the most common cancers worldwide, categorized into Hodgkin lymphoma and non-Hodgkin lymphoma.
Language: Английский
Computers in Biology and Medicine, Journal Year: 2024, Volume and Issue: 179, P. 108827 - 108827
Published: July 3, 2024
Radiomics, the high-throughput extraction of quantitative imaging features from medical images, holds immense potential for advancing precision medicine in oncology and beyond. While radiomics applied to positron emission tomography (PET) offers unique insights into tumor biology treatment response, it is imperative elucidate challenges constraints inherent this domain facilitate their translation clinical practice. This review examines limitations applying PET imaging, synthesizing findings last five years (2019-2023) highlights significance addressing these realize full molecular imaging. A comprehensive search was conducted across multiple electronic databases, including PubMed, Scopus, Web Science, using keywords relevant issues Only studies published peer-reviewed journals were eligible inclusion review. Although many have highlighted predicting assessing heterogeneity, enabling risk stratification, personalized therapy selection, various regarding practical implementation proposed models still need be addressed. illustrates cancer types, encompassing both phantom investigations. The analyzed highlight importance reproducible segmentation methods, standardized pre-processing post-processing methodologies, create large multicenter registered a centralized database promote continuous validation integration
Language: Английский
Citations
17Cancers, Journal Year: 2024, Volume and Issue: 16(20), P. 3511 - 3511
Published: Oct. 17, 2024
Lymphoma, encompassing a wide spectrum of immune system malignancies, presents significant complexities in its early detection, management, and prognosis assessment since it can mimic post-infectious/inflammatory diseases. The heterogeneous nature lymphoma makes challenging to definitively pinpoint valuable biomarkers for predicting tumor biology selecting the most effective treatment strategies. Although molecular imaging modalities, such as positron emission tomography/computed tomography (PET/CT), specifically
Language: Английский
Citations
4Cancer Innovation, Journal Year: 2025, Volume and Issue: 4(2)
Published: Feb. 20, 2025
Breast cancer (BC) remains a significant threat to women's health worldwide. The oncology field had an exponential growth in the abundance of medical images, clinical information, and genomic data. With its continuous advancement refinement, artificial intelligence (AI) has demonstrated exceptional capabilities processing intricate multidimensional BC-related AI proven advantageous various facets BC management, encompassing efficient screening diagnosis, precise prognosis assessment, personalized treatment planning. However, implementation into precision medicine practice presents ongoing challenges that necessitate enhanced regulation, transparency, fairness, integration multiple pathways. In this review, we provide comprehensive overview current research related BC, highlighting extensive applications throughout whole cycle management potential for innovative impact. Furthermore, article emphasizes significance constructing patient-oriented algorithms. Additionally, explore opportunities directions within burgeoning field.
Language: Английский
Citations
0Image and Vision Computing, Journal Year: 2025, Volume and Issue: unknown, P. 105495 - 105495
Published: March 1, 2025
Language: Английский
Citations
0European Journal of Nuclear Medicine and Molecular Imaging, Journal Year: 2025, Volume and Issue: unknown
Published: May 2, 2025
Language: Английский
Citations
0Medical Review, Journal Year: 2025, Volume and Issue: unknown
Published: Jan. 23, 2025
Abstract The diagnosis and prognosis of Prostate cancer (PCa) have undergone a significant transformation with the advent prostate-specific membrane antigen (PSMA)-targeted positron emission tomography (PET) imaging. PSMA-PET imaging has demonstrated superior performance compared to conventional methods by detecting PCa, its biochemical recurrence, sites metastasis higher sensitivity specificity. That now intersects rapid advances in artificial intelligence (AI) – including emergence generative AI. However, there are unique clinical challenges associated that still need be addressed ensure continued widespread integration into care research trials. Some those very wide dynamic range lesion uptake, benign uptake organs may adjacent disease, insufficient large datasets for training AI models, as well artifacts images. Generative e.g., adversarial networks, variational autoencoders, diffusion language models played crucial roles overcoming many such across various modalities, PET, computed tomography, magnetic resonance imaging, ultrasound, etc. In this review article, we delve potential role enhancing robustness utilization image analysis, drawing insights from existing literature while also exploring current limitations future directions domain.
Language: Английский
Citations
0Scientific Reports, Journal Year: 2025, Volume and Issue: 15(1)
Published: Feb. 3, 2025
Abstract The prognostic value of metabolic tumor burden parameters obtained from 18 F-FDG PET/CT imaging was evaluated in this retrospective national multicenter study patients with metastatic posterior uveal melanoma (PUM) and compared to the largest diameter lesion (LDLM) American Joint Committee on Cancer (AJCC) staging system. Maximal Standard Uptake Value (SUV max ), Metabolic Tumor Volume (MTV), Total Lesion Glycolysis (TLG) were 106 patients. Higher values SUV ( p = 0.007, log-rank), MTV < 0.001, TLG long-rank) associated shorter survival. three also independent predictors multivariate Cox model, while AJCC turned insignificant. Time-dependent positive predictive (PPV) analysis Receiver Operating Characteristics (ROC) curves showed that (Area Under Curve (AUC) 0.78), (AUC LDLM 0.76) good 1-year For subset 97 liver metastases, corresponding regional measurements tended be even better predictors. In conclusion, found survival PUM than system, but when used as a continuous variable it an equally prediction
Language: Английский
Citations
0Cancers, Journal Year: 2025, Volume and Issue: 17(4), P. 611 - 611
Published: Feb. 11, 2025
Lung cancer remains the leading cause of cancer-related mortality, underscoring need for improved diagnostic methods. This study seeks to enhance classification accuracy confocal laser endomicroscopy (pCLE) images lung by applying a dual transfer learning (TL) approach that incorporates histological imaging data. Histological samples and pCLE images, collected from 40 patients undergoing curative surgeries, were selected create 2 balanced datasets (800 benign 800 malignant each). Three CNN architectures-AlexNet, GoogLeNet, ResNet-were pre-trained on ImageNet re-trained (confocal TL) or using TL (first then pCLE). Model performance was evaluated AUC across 50 independent runs with 10-fold cross-validation. The statistically significant outperformed TL, AlexNet achieving mean 94.97% an 0.98, surpassing GoogLeNet (91.43% accuracy, 0.97 AUC) ResNet (89.87% 0.96 AUC). All networks demonstrated (p < 0.001) improvements in TL. Additionally, models showed reductions both false positives negatives, class activation mappings highlighting enhanced focus diagnostically relevant regions. Dual integrating imaging, results improvement classification. offers promising framework tissue future development testing, iy has potential improve patient outcomes.
Language: Английский
Citations
0Computers in Biology and Medicine, Journal Year: 2025, Volume and Issue: 190, P. 110052 - 110052
Published: March 23, 2025
Language: Английский
Citations
0Research Square (Research Square), Journal Year: 2025, Volume and Issue: unknown
Published: April 15, 2025
Language: Английский
Citations
0