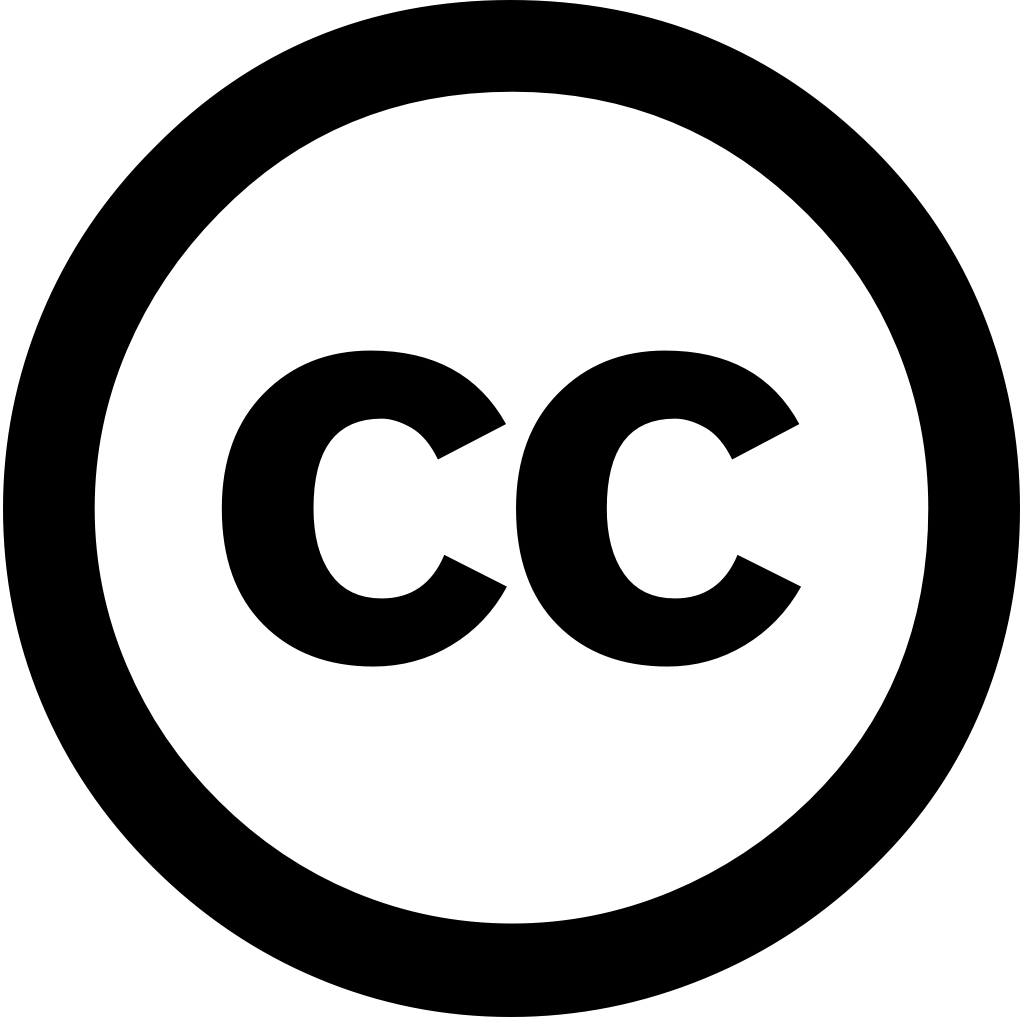
Aquacultural Engineering, Journal Year: 2024, Volume and Issue: unknown, P. 102466 - 102466
Published: Sept. 1, 2024
Language: Английский
Aquacultural Engineering, Journal Year: 2024, Volume and Issue: unknown, P. 102466 - 102466
Published: Sept. 1, 2024
Language: Английский
Ecological Informatics, Journal Year: 2025, Volume and Issue: unknown, P. 102989 - 102989
Published: Jan. 1, 2025
Language: Английский
Citations
1Geomorphology, Journal Year: 2024, Volume and Issue: 455, P. 109185 - 109185
Published: April 4, 2024
Field surveys to collect data from fluvial ecosystems traditionally focus on specific phenomena related geomorphology or hydrology. Low-cost unmanned aerial vehicles (UAVs) additionally empower the fast and massive collection of airborne photogrammetry, providing geospatially explicit information. This remote sensing complements field by offering contextual information geomorphological conditions, including digital terrain models. AI-based image recognition can augment extrapolate archetypal object classes through name labels, such as "gravel", "sand", "plant", "large wood". However, obtaining sufficient ground truth for these classifications, particularly in morphodynamic environments, is challenging induces high costs. study introduces a transfer learning approach address challenge low availability, enabling mapping complex objects landscapes. We leverage learned general structure deep convolutional neural network (CNN) pre-trained broad range images. The fixed latent features CNN stem GoogLeNet. A feature extractor serves classify with limited amounts. Satisfactory performance measured recall rate, expressing ability model find all occurrences class an image. High spatial heterogeneity locations measurements x-y plane improves performance. With minimum 400 labeled instances, achieves satisfactory 93.75-% wood" target class, evidence effectiveness studies. detect large woods river environments critical restoration efforts it helps create fish habitat, which essential supporting biodiversity.
