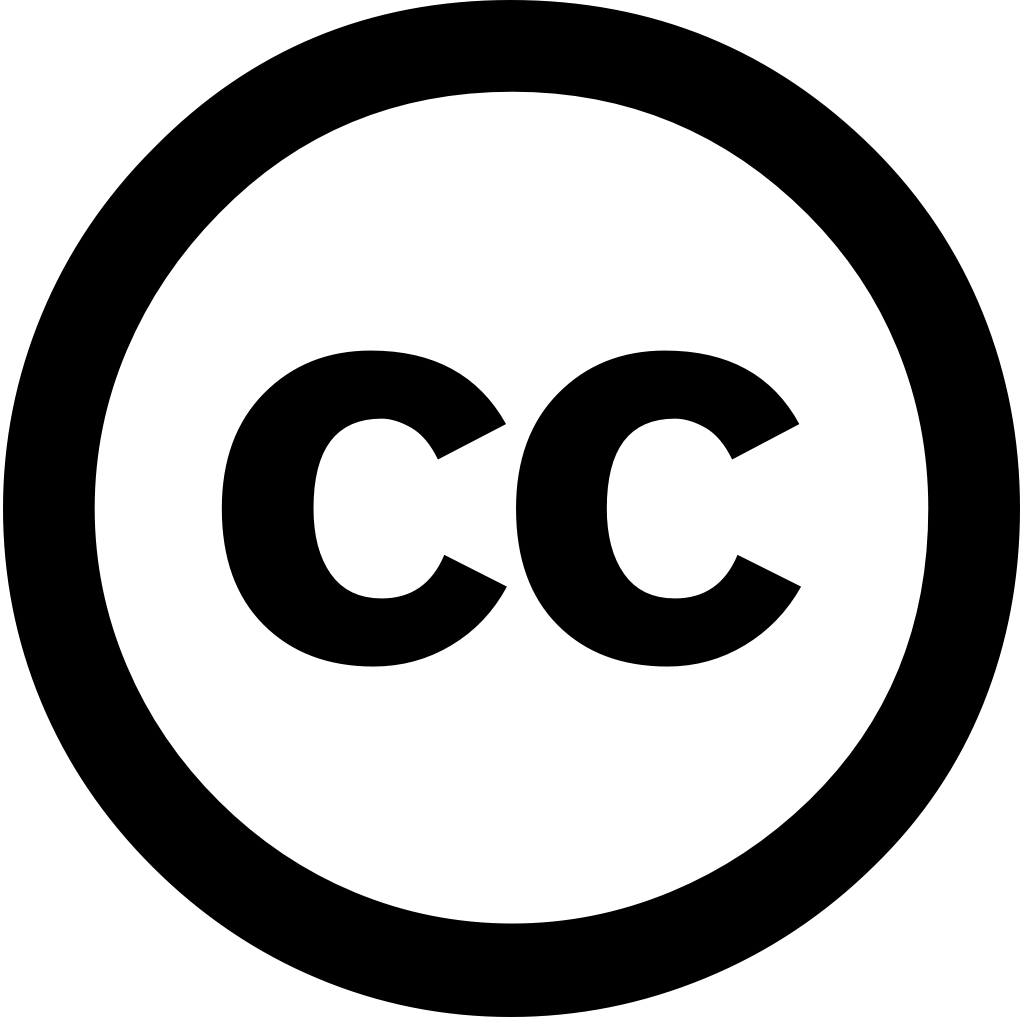
Journal of Medical Internet Research, Journal Year: 2025, Volume and Issue: unknown
Published: Jan. 13, 2025
Language: Английский
Journal of Medical Internet Research, Journal Year: 2025, Volume and Issue: unknown
Published: Jan. 13, 2025
Language: Английский
Diagnostics, Journal Year: 2023, Volume and Issue: 13(17), P. 2760 - 2760
Published: Aug. 25, 2023
This comprehensive review unfolds a detailed narrative of Artificial Intelligence (AI) making its foray into radiology, move that is catalysing transformational shifts in the healthcare landscape. It traces evolution from initial discovery X-rays to application machine learning and deep modern medical image analysis. The primary focus this shed light on AI applications elucidating their seminal roles segmentation, computer-aided diagnosis, predictive analytics, workflow optimisation. A spotlight cast profound impact diagnostic processes, personalised medicine, clinical workflows, with empirical evidence derived series case studies across multiple disciplines. However, integration radiology not devoid challenges. ventures labyrinth obstacles are inherent AI-driven radiology—data quality, ’black box’ enigma, infrastructural technical complexities, as well ethical implications. Peering future, contends road ahead for paved promising opportunities. advocates continuous research, embracing avant-garde imaging technologies, fostering robust collaborations between radiologists developers. conclusion underlines role catalyst change stance firmly rooted sustained innovation, dynamic partnerships, steadfast commitment responsibility.
