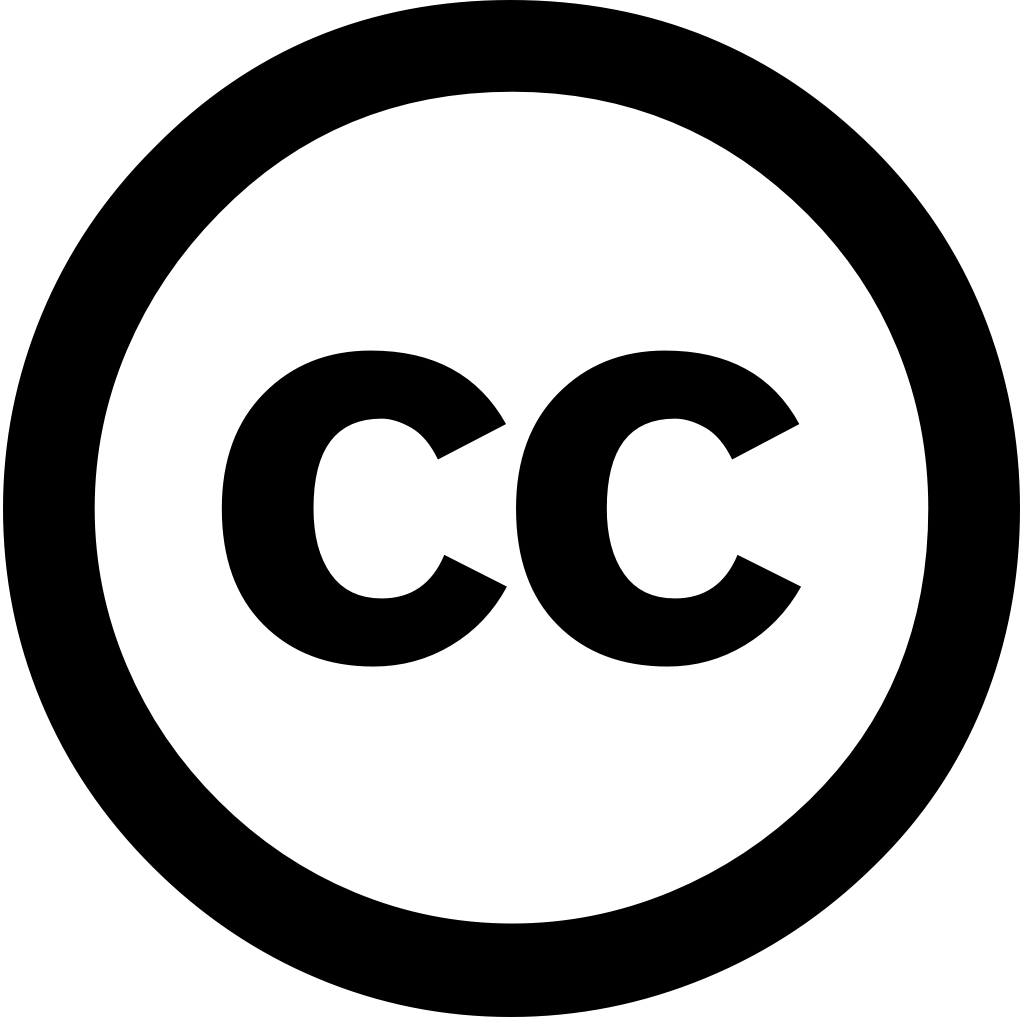
IEEE Access, Journal Year: 2024, Volume and Issue: 12, P. 165903 - 165917
Published: Jan. 1, 2024
Language: Английский
IEEE Access, Journal Year: 2024, Volume and Issue: 12, P. 165903 - 165917
Published: Jan. 1, 2024
Language: Английский
Emirates Journal of Food and Agriculture, Journal Year: 2024, Volume and Issue: 36, P. 1 - 9
Published: April 18, 2024
The impact of deep learning (DL) is substantial across numerous domains, particularly in agriculture. Within this context, our study focuses on the classification problematic soybean seeds. dataset employed encompasses five distinct classes, totaling 5513 images. Our model, based InceptionV3 architecture, undergoes modification with addition supplementary layers to enhance efficiency and performance. Techniques such as transfer learning, adaptive rate adjustment (to 0.001), model checkpointing are integrated optimize accuracy. During initial evaluation, achieved 88.07% accuracy training 86.67% validation. Subsequent implementation tuning strategies significantly improves Augmenting architecture additional layers, including Average Pooling, Flatten, Dense, Dropout, Softmax, plays a pivotal role enhancing Evaluation metrics, precision, recall, F1-score, underscore model’s effectiveness. Precision ranges from 0.9706 1.0000, while recall values demonstrate high capture all classes. reflecting balance between precision exhibits remarkable performance ranging 0.9851 1.0000. Comparative analysis existing studies reveals competitive 98.73% by proposed model. While variations exist specific purposes datasets among studies, showcases promising seed classification, contributing advancements agricultural technology for crop health assessment management.