Language: Английский
Citations
5Ecological Informatics, Journal Year: 2025, Volume and Issue: unknown, P. 103146 - 103146
Published: April 1, 2025
Language: Английский
Citations
0Frontiers in Marine Science, Journal Year: 2024, Volume and Issue: 11
Published: March 15, 2024
OPINION article Front. Mar. Sci., 15 March 2024Sec. Ocean Observation Volume 11 - 2024 | https://doi.org/10.3389/fmars.2024.1349705
Language: Английский
Citations
3Journal of Biomedical Science, Journal Year: 2024, Volume and Issue: 31(1)
Published: Aug. 23, 2024
Abstract Background Identification of lung cancer subtypes is critical for successful treatment in patients, especially those advanced stages. Many and personal treatments require knowledge specific mutations, as well up- down-regulations genes, effective targeting the cells. While many studies focus on individual cell structures delve deeper into gene sequencing, present study proposes a machine learning method classification based low-magnification outgrowth patterns 2D co-culture environment. Methods Using magnetic plate holder, circular pattern clusters were generated among fibroblasts, daily images captured to monitor over 9-day period. These then augmented used train convolutional neural network (CNN) model lightweight TinyVGG architecture. The was trained with pairs classes representing three NSCLC: A549 (adenocarcinoma), H520 (squamous carcinoma), H460 (large carcinoma). objective assess whether this could accurately classify lines at different stages outgrowth. Additionally, two patient-derived cells, one KRAS oncogene other EGFR oncogene, classified using CNN model. This demonstration aimed investigate translational potential learning-enabled classification. Results achieved 93% accuracy 1 day A549, H460, H520, reached 100% 7 days 4 Although these cells are Adenocarcinoma, their vary depending expressions (KRAS or EGFR). Conclusions results demonstrate that architecture, operating locally laptop without cloud connectivity, can effectively create capable classifying subtypes, including derived from upon presence surrounding fibroblasts. advancement underscores enhance early subtyping, offering promising avenues improving outcomes stage-patients.
Language: Английский
Citations
3Current Medical Research and Opinion, Journal Year: 2024, Volume and Issue: 40(9), P. 1483 - 1493
Published: July 31, 2024
Diabetes mellitus, stemming from either insulin resistance or inadequate secretion, represents a complex ailment that results in prolonged hyperglycemia and severe complications. Patients endure ramifications such as kidney disease, vision impairment, cardiovascular disorders, susceptibility to infections, leading significant physical suffering imposing substantial socio-economic burdens. This condition has evolved into an increasingly health crisis. There is urgent need develop new treatments with improved efficacy fewer adverse effects meet clinical demands. However, novel drug development costly, time-consuming, often associated side suboptimal efficacy, making it major challenge. Artificial Intelligence (AI) Machine Learning (ML) have revolutionized across its comprehensive lifecycle, spanning discovery, preclinical studies, trials, post-market surveillance. These technologies significantly accelerated the identification of promising therapeutic candidates, optimized trial designs, enhanced post-approval safety monitoring. Recent advances AI, including data augmentation, interpretable integration AI traditional experimental methods, offer strategies for overcoming challenges inherent AI-based discovery. Despite these advancements, there exists notable gap reviews detailing ML applications throughout entirety developing medications diabetes mellitus. review aims fill this by evaluating impact potential at various stages mellitus development. It does synthesizing current research findings technological so effectively control mitigate far-reaching social economic impacts. The promises revolutionize treatment strategies, offering hope patient outcomes reduced healthcare burdens worldwide.
Language: Английский
Citations
2Published: Jan. 1, 2024
Language: Английский
Citations
0Aquacultural Engineering, Journal Year: 2024, Volume and Issue: unknown, P. 102466 - 102466
Published: Sept. 1, 2024
Language: Английский
Citations
0