Language: Английский
Citations
311Cureus, Journal Year: 2023, Volume and Issue: unknown
Published: Feb. 20, 2023
Background Artificial intelligence (AI) is evolving for healthcare services. Higher cognitive thinking in AI refers to the ability of system perform advanced processes, such as problem-solving, decision-making, reasoning, and perception. This type goes beyond simple data processing involves understand manipulate abstract concepts, interpret, use information a contextually relevant way, generate new insights based on past experiences accumulated knowledge. Natural language models like ChatGPT conversational program that can interact with humans provide answers queries. Objective We aimed ascertain capability solving higher-order reasoning subject pathology. Methods cross-sectional study was conducted internet using an AI-based chat provides free service research purposes. The current version (January 30 version) used converse total 100 These questions were randomly selected from question bank institution categorized according different systems. responses each collected stored further analysis. evaluated by three expert pathologists zero five scale into structure observed learning outcome (SOLO) taxonomy categories. score compared one-sample median test hypothetical values find its accuracy. Result A solved average 45.31±7.14 seconds answer. overall 4.08 (Q1-Q3: 4-4.33) which below maximum value (one-test p <0.0001) similar four = 0.14). majority (86%) "relational" category SOLO taxonomy. There no difference scores asked various organ systems Pathology (Kruskal Wallis 0.55). rated had excellent level inter-rater reliability (ICC 0.975 [95% CI: 0.965-0.983]; F 40.26; < 0.0001). Conclusion solve pathology relational Hence, text output connections among parts meaningful response. approximately 80%. academicians or students get help reasoning-type also. As evolving, studies are needed accuracy any versions.
Language: Английский
Citations
165Published: June 16, 2023
This comprehensive review unfolds a detailed narrative of Artificial Intelligence (AI) making its foray into radiology, move that is catalysing transformational shifts in the healthcare landscape. It sheds light on journey from pioneering discovery X-rays to today’s intricate imaging technologies, infused with machine learning and deep medical image analysis. At crux this lies an in-depth study AI applications elucidating seminal roles segmentation, computer-aided diagnosis, predictive analytics, workflow optimisation. A spotlight cast profound impact diagnostic processes, personalised medicine, clinical workflows, empirical evidence derived series case studies across multiple disciplines. However, integration radiology not devoid challenges. The ventures labyrinth obstacles are inherent AI-driven — data quality, ’black box’ enigma, infrastructural technical complexities, as well ethical implications. Peering future, contends road ahead for paved promising opportunities. advocates continuous research, embracing avant-garde fostering robust collaborations between radiologists developers. concludes by firmly cementing role catalyst change stance rooted sustained innovation, dynamic partnerships, steadfast commitment responsibility.
Language: Английский
Citations
52Published: Jan. 1, 2025
Language: Английский
Citations
2BMC Medical Informatics and Decision Making, Journal Year: 2025, Volume and Issue: 25(1)
Published: Feb. 5, 2025
Abstract Background Artificial intelligence (AI)-based systems are being rapidly integrated into the fields of health and social care. Although such can substantially improve provision care, diverse marginalized populations often incorrectly or insufficiently represented within these systems. This review aims to assess influence AI on care among populations, particularly with regard issues related inclusivity regulatory concerns. Methods We followed Preferred Reporting Items for Systematic Reviews Meta-Analyses guidelines. Six leading databases were searched, 129 articles selected this in line predefined eligibility criteria. Results research revealed disparities outcomes, accessibility, representation groups due biased data sources a lack training datasets, which potentially exacerbate inequalities delivery communities. Conclusion development practices, legal frameworks, policies must be reformulated ensure that is applied an equitable manner. A holistic approach used address disparities, enforce effective regulations, safeguard privacy, promote inclusion equity, emphasize rigorous validation.
