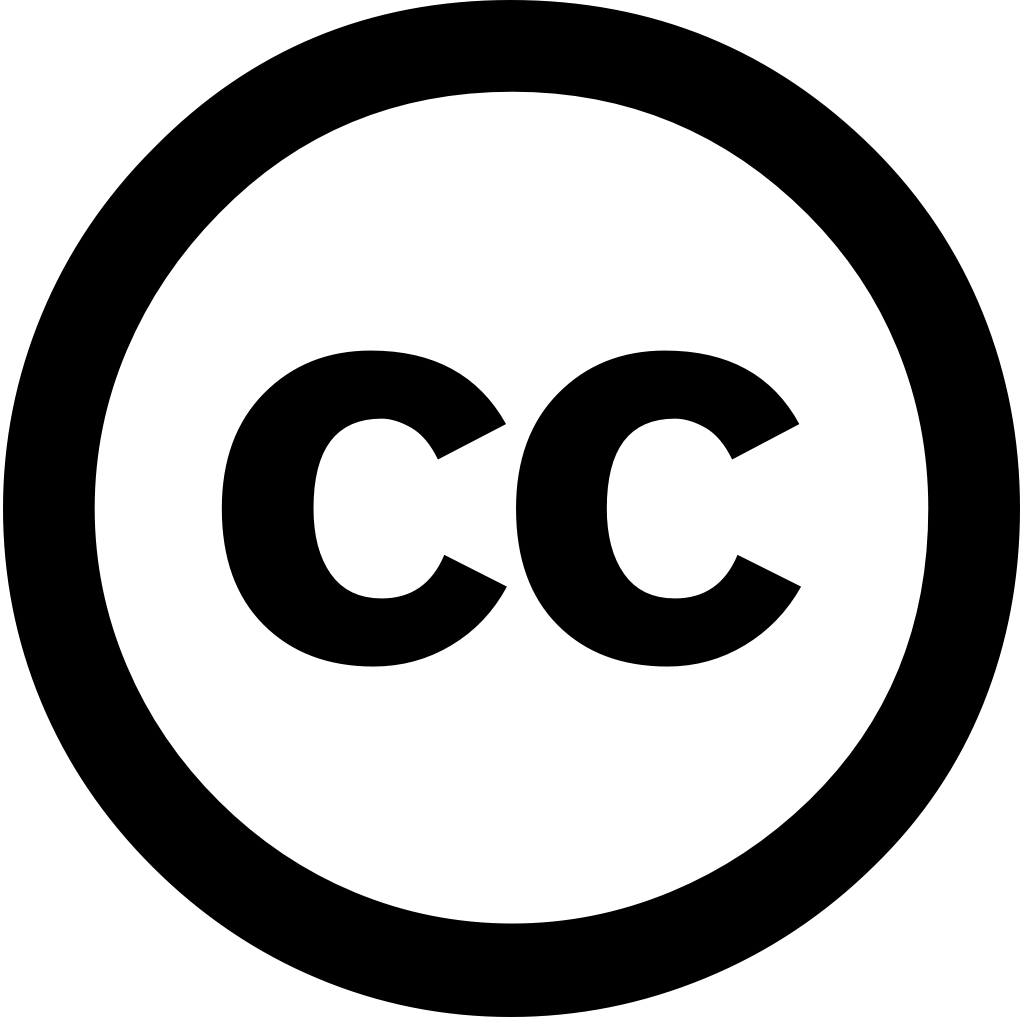
Applied Sciences, Journal Year: 2024, Volume and Issue: 14(24), P. 11545 - 11545
Published: Dec. 11, 2024
The growth of the Internet Things (IoT) and its integration with Industry 4.0 5.0 are generating new security challenges. One key elements IoT systems is effective anomaly detection, which identifies abnormal behavior in devices or entire systems. This paper presents a comprehensive overview existing methods for detection networks using machine learning (ML). A detailed analysis various ML algorithms, both supervised (e.g., Random Forest, Gradient Boosting, SVM) unsupervised Isolation Autoencoder), was conducted. results tests conducted on popular datasets (IoT-23 CICIoT-2023) were collected analyzed detail. performance selected algorithms evaluated commonly used metrics (Accuracy, Precision, Recall, F1-score). experimental showed that Forest Autoencoder highly detecting anomalies. article highlights importance appropriate data preprocessing to improve accuracy. Furthermore, limitations centralized approach context distributed discussed. also potential directions future research field IoT.
Language: Английский