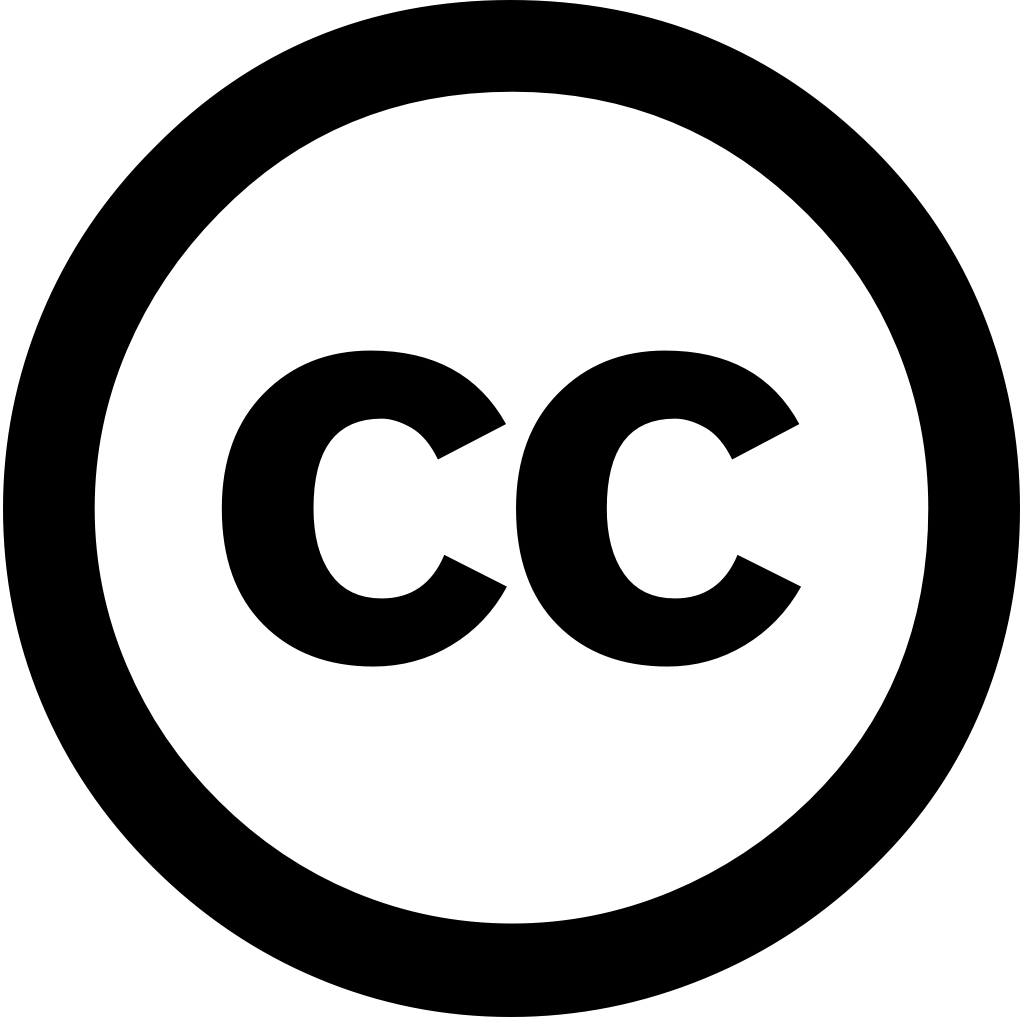
PLoS ONE, Journal Year: 2024, Volume and Issue: 19(8), P. e0308789 - e0308789
Published: Aug. 28, 2024
Addressing the challenges in effectively extracting multi-scale features and preserving pose information during hyperspectral image (HSI) classification, a Multi-Scale Depthwise Separable Capsule Network (MDSC-Net) is proposed this article for HSI classification. Initially, hierarchical are extracted by MDSC-Net through employment of parallel convolutional kernels, while computational complexity reduced via depthwise separable convolutions, thus reducing overall load achieving efficient feature extraction. Subsequently, to enhance translational invariance reduce loss information, various scales processed independent capsule networks, with improvements max pooling achieved dynamic routing. Lastly, different concatenated integrated concatenate operation, thereby facilitating precise analysis multi-level classification process. Experimental comparisons demonstrate that achieves average accuracies 94%, 98%, 99% on Kennedy Space Center, University Pavia, Salinas datasets, respectively, indicating significant performance advantage over recent models validating effectiveness model.
Language: Английский