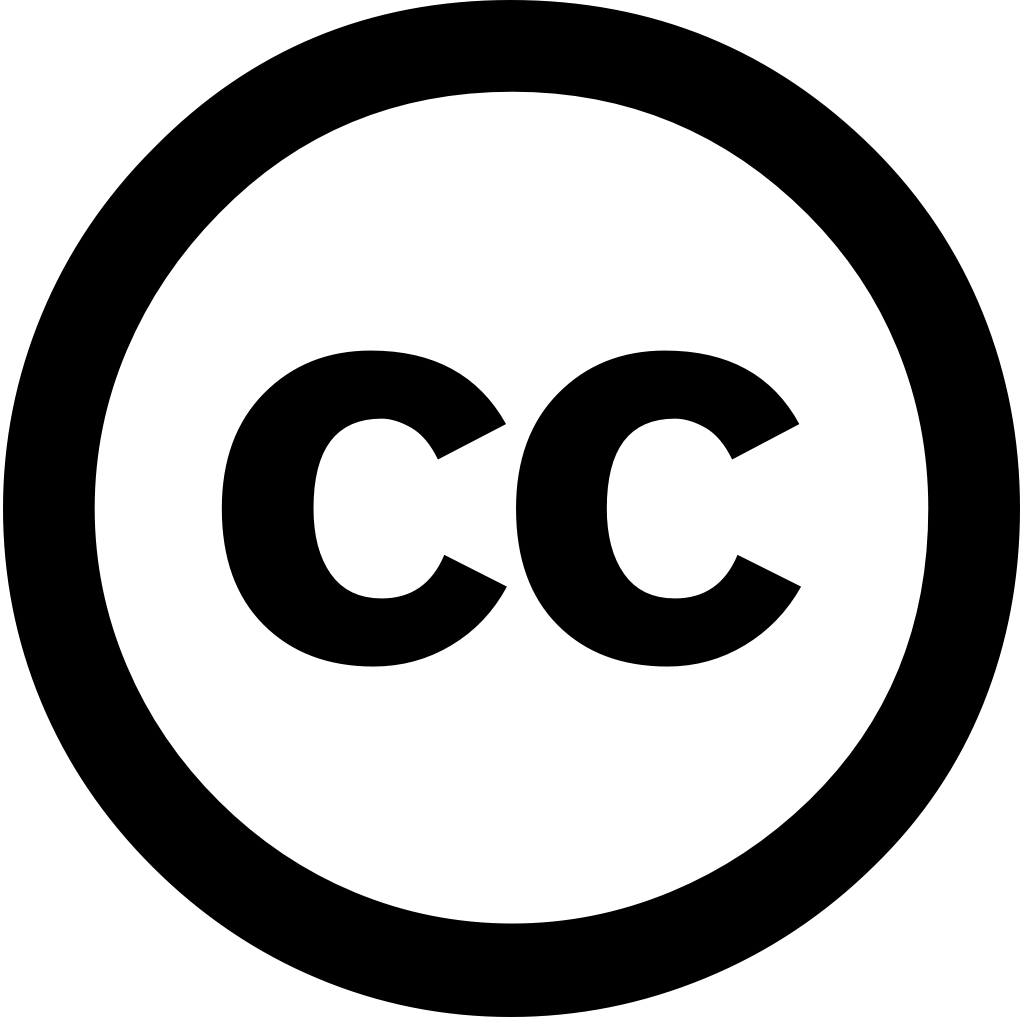
Computers and Electronics in Agriculture, Journal Year: 2024, Volume and Issue: 229, P. 109664 - 109664
Published: Dec. 6, 2024
Language: Английский
Computers and Electronics in Agriculture, Journal Year: 2024, Volume and Issue: 229, P. 109664 - 109664
Published: Dec. 6, 2024
Language: Английский
Journal of Imaging, Journal Year: 2025, Volume and Issue: 11(1), P. 28 - 28
Published: Jan. 20, 2025
Crop field monitoring using unmanned aerial vehicles (UAVs) is one of the most important technologies for plant growth control in modern precision agriculture. One and widely used tasks stand counting. The accurate identification plants images provides estimates number per unit area, detects missing seedlings, predicts crop yield. Current methods are based on detection obtained from UAVs by means computer vision algorithms deep learning neural networks. These approaches depend image spatial resolution quality markup. performance automatic may affect efficiency downstream analysis a cropping pattern. In present work, method presented detecting five species acquired via UAV basis segmentation (convolutional networks). Twelve orthomosaics were collected marked at several sites Russia to train test network algorithms. Additionally, 17 existing datasets various resolutions markup levels Roboflow service extend training sets. Finally, we compared texture features between manually evaluated neural-network-estimated masks. It was demonstrated that adding sample (even those lower quality) improves counting significantly. work indicates how accuracy their pattern evaluation characteristics. For some characteristics (GLCM mean, GLRM long run, run ratio) automatically close. others, differences large lead erroneous conclusions about properties patterns. Nonetheless, overall, with higher show better agreement parameters images.