Language: Английский
Citations
20Frontiers in Computer Science, Journal Year: 2024, Volume and Issue: 6
Published: Sept. 11, 2024
Background The occurrence of diseases in rice leaves presents a substantial challenge to farmers on global scale, hence jeopardizing the food security an expanding population. timely identification and prevention these are utmost importance order mitigate their impact. Methods present study conducts comprehensive evaluation contemporary literature pertaining diseases, covering period from 2008 2023. process selecting pertinent studies followed guidelines outlined by Kitchenham, which ultimately led inclusion 69 for purpose review. It is worth mentioning that significant portion research endeavours have been directed towards studying such as brown spot, blast, bacterial blight. primary performance parameter emerged was accuracy. Researchers strongly advocated combination hybrid deep learning machine methodologies improve rates recognition leaf diseases. Results collection scholarly investigations focused detection characterization affecting leaves, with specific emphasis prominence accuracy measure highlights precision diagnosis Furthermore, efficacy employing combine techniques exemplified enhancing capacities leaves. Conclusion This systematic review provides insight into conducted scholars field disease during previous decade. text underscores significance calls implementation augment identification, presenting possible resolutions obstacles presented agricultural hazards.
Language: Английский
Citations
6Journal of Algorithms & Computational Technology, Journal Year: 2025, Volume and Issue: 19
Published: Jan. 1, 2025
This study introduces significant improvements in the construction of deep convolutional neural network models for classifying agricultural products, specifically oranges, based on their shape, size, and color. Utilizing MobileNetV2 architecture, this research leverages its efficiency lightweight nature, making it suitable mobile embedded applications. Key techniques such as depthwise separable convolutions, linear bottlenecks, inverted residuals help reduce number parameters computational load while maintaining high performance feature extraction. Additionally, employs comprehensive data augmentation methods, including horizontal vertical flips, grayscale transformations, hue adjustments, brightness noise addition to enhance model's robustness generalization capabilities. The proposed model demonstrates superior performance, achieving an overall accuracy 99.53%∼100% with nearly perfect precision, recall 95.7%, F1-score 94.6% both “orange_good” “orange_bad” classes, significantly outperforming previous which typically achieved accuracies between 70% 90%. While classification was near-perfect some aspects, there were minor errors specific detection tasks. confusion matrix shows that has sensitivity specificity, very few misclassifications. Finally, highlights practical applicability model, particularly easy deployment resource-constrained devices effectiveness product quality control processes. These findings affirm a reliable highly efficient tool classification, surpassing capabilities traditional field.
