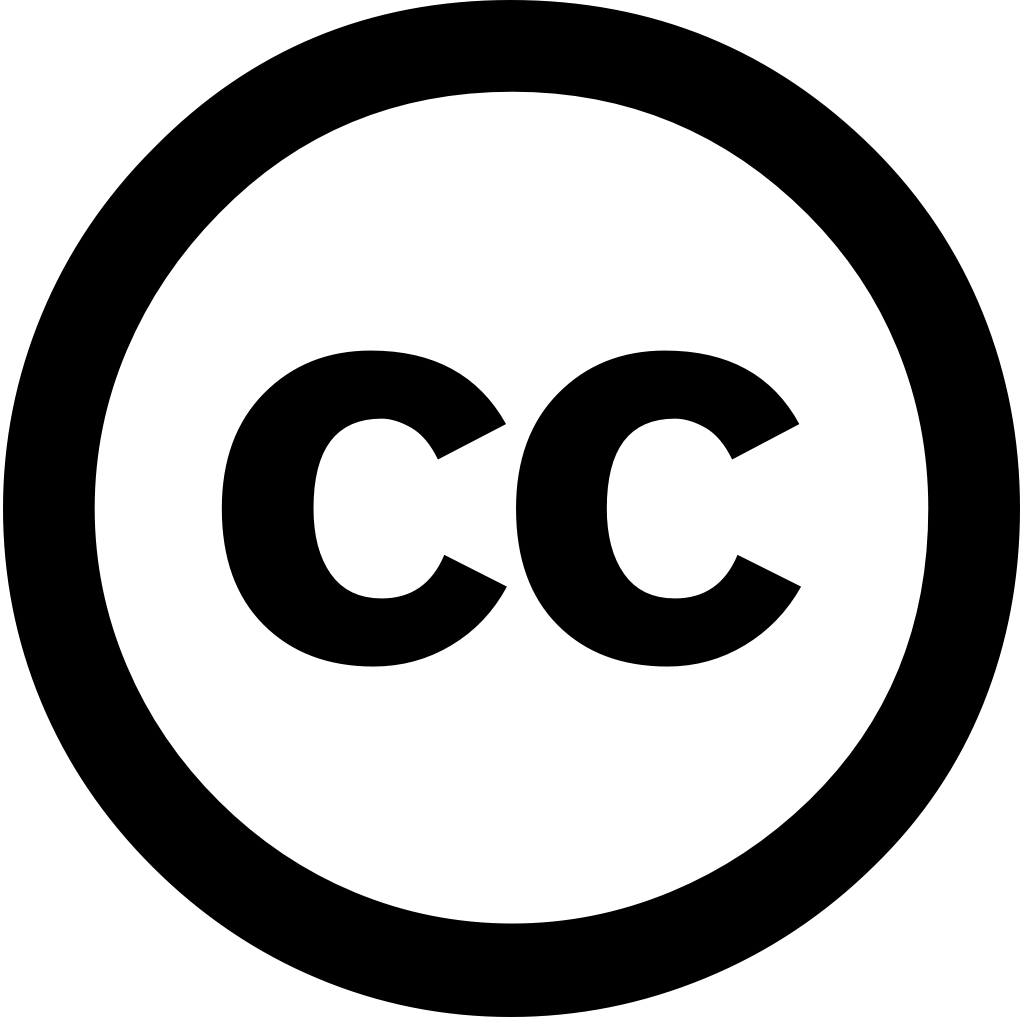
IEEE Access, Journal Year: 2024, Volume and Issue: 12, P. 165903 - 165917
Published: Jan. 1, 2024
Language: Английский
IEEE Access, Journal Year: 2024, Volume and Issue: 12, P. 165903 - 165917
Published: Jan. 1, 2024
Language: Английский
IEEE Access, Journal Year: 2024, Volume and Issue: 12, P. 169518 - 169532
Published: Jan. 1, 2024
Language: Английский
Citations
1Agronomy, Journal Year: 2024, Volume and Issue: 14(6), P. 1206 - 1206
Published: June 3, 2024
Agriculture is an area that currently benefits from the use of new technologies and techniques, such as artificial intelligence, to improve production in crop fields. Zacatecas one states producing most onions northeast region Mexico. Identifying determining vegetation, soil, humidity zones could help solve problems irrigation demands or excesses, identify spaces with different levels soil homogeneity, estimate yield health crop. This study examines application intelligence through deep learning, specifically convolutional neural networks, patterns can be found a field, this case, zones. To extract mentioned patterns, K-nearest neighbor algorithm was used pre-process images taken using unmanned aerial vehicles form dataset composed 3672 (1224 for each class). A total six network models were classify namely Alexnet, DenseNet, VGG16, SqueezeNet, MobileNetV2, Res-Net18. Each model evaluated following validation metrics: accuracy, F1-score, precision, recall. The results showed variation performance between 90% almost 100%. Alexnet obtained highest metrics accuracy 99.92%, while MobileNetV2 had lowest 90.85%. Other models, ResNet18, 92.02% 98.78%. Furthermore, our highlights importance adopting agriculture, particularly management onion fields Zacatecas, findings farmers agronomists make more informed efficient decisions, which lead greater sustainability local agriculture.
Language: Английский
Citations
0Applied Sciences, Journal Year: 2024, Volume and Issue: 14(13), P. 5894 - 5894
Published: July 5, 2024
As living standards have improved, consumer demand for high-quality dried abalone has increased. Traditional grading is achieved through slice analysis (sampling analysis) combined with human experience. However, this method several issues, including non-uniform standards, low detection accuracy, inconsistency between internal and external quality, high loss rate. Therefore, we propose a deep-learning-aided approach leveraging X-ray images that can achieve efficient non-destructive quality of abalone. To the best our knowledge, first work to use image structure The was divided into three phases. First, database constructed, containing 644 samples, relationship analyzed. Second, augmented by rotation, mirroring, noise superposition. Subsequently, model selection evaluation process carried out. results showed that, in comparison models such as VGG-16, MobileNet (Version 1.0), AlexNet, Xception, VGG-19 demonstrated performance Finally, modified network based on CBAM proposed classify show effective, achieving score 95.14%, outperformed competitors, i.e., alone squeeze-and-excitation block (SE) attention mechanism.
Language: Английский
Citations
0Research Square (Research Square), Journal Year: 2024, Volume and Issue: unknown
Published: July 19, 2024
Language: Английский
Citations
0IEEE Access, Journal Year: 2024, Volume and Issue: 12, P. 165903 - 165917
Published: Jan. 1, 2024
Language: Английский
Citations
0