Language: Английский
Citations
2Lecture notes in computer science, Journal Year: 2023, Volume and Issue: unknown, P. 313 - 326
Published: Jan. 1, 2023
Language: Английский
Citations
34BJR|Open, Journal Year: 2023, Volume and Issue: 5(1)
Published: July 3, 2023
Artificial intelligence (AI) has transitioned from the lab to bedside, and it is increasingly being used in healthcare. Radiology Radiography are on frontline of AI implementation, because use big data for medical imaging diagnosis different patient groups. Safe effective implementation requires that responsible ethical practices upheld by all key stakeholders, there harmonious collaboration between professional groups, customised educational provisions involved. This paper outlines principles AI, highlights recent initiatives clinical practitioners discusses synergies professionals as they prepare digital future Europe. Responsible vital enhance a culture safety trust healthcare patients alike. Educational training central understanding basic applications many offerings currently Education can facilitate transparency tools, but more formalised, university-led needed ensure academic scrutiny, appropriate pedagogy, multidisciplinarity customisation learners' unique needs adhered to. As radiographers radiologists work together with other understand harness benefits imaging, becomes clear faced same challenges have needs. The belongs multidisciplinary teams seamlessly together, learn manage risk collectively collaborate benefit serve.
Language: Английский
Citations
24Journal of Medical Imaging and Radiation Oncology, Journal Year: 2024, Volume and Issue: 68(3), P. 257 - 264
Published: Jan. 19, 2024
Summary This study aimed to comprehensively evaluate the current utilization and future potential of ChatGPT, an AI‐based chat model, in field radiology. The primary focus is on its role enhancing decision‐making processes, optimizing workflow efficiency, fostering interdisciplinary collaboration teaching within healthcare. A systematic search was conducted PubMed, EMBASE Web Science databases. Key aspects, such as impact complex decision‐making, enhancement collaboration, were assessed. Limitations challenges associated with ChatGPT implementation also examined. Overall, six studies met inclusion criteria included our analysis. All prospective nature. total 551 chatGPT (version 3.0 4.0) assessment events Considering generation academic papers, found output data inaccuracies 80% time. When asked questions regarding common interventional radiology procedures, it contained entirely incorrect information 45% seen better answer US board‐style when lower order thinking required ( P = 0.002). Improvements between 3.5 4.0 regard imaging accuracy rates 61 versus 85%( 0.009). observed have average translational ability score 4.27/5 Likert scale CT MRI findings. demonstrates substantial augment workflow. While ChatGPT's promise evident, thorough evaluation validation are imperative before widespread adoption
Language: Английский
Citations
13Medicine, Journal Year: 2024, Volume and Issue: 103(27), P. e38811 - e38811
Published: July 5, 2024
The application of artificial intelligence (AI) technologies in scientific research has significantly enhanced efficiency and accuracy but also introduced new forms academic misconduct, such as data fabrication text plagiarism using AI algorithms. These practices jeopardize integrity can mislead directions. This study addresses these challenges, underscoring the need for community to strengthen ethical norms, enhance researcher qualifications, establish rigorous review mechanisms. To ensure responsible transparent processes, we recommend following specific key actions: Development enforcement comprehensive guidelines that include clear protocols use analysis publication, ensuring transparency accountability AI-assisted research. Implementation mandatory ethics training researchers, aimed at fostering an in-depth understanding potential misuses promoting practices. Establishment international collaboration frameworks facilitate exchange best development unified standards Protecting is paramount maintaining public trust science, making recommendations urgent consideration action.
Language: Английский
Citations
13Insights into Imaging, Journal Year: 2024, Volume and Issue: 15(1)
Published: Feb. 5, 2024
Abstract Objective To provide a comprehensive framework for value assessment of artificial intelligence (AI) in radiology. Methods This paper presents the RADAR framework, which has been adapted from Fryback and Thornbury’s imaging efficacy to facilitate valuation radiology AI conception local implementation. Local newly introduced underscore importance appraising an technology within its environment. Furthermore, is illustrated through myriad study designs that help assess value. Results seven-level hierarchy, providing radiologists, researchers, policymakers with structured approach AI. designed be dynamic meet different needs throughout AI’s lifecycle. Initial phases like technical diagnostic (RADAR-1 RADAR-2) are assessed pre-clinical deployment via silico clinical trials cross-sectional studies. Subsequent stages, spanning thinking patient outcome (RADAR-3 RADAR-5), require integration explored randomized controlled cohort Cost-effectiveness (RADAR-6) takes societal perspective on financial feasibility, addressed health-economic evaluations. The final level, RADAR-7, determines how prior valuations translate locally, evaluated budget impact analysis, multi-criteria decision analyses, prospective monitoring. Conclusion offers valuing Its layered, hierarchical structure, combined focus relevance, aligns seamlessly principles value-based Critical relevance statement advances by delineating much-needed valuation. Keypoints • Radiology lacks assessment. provides dynamic, method thorough bridging implementation gap.
Language: Английский
Citations
10