Traffic Classification in SDN-Based IoT Network using Two-Level Fused Network with Self-Adaptive Manta Ray Foraging
Abstract
The
rapid
expansion
of
IoT
networks,
combined
with
the
flexibility
Software-Defined
Networking
(SDN),
has
significantly
increased
complexity
traffic
management,
requiring
accurate
classification
to
ensure
optimal
quality
service
(QoS).
Existing
techniques
often
rely
on
manual
feature
selection,
limiting
adaptability
and
efficiency
in
dynamic
environments.
This
paper
presents
a
novel
framework
for
SDN-based
introducing
Two-Level
Fused
Network
integrated
self-adaptive
Manta
Ray
Foraging
Optimization
(SMRFO)
algorithm.
automatically
selects
features
fuses
multi-level
network
insights
enhance
accuracy.
is
classified
into
four
key
categories—delay-sensitive,
loss-sensitive,
bandwidth-sensitive,
best-effort—tailoring
QoS
meet
specific
requirements
each
class.
proposed
model
evaluated
using
publicly
available
datasets
(CIC-Darknet
ISCX-ToR),
achieving
superior
performance
over
99%
results
demonstrate
effectiveness
SMRFO
outperforming
state-of-the-art
methods,
providing
scalable
solution
management.
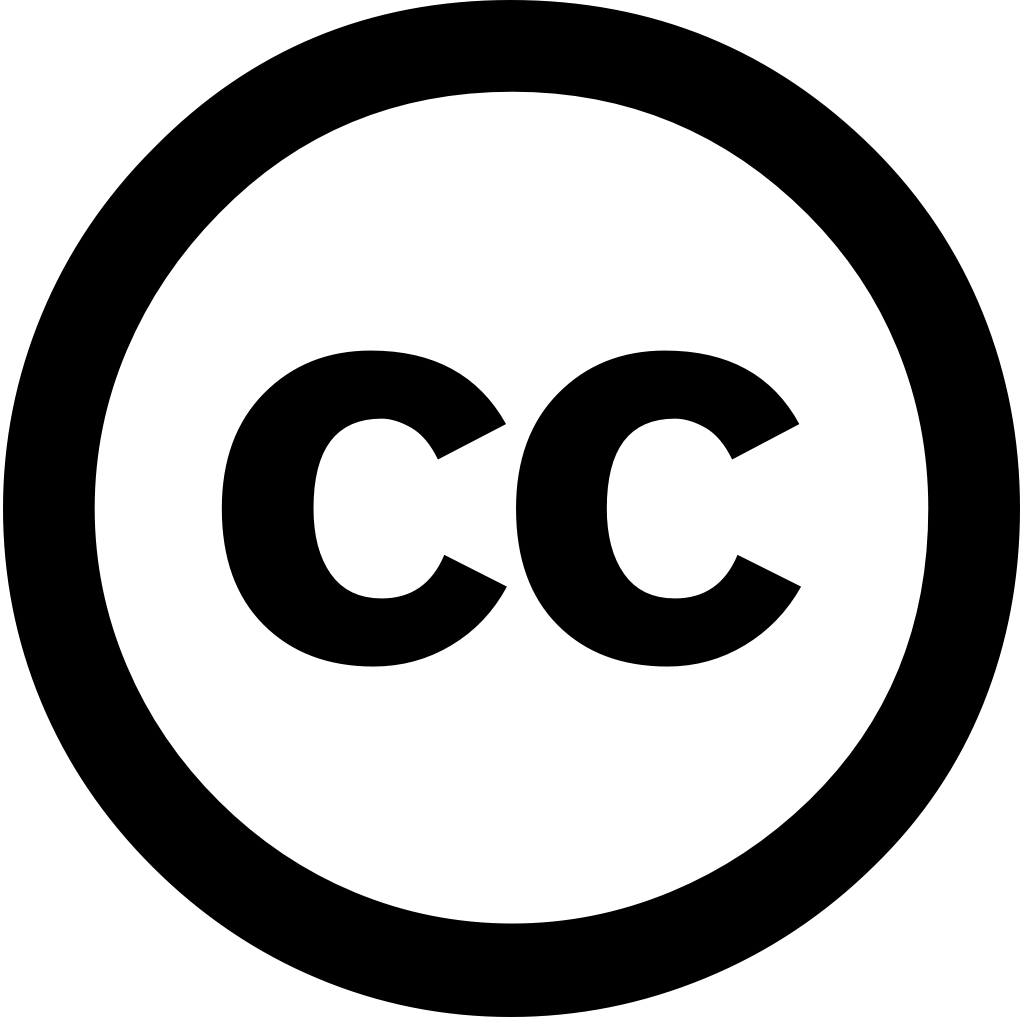
Research Square (Research Square), Journal Year: 2024, Volume and Issue: unknown
Published: Nov. 8, 2024
Language: Английский