Language: Английский
Citations
0Agriculture, Journal Year: 2024, Volume and Issue: 14(4), P. 551 - 551
Published: March 30, 2024
The wheat grain yields increased in EU from 4.98 t ha−1 to 5.45 the periods 2006 2014 2015 2023. It is hypothesized that changes specific morphological traits over years resulted yield increase due utilization of new varieties production. To highlight current status and time, we evaluated a comprehensive panel 1322 included testing recognized period till Positive relation registration year with such as seed color, glaucosity neck culm, plant height, ear length, scurs awns shape beak lower glume was obtained. most significant time darker color seed, decreased area hairiness convex surface apical rachis segment, enhanced culm frequency plants recurved flag leaves. shown leaves, emergence, existence awns, segment had decreases time. This research demonstrated importance twelve varietal improvement
Language: Английский
Citations
2Agronomy, Journal Year: 2024, Volume and Issue: 14(9), P. 2036 - 2036
Published: Sept. 6, 2024
Wheat spike count is one of the critical indicators for assessing growth and yield wheat. However, illumination variations, mutual occlusion, background interference have greatly affected wheat detection. A lightweight detection method was proposed based on YOLOv5s. Initially, original YOLOv5s improved by combing additional small-scale layer integrating ECA (Efficient Channel Attention) attention mechanism into all C3 modules (YOLOv5s + 4 ECAC3). After comparing GhostNet, ShuffleNetV2, MobileNetV3, GhostNet architecture finally selected as optimal model framework its superior performance in various evaluations. Subsequently, incorporation five different activation functions network led to identification RReLU (Randomized Leaky ReLU) function most effective augmenting network’s performance. Ultimately, loss CIoU (Complete Intersection over Union) optimized using EIoU function. Despite a minor reduction 2.17% accuracy refined ECAC3 G RR E when compared ECAC3, there marginal improvement 0.77% Furthermore, parameter diminished 32% 28.2% relative YOLOv5s, respectively. The size reduced 28.0% 20%, Giga Floating-point Operations Per Second (GFLOPs) were lowered 33.2% 9.5%, respectively, signifying substantial efficiency without significantly compromising accuracy. This study offers methodological reference rapid accurate agricultural objects through enhancement deep learning network.
Language: Английский
Citations
1Plants, Journal Year: 2024, Volume and Issue: 13(19), P. 2736 - 2736
Published: Sept. 30, 2024
The spike shape and morphometric characteristics are among the key of cultivated cereals, being associated with their productivity. These traits often used for plant taxonomy authenticity hexaploid wheat species. Manual measurement is tedious not precise. Recently, authors this study developed a method morphometry utilizing 2D image analysis. Here, applied to variations in size 190 plants seven (2n = 6x 42) species one artificial amphidiploid wheat. Five manually estimated 26 obtained from digital analysis were analyzed. Image-based describe base, center apex common parameters (circularity, roundness, perimeter, etc.). Estimates similar by manual shown be highly correlated, suggesting practical importance phenotyping. utility classification into types (spelt, normal compact) or shown. It also demonstrated that estimates made it possible identify differing significantly between accessions within same present work suggests usefulness using an approach based on
Language: Английский
Citations
0Computers and Electronics in Agriculture, Journal Year: 2024, Volume and Issue: 229, P. 109664 - 109664
Published: Dec. 6, 2024
Language: Английский
Citations
0