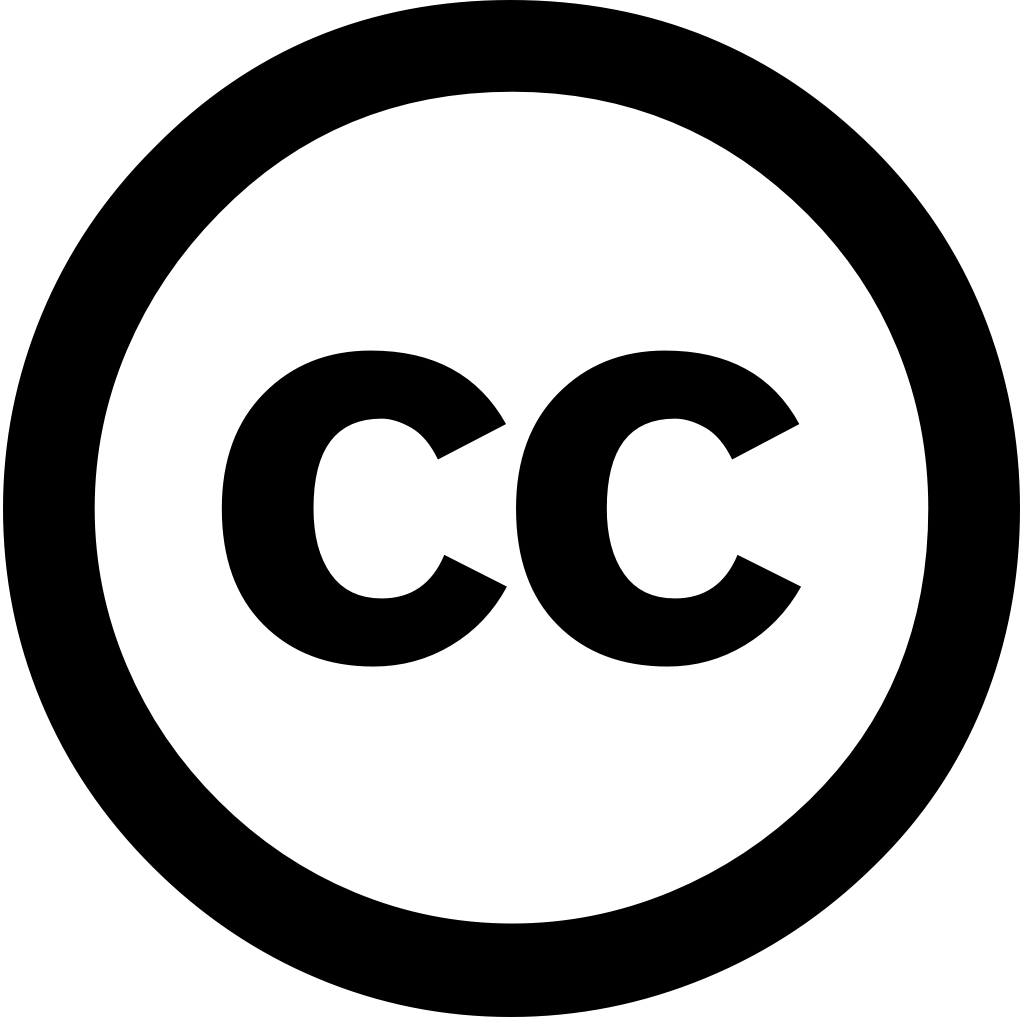
Journal of The Royal Society Interface, Journal Year: 2025, Volume and Issue: 22(226)
Published: May 1, 2025
Bayesian networks (BNs) have been used for reconstructing interactions from biological data, in disciplines ranging molecular biology to ecology and neuroscience. BNs learn conditional dependencies between variables, which best ‘explain’ the represented as a directed graph approximates relationships variables. In 2000s, were popular method that promised an approach capable of inferring data. Here, we review use applied data over past two decades evaluate their efficacy. We find are successful networks, frequently identifying novel or network components missed by previous analyses. suggest false positive results underreported, it is difficult assess accuracy networks. BN learning appears most small numbers variables with high-quality datasets either discretize into few states include perturbative failed live up promise 2000s but this likely due experimental constraints on datasets, success at variety contexts suggests they powerful tool biologists.
Language: Английский