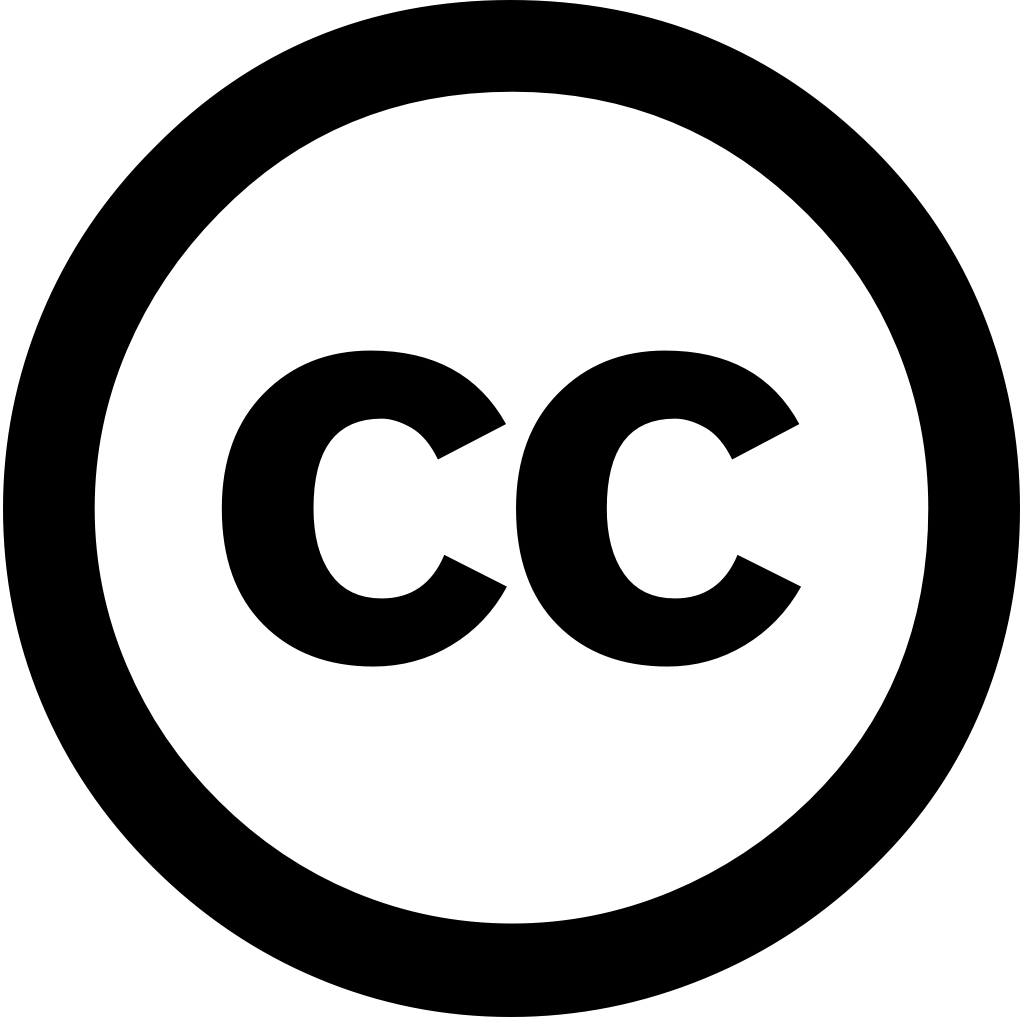
Systems, Journal Year: 2025, Volume and Issue: 13(4), P. 240 - 240
Published: March 31, 2025
The escalating frequency and complexity of natural disasters highlight the urgent need for deeper insights into how individuals communities perceive respond to risk information. Yet, conventional research methods—such as surveys, laboratory experiments, field observations—often struggle with limited sample sizes, external validity concerns, difficulties in controlling confounding variables. These constraints hinder our ability develop comprehensive models that capture dynamic, context-sensitive nature disaster decision-making. To address these challenges, we present a novel multi-stage simulation framework integrates Large Language Model (LLM)-driven social–cognitive agents well-established theoretical perspectives from psychology, sociology, decision science. This enables three critical phases—information perception, cognitive processing, decision-making—providing granular analysis demographic attributes, situational factors, social influences interact shape behavior under uncertain evolving conditions. A case study focusing on pre-disaster preventive measures demonstrates its effectiveness. By aligning agent demographics real-world survey data across 5864 simulated scenarios, reveal nuanced behavioral patterns closely mirroring human responses, underscoring potential overcome longstanding methodological limitations offer improved ecological flexibility explore diverse environments policy interventions. While acknowledging current constraints, such enhanced emotional modeling multimodal inputs, lays foundation more nuanced, empirically grounded analyses perception response patterns. seamlessly blending theory, advanced LLM capabilities, empirical alignment strategies, this not only advances state computational but also provides valuable guidance developing targeted management strategies.
Language: Английский