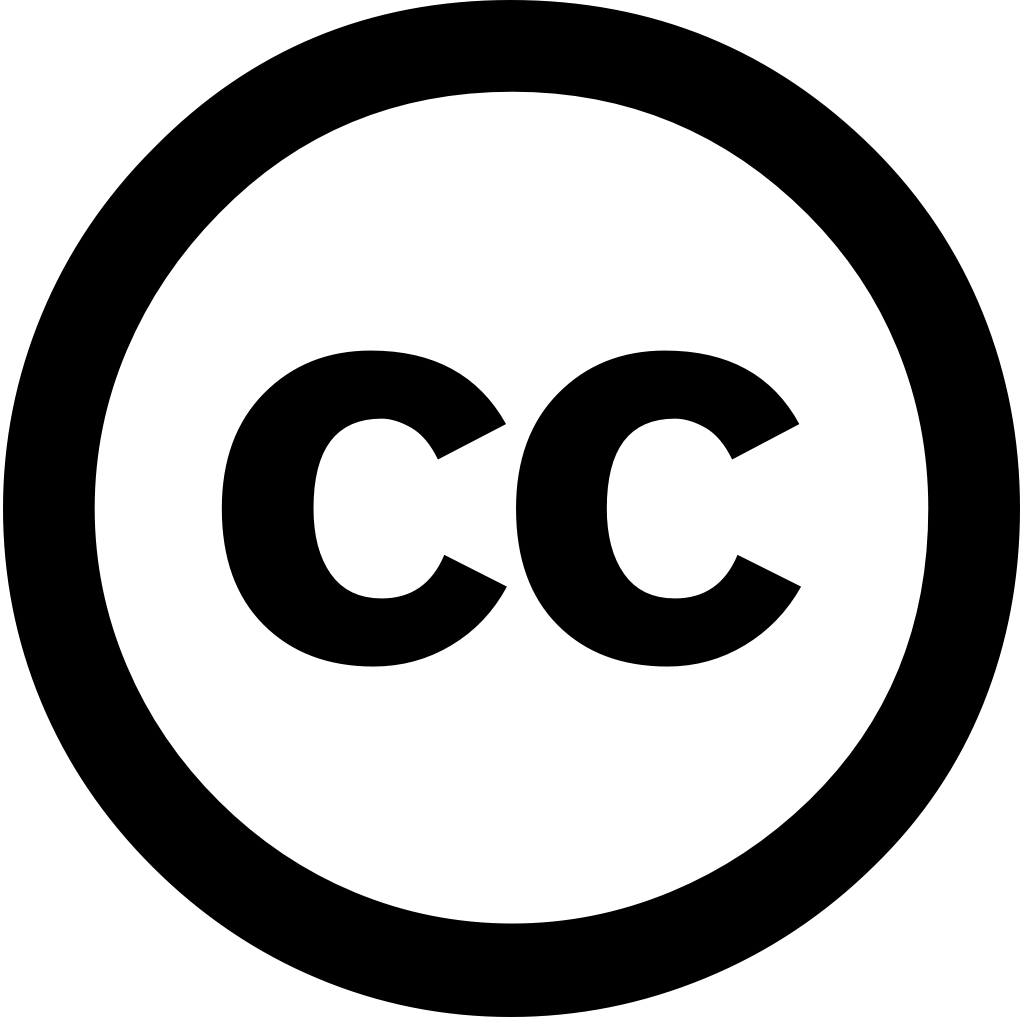
Research Square (Research Square), Journal Year: 2024, Volume and Issue: unknown
Published: April 19, 2024
Abstract Background Idiopathic pulmonary fibrosis (IPF) is one of the interstitial lung diseases (ILDs) with poor prognosis. Multiple regulated cell death (RCD) pathways are involved in regulating progression at different stages. Methods A total 20 RCD and crucial regulatory genes were collected from available literature. The study initially elucidated profiling kinds normal fibrotic tissues based on scRNAseq dataset bulk-RNAseq dataset. Targets associated IPF identified by Mendelian randomization analysis, univariate Cox regression was used to further identify RCD-related significantly overall survival (OS). combination 101 distinct machine-learning algorithms utilized develop a prognostic signature. In addition, we investigated relationship between signature clinical characteristics. Results By integrating data data, pathway activity patterns patients. following detailed research various patterns, developed CDI 13 genes, which combined multiple machine learning methods generate has strong predictive influence prognosis As proven independent datasets, patients high had poorer outcome. From characteristics, have impaired function. Finally, nomogram ability generated Conclusion summary, novel model that effectively forecasts patterns.
Language: Английский