Language: Английский
Citations
0Journal of Imaging, Journal Year: 2025, Volume and Issue: 11(2), P. 32 - 32
Published: Jan. 24, 2025
This research study utilized artificial intelligence (AI) to detect natural disasters from aerial images. Flooding and desertification were two taken into consideration. The Climate Change Dataset was created by compiling various open-access data sources. dataset contains 6334 images UAV (unmanned vehicles) satellite then used train Deep Learning (DL) models identify disasters. Four different Machine (ML) used: convolutional neural network (CNN), DenseNet201, VGG16, ResNet50. These ML trained on our so that their performance could be compared. DenseNet201 chosen for optimization. All four performed well. ResNet50 achieved the highest testing accuracies of 99.37% 99.21%, respectively. project demonstrates potential AI address environmental challenges, such as climate change-related study’s approach is novel creating a new dataset, optimizing an model, cross-validating, presenting one DL detection. Three categories (Flooded, Desert, Neither). Our relates Environmental Sustainability. Drone emergency response would practical application project.
Language: Английский
Citations
0Lecture notes in networks and systems, Journal Year: 2025, Volume and Issue: unknown, P. 109 - 121
Published: Jan. 1, 2025
Language: Английский
Citations
0Current Plant Biology, Journal Year: 2025, Volume and Issue: unknown, P. 100459 - 100459
Published: Feb. 1, 2025
Language: Английский
Citations
0Journal of Food Science, Journal Year: 2025, Volume and Issue: 90(3)
Published: March 1, 2025
Abstract Using on‐site images to classify and identify wild mushroom species is the most effective way prevent incidents of harm caused by eating mushrooms. However, complexity natural scenes similarity morphology bring challenges for accurate classification recognition. To this end, paper proposes an improved ConvNeXt V2 network model recognition mushrooms in complex similar appearances. First, study applies data enhancement techniques such as image flipping, adding noise mosaic solve problem dataset equalization, constructs a containing 18 categories number 10,986 images. Second, cross‐modular approach used extract fuse features different dimensions enhance feature capture capability model. In addition, optimized one‐hot coding spatial pyramid pooling techniques. The experimental results show that outperforms comparative models ResNet, MobileVit, Swin Transformer, ConvNeXt, terms accuracy, precision, recall, F1‐Score, which are 96.7%, 96.84%, 96.83%, 96.84%. ablation experiments further verify effectiveness superiority proposed improvement strategy enhancing performance, can effectively improve efficiency accuracy Practical Application : be identification edible nonedible mushroom, it provide technical support reduce incidence poisoning ensure food safety.
Language: Английский
Citations
0Elsevier eBooks, Journal Year: 2025, Volume and Issue: unknown, P. 279 - 289
Published: Jan. 1, 2025
Language: Английский
Citations
0Frontiers in Artificial Intelligence, Journal Year: 2025, Volume and Issue: 8
Published: March 26, 2025
Maize, a globally essential staple crop, suffers significant yield losses due to diseases. Traditional diagnostic methods are often inefficient and subjective, posing challenges for timely accurate pest management. This study introduces MoSViT, an innovative classification model leveraging advanced machine learning computer vision technologies. Built on the MobileViT V2 framework, MoSViT integrates CLA focus mechanism, DRB module, Block, LeakyRelu6 activation function enhance feature extraction accuracy while reducing computational complexity. Trained dataset of 3,850 images encompassing Blight, Common Rust, Gray Leaf Spot, Healthy conditions, achieves exceptional performance, with accuracy, Precision, Recall, F1 Score 98.75%, 98.73%, 98.72%, respectively. These results surpass leading models such as Swin Transformer V2, DenseNet121, EfficientNet in both parameter efficiency. Additionally, model's interpretability is enhanced through heatmap analysis, providing insights into its decision-making process. Testing small sample datasets further demonstrates MoSViT's generalization capability potential small-sample detection scenarios.
Language: Английский
Citations
0Smart Agricultural Technology, Journal Year: 2025, Volume and Issue: unknown, P. 100934 - 100934
Published: April 1, 2025
Language: Английский
Citations
0