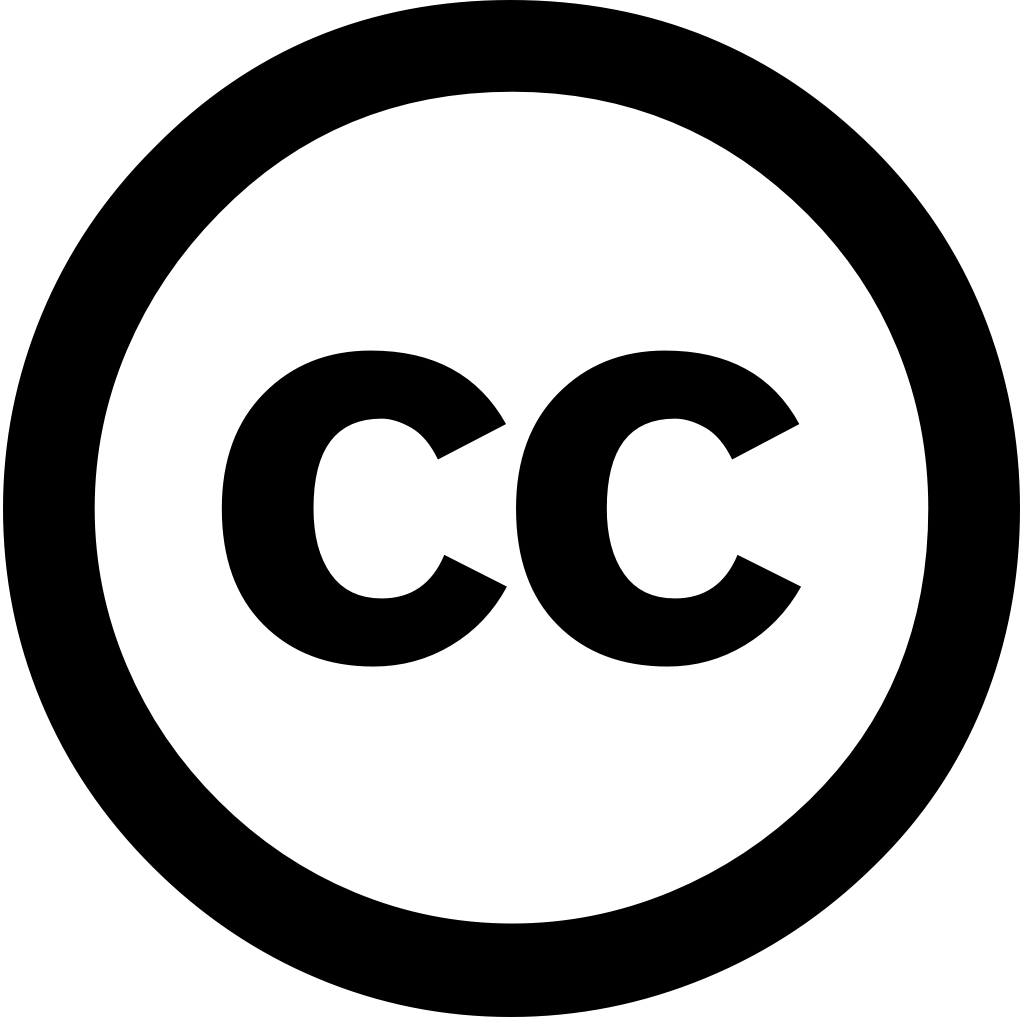
Journal of Rock Mechanics and Geotechnical Engineering, Journal Year: 2024, Volume and Issue: unknown
Published: Sept. 1, 2024
Language: Английский
Journal of Rock Mechanics and Geotechnical Engineering, Journal Year: 2024, Volume and Issue: unknown
Published: Sept. 1, 2024
Language: Английский
Computers & Geosciences, Journal Year: 2023, Volume and Issue: 181, P. 105466 - 105466
Published: Sept. 27, 2023
Language: Английский
Citations
3Deleted Journal, Journal Year: 2024, Volume and Issue: 6(6)
Published: June 12, 2024
Abstract Automatic and fast rock classification identification is an important part of geotechnical intelligent survey system. Image based supervised deep learning analysis, especially for convolutional neural networks (CNN), has potential in optimizing lithologic interpretation using borehole core images. However, the accuracy efficiency lithology models are low at present. In this work, a systematic enormous data framework on geological system firstly established to provide datasets. The dataset composed approximately 150,000 images samples, which covers igneous rocks, sedimentary metamorphic rocks. Secondly, CNN-deep transfer algorithm, end-to-end, image-to-label established. Finally, generalization proposed model field drilling verification test show that constructed recognition ability identify rocks quickly accurately, 12 kinds common engineering more than 95%. provides convenient tool geologists scientific researchers.
Language: Английский
Citations
0Linköping electronic conference proceedings, Journal Year: 2024, Volume and Issue: 208, P. 188 - 194
Published: June 14, 2024
We explore the use of various machine learning (ML) models for classifying lithologies utilizing data from X-ray fluorescence (XRF) and computed tomography (XCT). Typically, are identified over several meters, which restricts ML due to limited training data. To address this issue, we augment original interval dataset, where marked extensive sections, into finer segments 10cm, produce a high resolution dataset with vastly increased sample size. Additionally, examine impact adjacent on building more generalized model. also demonstrate that combining XRF XCT leads an improved classification accuracy compared using only data, is common practice in current studies, or solely relying
Language: Английский
Citations
0Petroleum Science, Journal Year: 2024, Volume and Issue: unknown
Published: July 1, 2024
It is of great significance to accurately and rapidly identify shale lithofacies in relation the evaluation prediction sweet spots for oil gas reservoirs. To address problem low resolution logging curves, this study establishes a grayscale-phase model based on high-resolution grayscale curves using clustering analysis algorithms identification, working with Shahejie Formation, Bohai Bay Basin, China. The phase defined as sum absolute relative amplitude well their features. magnitude gray values utilized evaluating material composition (mineral + total organic carbon) shale, while difference between adjacent used structure type. research results show that can well, accuracy applicability were verified by fitting relationship mineral composition, corresponding relationships amplitudes laminae development shales. Four are identified target layer area: massive mixed laminated calcareous shale. This method not only effectively characterize but also numerically degree laminae, solve difficult millimeter-scale which provide technical support spot complex continental lacustrine basins.
Language: Английский
Citations
0Journal of Rock Mechanics and Geotechnical Engineering, Journal Year: 2024, Volume and Issue: unknown
Published: Sept. 1, 2024
Language: Английский
Citations
0